Physics-Informed Neural Networks for Solving Two-Dimensional Magnetostatic Fields
IEEE Transactions on Magnetics(2023)
摘要
Physics-informed neural network (PINN) has shown great potential in the inverse and parametric designing problems in electrical engineering. Moreover, most existing works on PINN are dedicated to computational fluids, and very few attentions have been paid to static and low-frequency electromagnetic near fields with multiple media in electrical engineering applications. In this work, a PINN for solving two-dimensional (2D) magnetostatic fields in electromagnetic devices and systems is proposed. The magnetic field intensity and the magnetic vector potential are solved by training a neural network which encodes partial differential equations and boundary conditions as residuals. The computation of the spatial derivatives of media constitutive parameters, which negatively impacts the training of PINN, is eliminated. A mesh-assisted non-uniform sampling method for the selection of collocation points is proposed to further improve the performance of PINN. The proposed PINN is verified by comparing its results with those of the finite element method in two 2D magnetostatic case studies. It is expected that this work will promote further applications of PINN in the modelling, numerical analysis, and parametric design of electromagnetic devices and systems.
更多查看译文
关键词
neural networks,physics-informed,two-dimensional
AI 理解论文
溯源树
样例
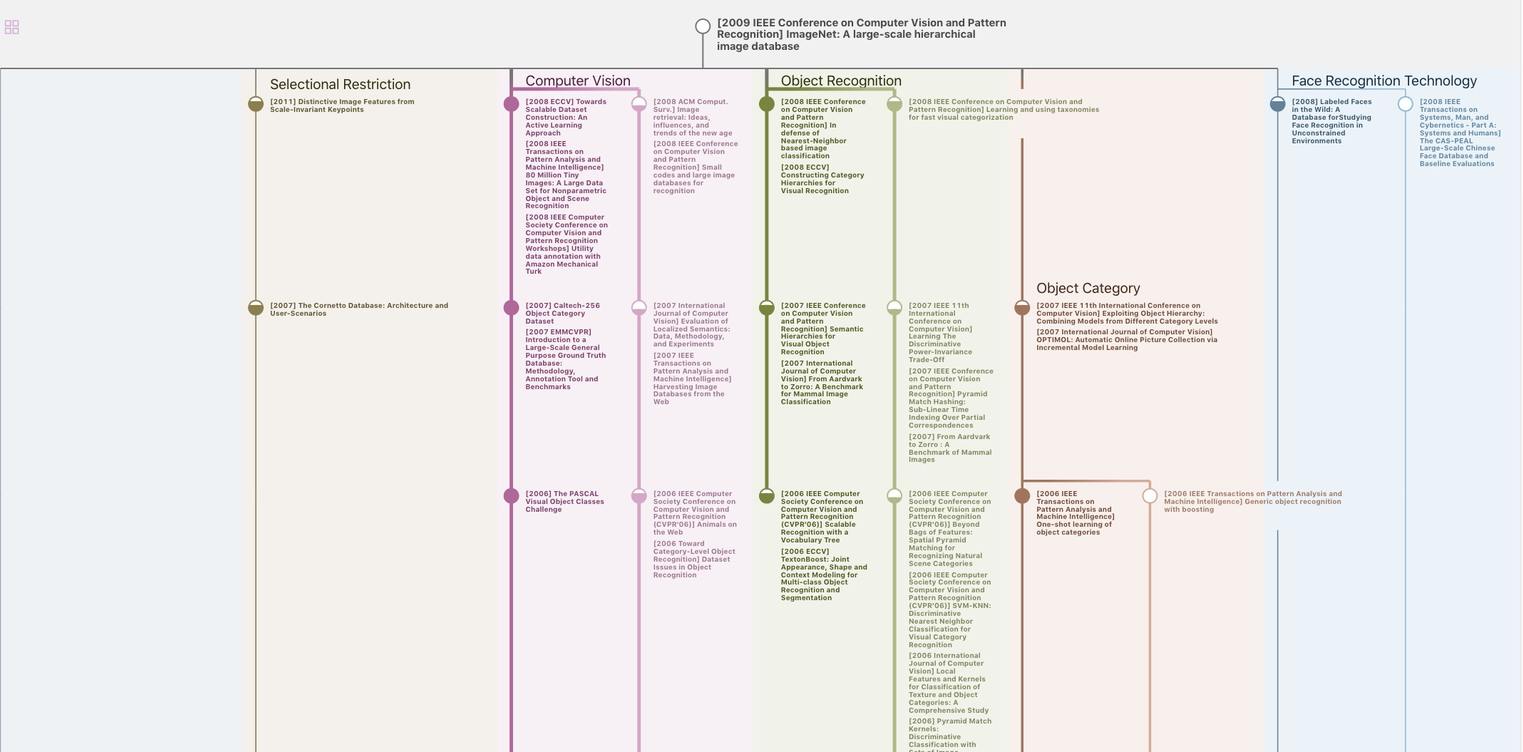
生成溯源树,研究论文发展脉络
Chat Paper
正在生成论文摘要