FLLF: A Fast-Lightweight Location Detection Framework for False Data Injection Attacks in Smart Grids
IEEE TRANSACTIONS ON SMART GRID(2024)
摘要
This paper proposes a fast-lightweight location detection framework (FLLF) for false data injection attacks (FDIAs). The location detection of false data injection attacks is traditionally realized by computationally intensive neural networks, which can cause large detection delays due to the limited computation power and storage resources of the detection device. By contrast, the proposed method is lightweight and can be deployed on low-cost devices such as embedded devices, significantly improving detection speed while reducing power consumption. The proposed method consists of an efficient FDIA location detection model and an automatic model search algorithm. The model is a lightweight model that enables fast and accurate attack location detection through a combination of the early exiting mechanism and mixed-precision quantization (EE-MPQ). Then, an automatic model search algorithm is designed to enable the EE-MPQ model to match the smart grids with different structures. Finally, it is shown that the proposed method can accurately detect and locate FDIAs through numerical analysis on the IEEE 14-bus and 118-bus power systems.
更多查看译文
关键词
False data injection attack,deep neural network,early exiting mechanism,mixed-precision quantization,smart grids
AI 理解论文
溯源树
样例
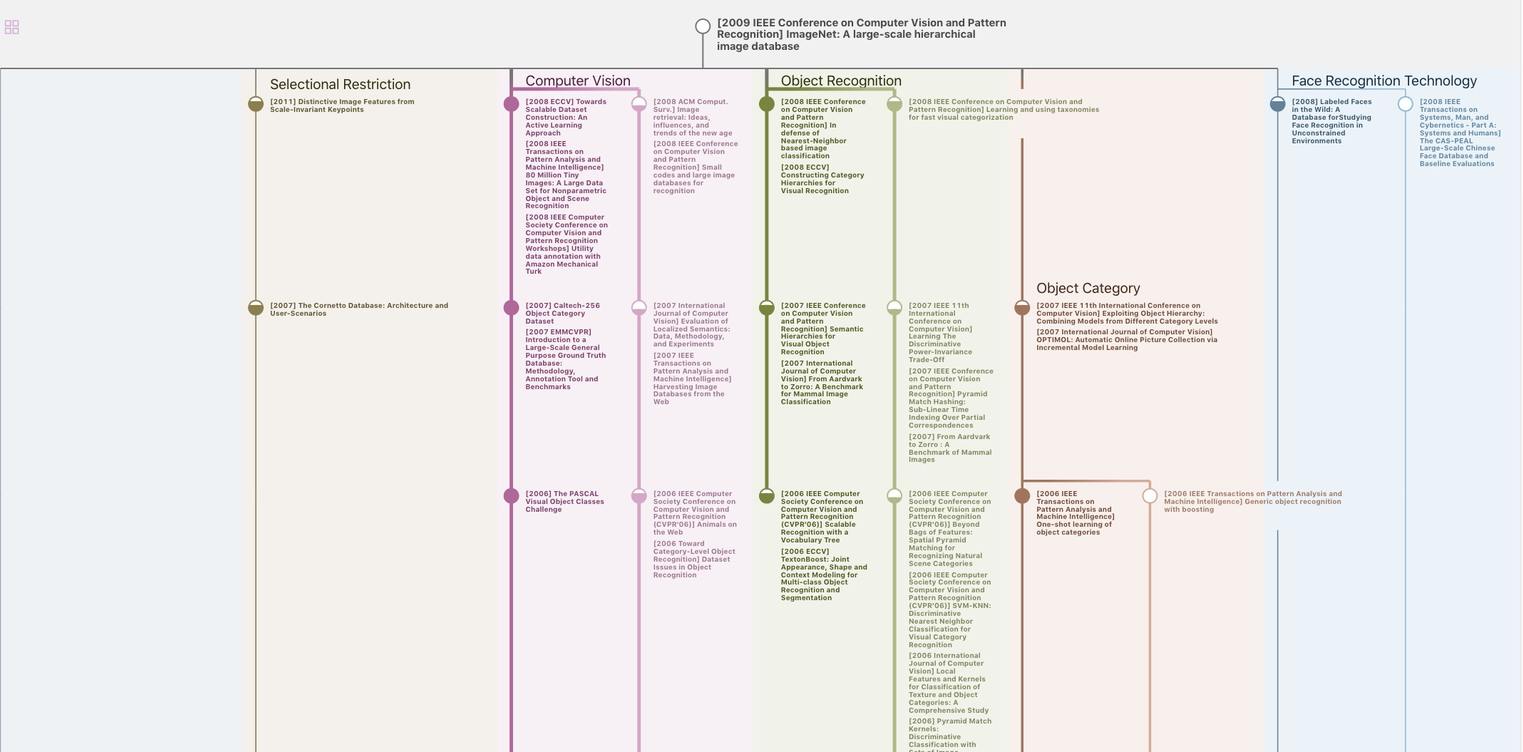
生成溯源树,研究论文发展脉络
Chat Paper
正在生成论文摘要