Spectral Change Point Estimation for High Dimensional Time Series by Sparse Tensor Decomposition
arXiv (Cornell University)(2023)
摘要
We study the problem of change point (CP) detection with high dimensional time series, within the framework of frequency domain. The overarching goal is to locate all change points and for each change point, delineate which series are activated by the change, over which set of frequencies. The working assumption is that only a few series are activated per change and frequency. We solve the problem by computing a CUSUM tensor based on spectra estimated from blocks of the observed time series. A frequency-specific projection approach is applied to the CUSUM tensor for dimension reduction. The projection direction is estimated by a proposed sparse tensor decomposition algorithm. Finally, the projected CUSUM vectors across frequencies are aggregated by a sparsified wild binary segmentation for change point detection. We provide theoretical guarantees on the number of estimated change points and the convergence rate of their locations. We derive error bounds for the estimated projection direction for identifying the frequency-specific series that are activated in a change. We provide data-driven rules for the choice of parameters. We illustrate the efficacy of the proposed method by simulation and a stock returns application.
更多查看译文
关键词
spectral change point estimation,high dimensional time series,tensor
AI 理解论文
溯源树
样例
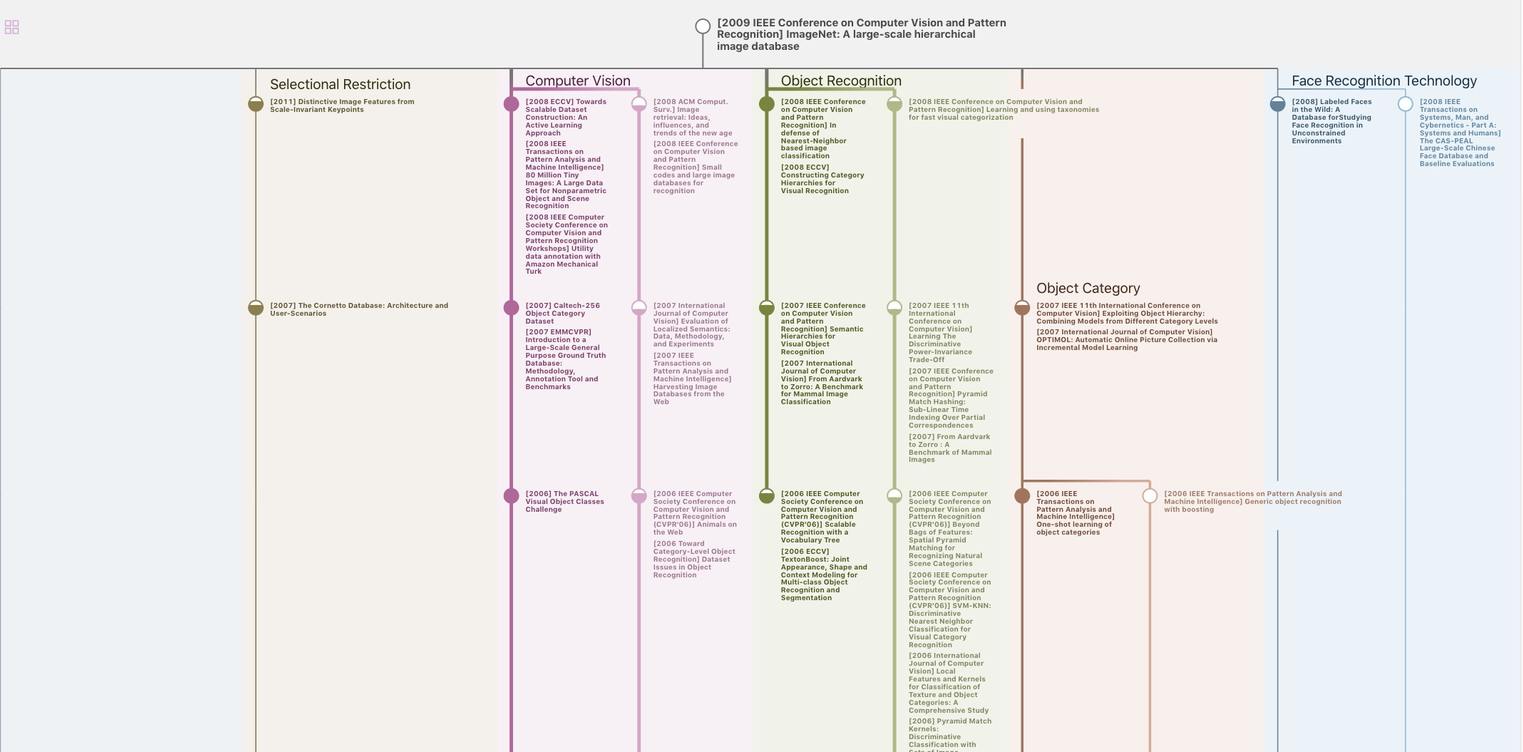
生成溯源树,研究论文发展脉络
Chat Paper
正在生成论文摘要