Enhancing Representation Learning With Spatial Transformation and Early Convolution for Reinforcement Learning-Based Small Object Detection
IEEE TRANSACTIONS ON CIRCUITS AND SYSTEMS FOR VIDEO TECHNOLOGY(2024)
Abstract
Although object detection has achieved significant progress in the past decade, detecting small objects is still far from satisfactory due to the high variability of object scales and complex backgrounds. The common way to enhance small object detection is to use high-resolution (HR) images. However, this method incurs huge computational resources which grow squarely with the resolution of images. To achieve both accuracy and efficiency, we propose a novel reinforcement learning framework that employs an efficient policy network consisting of a Spatial Transformation Network to enhance the state representation learning and a Transformer model with early convolution to improve feature extraction. Our method has two main steps: (1) coarse location query (CLQ), where an RL agent is trained to predict the locations of small objects on low-resolution (LR) (down-sampled version of HR) images; (2) context-sensitive object detection where HR image patches are used to detect objects on the selected coarse locations and LR image patches on background areas (containing no small objects). In this way, we can obtain high detection performance on small objects while avoiding unnecessary computation on background areas. The proposed method has been tested and benchmarked on various datasets. On the Caltech Pedestrians Detection and Web Pedestrians datasets, the proposed method improves the detection accuracy by 2%, while reducing the number of processed pixels. On the Vision meets Drone object detection dataset and the Oil and Gas Storage Tank dataset, the proposed method outperforms the state-of-the-art (SotA) methods. On MS COCO mini-val set, our method outperforms SotA methods on small object detection, while also achieving comparable performance on medium and large objects.
MoreTranslated text
Key words
Detectors,Training,Object detection,Feature extraction,Costs,Transformers,Prediction algorithms,Small object detection,reinforcement learning,coarse-to-fine framework
AI Read Science
Must-Reading Tree
Example
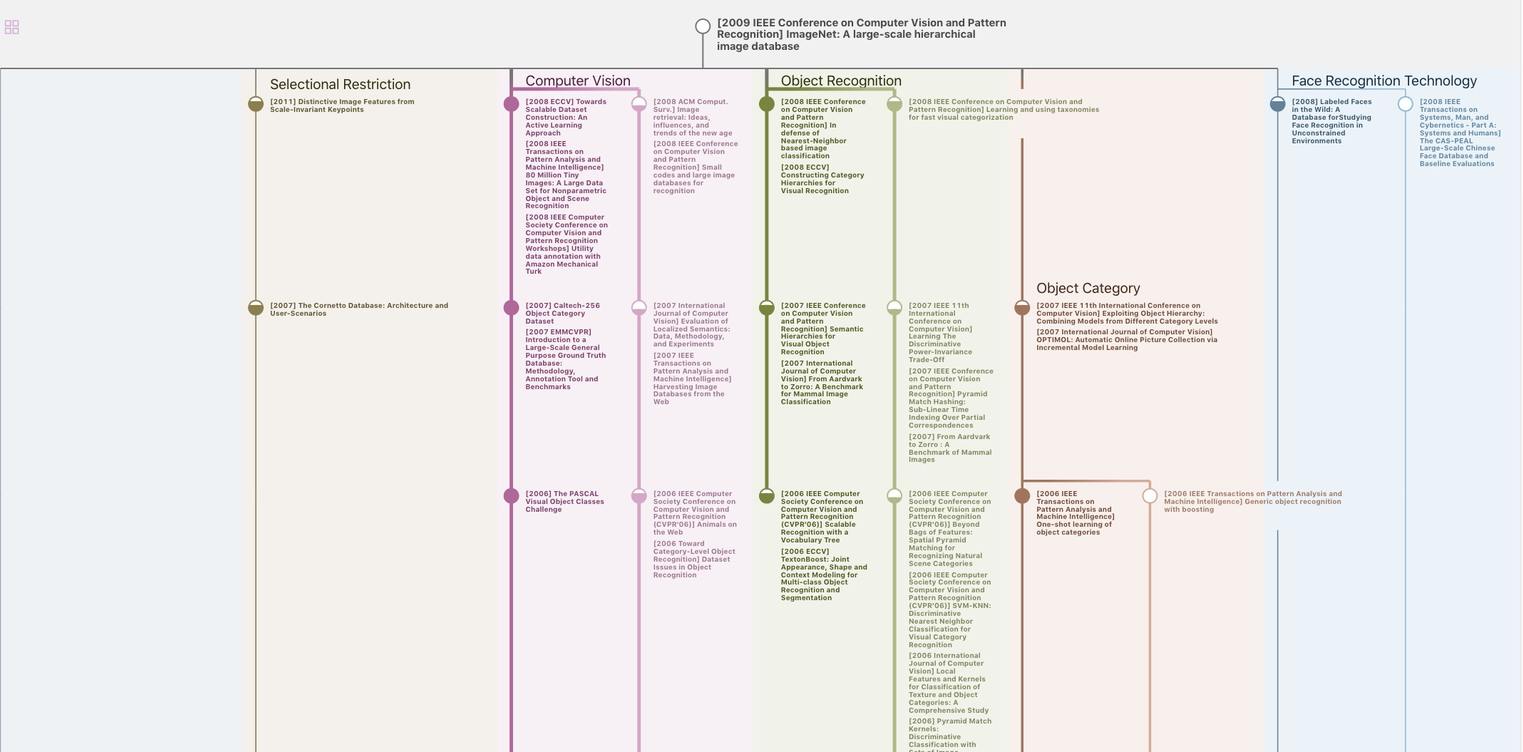
Generate MRT to find the research sequence of this paper
Chat Paper
Summary is being generated by the instructions you defined