A vector quantized masked autoencoder for audiovisual speech emotion recognition
arXiv (Cornell University)(2023)
摘要
While fully-supervised models have been shown to be effective for audiovisual speech emotion recognition (SER), the limited availability of labeled data remains a major challenge in the field. To address this issue, self-supervised learning approaches, such as masked autoencoders (MAEs), have gained popularity as potential solutions. In this paper, we propose the VQ-MAE-AV model, a vector quantized MAE specifically designed for audiovisual speech self-supervised representation learning. Unlike existing multimodal MAEs that rely on the processing of the raw audiovisual speech data, the proposed method employs a self-supervised paradigm based on discrete audio and visual speech representations learned by two pre-trained vector quantized variational autoencoders. Experimental results show that the proposed approach, which is pre-trained on the VoxCeleb2 database and fine-tuned on standard emotional audiovisual speech datasets, outperforms the state-of-the-art audiovisual SER methods.
更多查看译文
关键词
audiovisual speech emotion
AI 理解论文
溯源树
样例
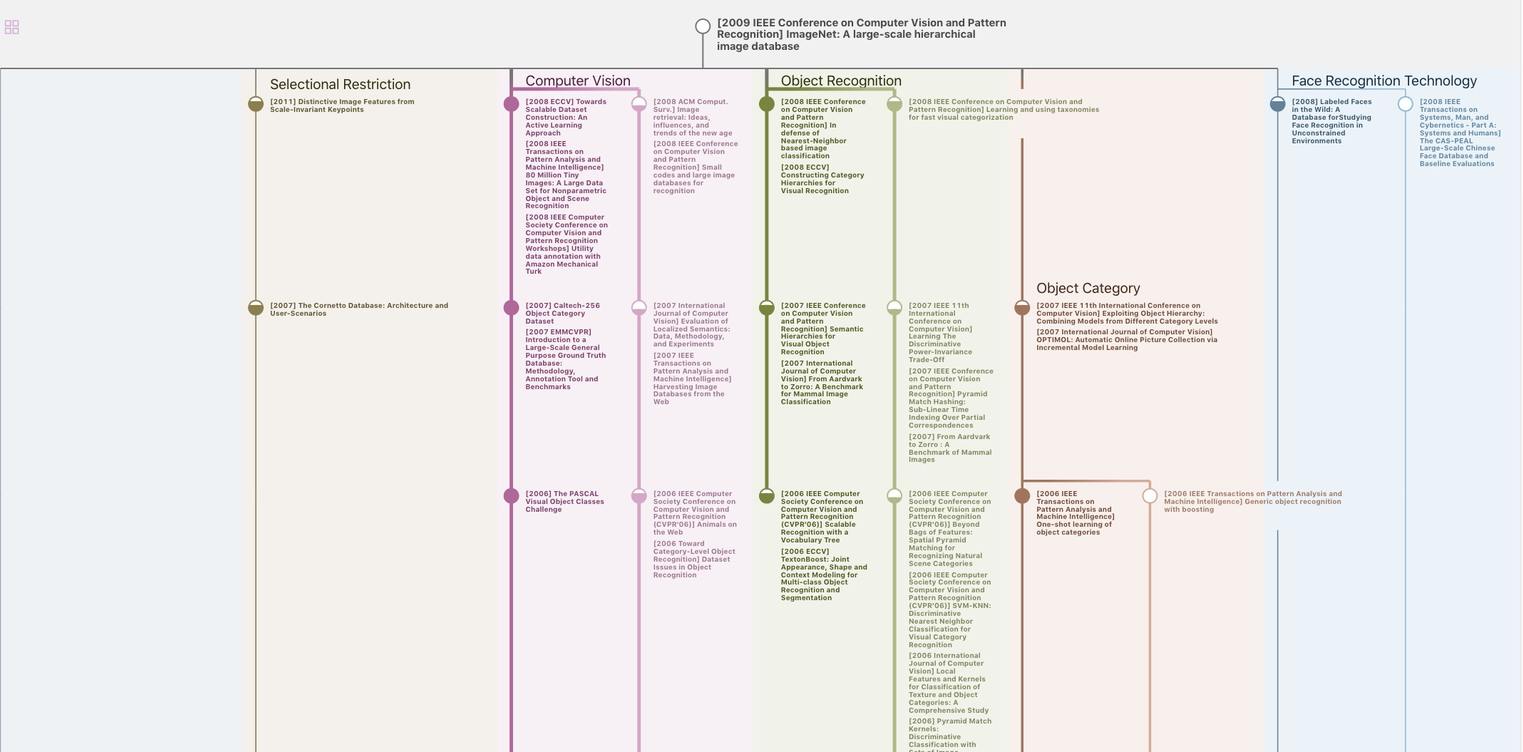
生成溯源树,研究论文发展脉络
Chat Paper
正在生成论文摘要