Adversarial Attack-Resilient Perception Module for Traffic Sign Classification
Research Square (Research Square)(2023)
摘要
Abstract Deep Learning (DL)-based image classification models are essential for autonomous vehicle (AV) perception modules since incorrect categorization might have severe repercussions. Adversarial attacks are widely studied cyberattacks that can lead DL models to predict inaccurate output, such as incorrectly classified traffic signs by the perception module of an autonomous vehicle. In this study, we create and compare Hybrid Classical-Quantum Deep Learning (HCQ-DL) models with Classical Deep Learning (C-DL) models to demonstrate robustness against adversarial attacks for perception modules. Before feeding them into the quantum system, we used transfer learning models like AlexNet and VGG-16 as feature extractors. We tested over 1000 quantum circuits in our HCQ-DL models for Projected Gradient Descent (PGD), Fast Gradient Sign Attack (FGSA), and Gradient Attack (GA), which are three well-known untargeted adversarial approaches. We evaluated the performance of all models during adversarial attack and no-attack scenarios. Our HCQ-DL models maintain accuracy above 95% during a no-attack scenario and above 91% for GA and FGSA attacks, which is higher than C-DL models. During the PGD attack, our AlexNet-based HCQ-DL model maintained an accuracy of 85% compared to C-DL models that achieved accuracies below 21%.
更多查看译文
关键词
classification,sign,perception,attack-resilient
AI 理解论文
溯源树
样例
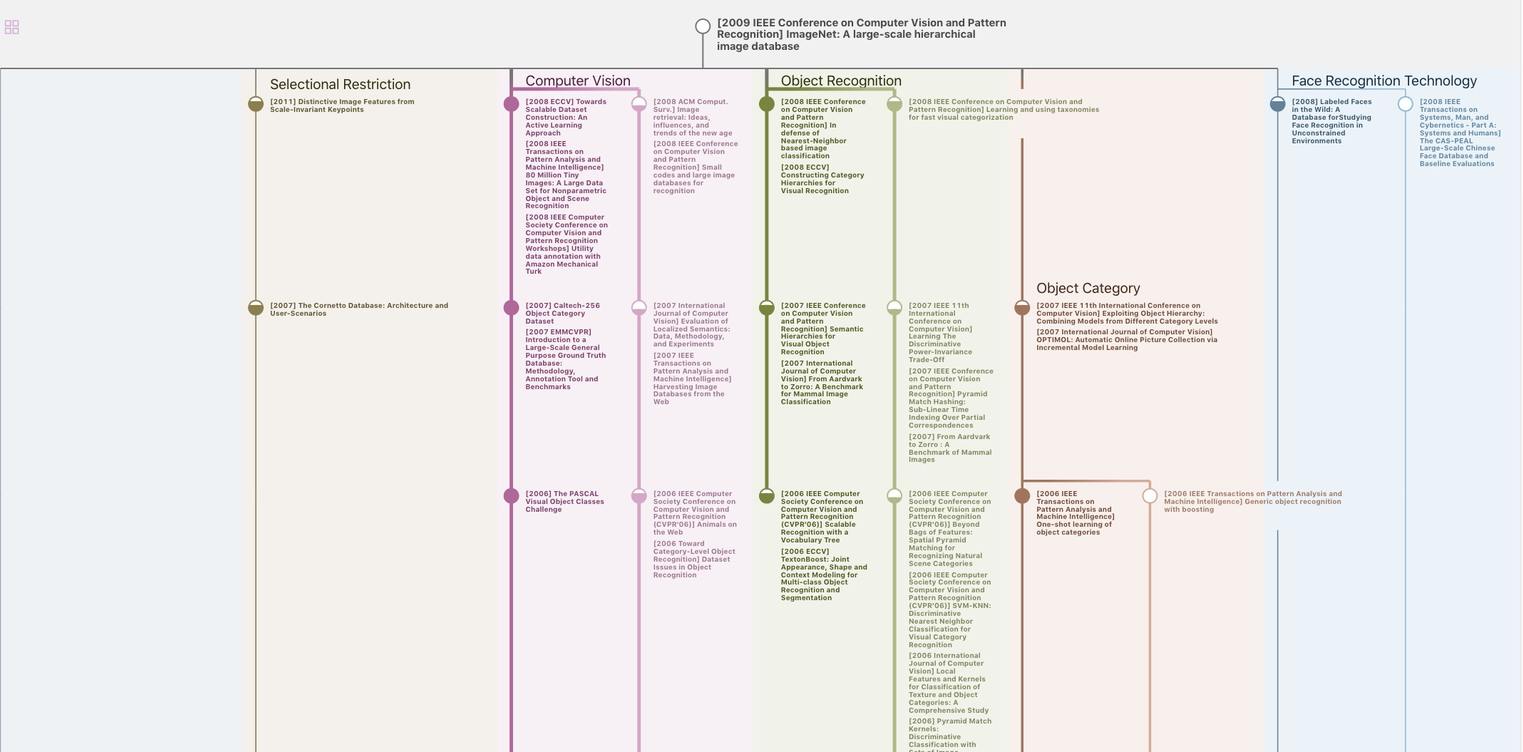
生成溯源树,研究论文发展脉络
Chat Paper
正在生成论文摘要