Machine learning outcome prediction using stress perfusion cardiac magnetic resonance and electronic health records
European Journal of Echocardiography(2023)
摘要
Abstract Funding Acknowledgements Type of funding sources: Public grant(s) – National budget only. Main funding source(s): Wellcome Trust. Background Stress perfusion cardiac magnetic resonance (SP-CMR) is a well validated and guidelines backed diagnostic test for non-invasive assessment of patients with known or suspected coronary artery disease (CAD)(1). The prognostic value of SP-CMR is enhanced by adding clinical information, however, most electronic health record (EHR) is stored in an unstructured format, therefore limiting access to the information stored when large datasets are concerned. Furthermore, current outcome prediction models rely on linear models, but recent literature has shown stronger prediction using non-linear models (2). Purpose We aimed to address these challenges by using artificial intelligence (AI) tools to extract unstructured data from SP-CMR database and improve outcome prediction. Methods SP-CMR cases from 2011 to 2021 were screened. Data extraction was performed using Cogstack (3), an information retrieval and extraction platform for unstructured data based on natural language processing (NLP). Data were analysed for survival using Log Normal curves. Multivariate analysis was performed using survival Cox model. Different machine learning models were trained to predict survival, and compared using area under the receiver operating characteristic curve (AUC) and the DeLong test. 80% of dataset was used for training and 20% for testing. P value of <0.05 was considered statistically significant. Results 4,188 cases were included in the analysis. Total number of events (deaths) was 252 (6%). Ischaemia on stress perfusion imaging or the presence of myocardial scar on late gadolinium enhancement were significantly associated with events (Logrank p<0.001). Independent predictors from Cox survival analysis used to train machine learning models were: age, chronic kidney disease, hypertension, male gender, smoking, heart failure, positive ischaemic myocardial scar, positive stress perfusion, and ventricular ejection fraction. Considering clinical parameters only, support vector machine survival prediction performed best (AUC 0.76, F1 score 0.24), followed by XGBoost, random forest and ensemble classifier. After adding CMR predictors (stress perfusion, ischaemic myocardial scar, and left ventricular ejection fraction), prediction power improved for all machine learning models, with support vector machine having the best performance (AUC 0.82, F1 score 0.30). After comparing AUCs, non-linear models were better than multilinear regression (p<0.001, Z score −19). Conclusion NLP-based data extraction enables immediate access to unstructured EHR and reveals plausible results, which can be used within an integrated pipeline for data analysis and prediction, and has advantages over structured data due to access to large datasets. Machine learning-based prediction is superior to conventional linear models due to the added ability to model non-linear relationships. CMR predictors improve the survival prediction, compared to clinical variables alone.
更多查看译文
关键词
stress perfusion,cardiac magnetic resonance,machine learning,prediction
AI 理解论文
溯源树
样例
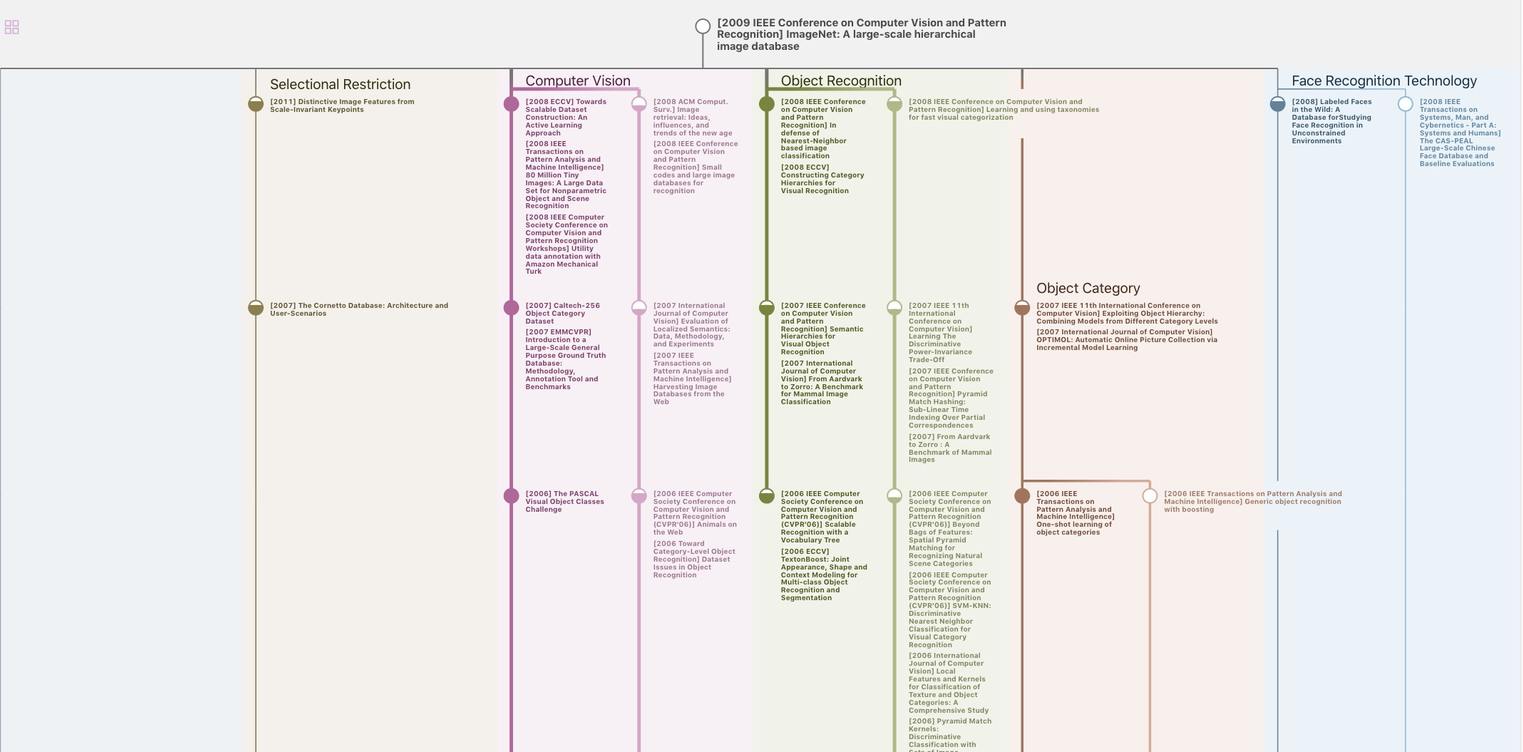
生成溯源树,研究论文发展脉络
Chat Paper
正在生成论文摘要