Abstract 3166: CanSig: De novo discovery of shared transcriptional states in cancer single cells
Cancer Research(2023)
Abstract
Abstract Tumor heterogeneity is regarded as a significant obstacle to successful personalized cancer medicine. Specifically, multiple cancer types have been shown to exhibit heterogeneity in the transcriptional states of malignant cells even within the same tumor. Some of these specific transcriptional states of malignant cells have been linked to cancer relapse and resistance to treatment. However, today there is no universal and easy-to-use computational method to extract information about shared transcriptional states from single-cell RNA sequencing measurements (scRNA-seq). To reliably identify shared transcriptional states of cancer cells, we propose a novel computational tool, CanSig. CanSig automatically preprocesses, integrates, and analyzes cancer scRNA-seq data from multiple patients to provide novel signatures of shared transcriptional states; it also associates these states to known and potentially targetable biological pathways. CanSig jointly analyzes cells from multiple cancer patients while correcting for batch effects and differences in gene expressions caused by genetic heterogeneity. For this, CanSig automatically infers copy number variations in malignant cells and trains a deep learning architecture based on conditional variational autoencoders integrating scRNA-seq measurements. In our benchmarks, CanSig shows state-of-the-art performance in data integration and reliably re-discovers known transcriptional signatures on four previously published cancer scRNA-seq datasets. For instance, CanSig re-discovers four main cellular states of glioblastoma cells previously reported by Neftel et al., Cell, 2019. We further illustrate CanSig’s investigative potential by uncovering signatures of novel transcriptional states in several cancer types; some of the novel signatures are linked to such cancer hallmarks as cell migration and proliferation and are enriched in more advanced tumors. We also uncover signatures associated with specific genomic aberrations such as gene copy number gains. In conclusion, CanSig detects shared transcriptional states in tumors using as input scRNA-seq data for cells with different genetic backgrounds and possibly exhibiting strong batch effects. It can significantly facilitate the analysis of scRNA-seq cancer data and efficiently identify transcriptional signatures linked to known biological pathways. The CanSig method implemented in Python is available at https://github.com/BoevaLab/CanSig Citation Format: Josephine Yates, Florian Barkmann, Pawel Piotr Czyz, Agnieszka Kraft, Niko Beerenwinkel, Valentina Boeva. CanSig: De novo discovery of shared transcriptional states in cancer single cells [abstract]. In: Proceedings of the American Association for Cancer Research Annual Meeting 2023; Part 1 (Regular and Invited Abstracts); 2023 Apr 14-19; Orlando, FL. Philadelphia (PA): AACR; Cancer Res 2023;83(7_Suppl):Abstract nr 3166.
MoreTranslated text
Key words
shared transcriptional states,single cells,cancer
AI Read Science
Must-Reading Tree
Example
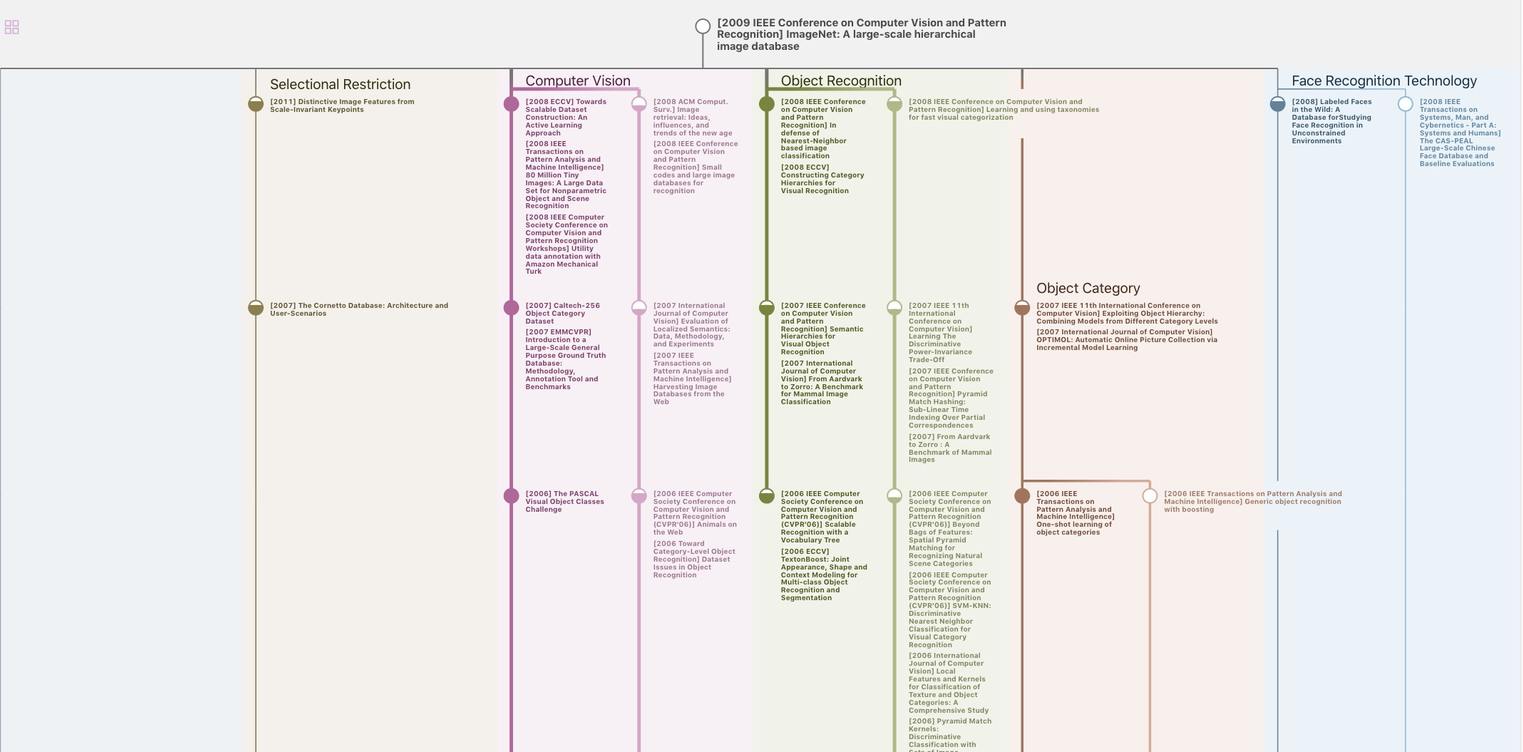
Generate MRT to find the research sequence of this paper
Chat Paper
Summary is being generated by the instructions you defined