Learn to Unlearn: A Survey on Machine Unlearning
arXiv (Cornell University)(2023)
摘要
Machine Learning (ML) models have been shown to potentially leak sensitive information, thus raising privacy concerns in ML-driven applications. This inspired recent research on removing the influence of specific data samples from a trained ML model. Such efficient removal would enable ML to comply with the "right to be forgotten" in many legislation, and could also address performance bottlenecks from low-quality or poisonous samples. In that context, machine unlearning methods have been proposed to erase the contributions of designated data samples on models, as an alternative to the often impracticable approach of retraining models from scratch. This article presents a comprehensive review of recent machine unlearning techniques, verification mechanisms, and potential attacks. We further highlight emerging challenges and prospective research directions (e.g. resilience and fairness concerns). We aim for this paper to provide valuable resources for integrating privacy, equity, andresilience into ML systems and help them "learn to unlearn".
更多查看译文
关键词
learn,machine,survey
AI 理解论文
溯源树
样例
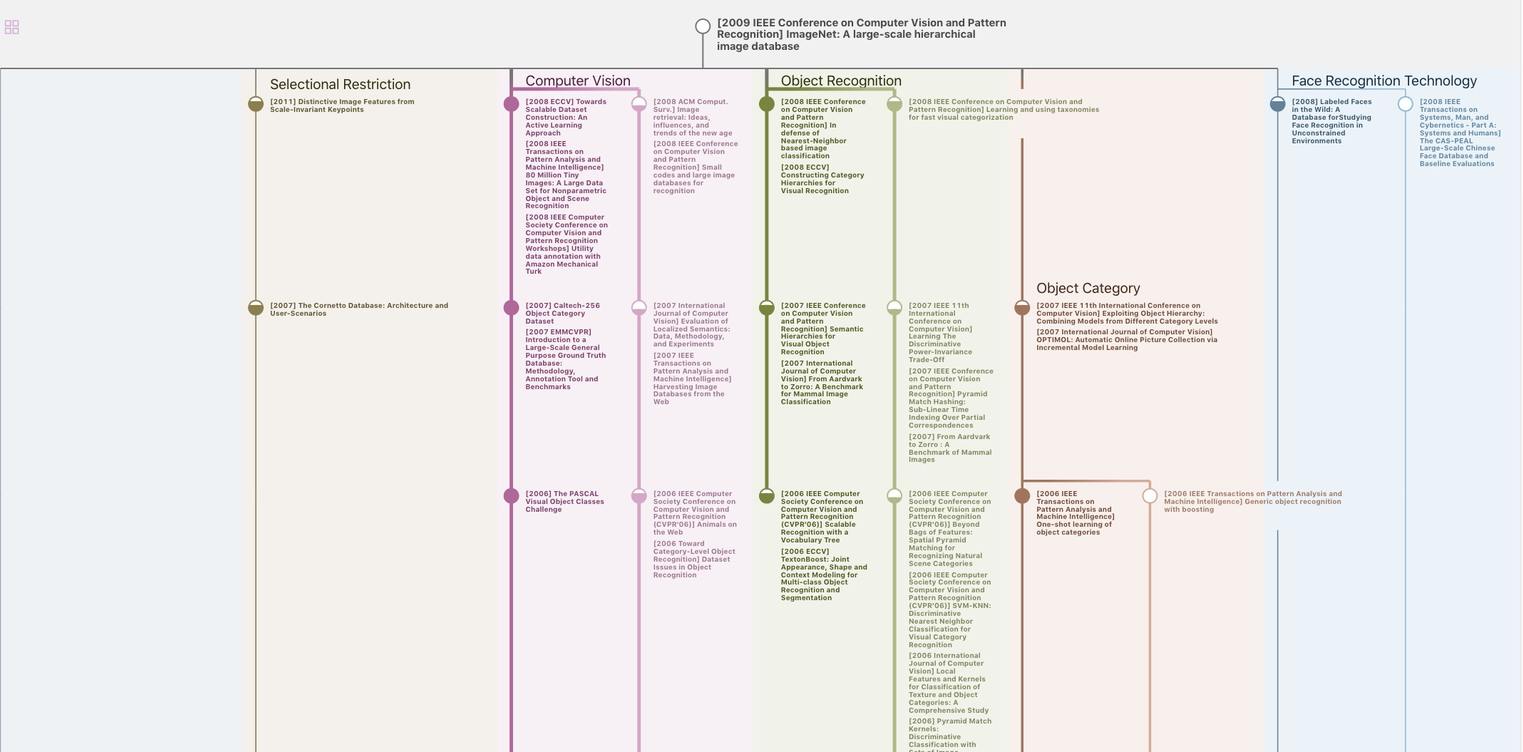
生成溯源树,研究论文发展脉络
Chat Paper
正在生成论文摘要