Machine Learning-Assisted Fabrication of PCBM-Perovskite Solar Cells with Nanopatterned TiO2 Layer
ENERGY & ENVIRONMENTAL MATERIALS(2023)
摘要
To unlock the full potential of PSCs, machine learning (ML) was implemented in this research to predict the optimal combination of mesoporous-titanium dioxide (mp-TiO2) and weight percentage (wt%) of phenyl-C-61-butyric acid methyl ester (PCBM), along with the current density (J(sc)), open-circuit voltage (V-oc), fill factor (ff), and energy conversion efficiency (ECE). Then, the combination that yielded the highest predicted ECE was selected as a reference to fabricate PCBM-PSCs with nanopatterned TiO2 layer. Subsequently, the PCBM-PSCs with nanopatterned TiO2 layers were fabricated and characterized to further understand the effects of nanopatterning depth and wt% of PCBM on PSCs. Experimentally, the highest ECE of 17.338% is achieved at 127 nm nanopatterning depth and 0.10 wt% of PCBM, where the J(sc), V-oc, and ff are 22.877 mA cm(-2), 0.963 V, and 0.787, respectively. The measured J(sc), V-oc, ff, and ECE values show consistencies with the ML prediction. Hence, these findings not only revealed the potential of ML to be used as a preliminary investigation to navigate the research of PSCs but also highlighted that nanopatterning depth has a significant impact on J(sc), and the incorporation of PCBM on perovskite layer influenced the V-oc and ff, which further boosted the performance of PSCs.
更多查看译文
关键词
machine learning,nanopatterning,PCBM,perovskite solar cells,prediction
AI 理解论文
溯源树
样例
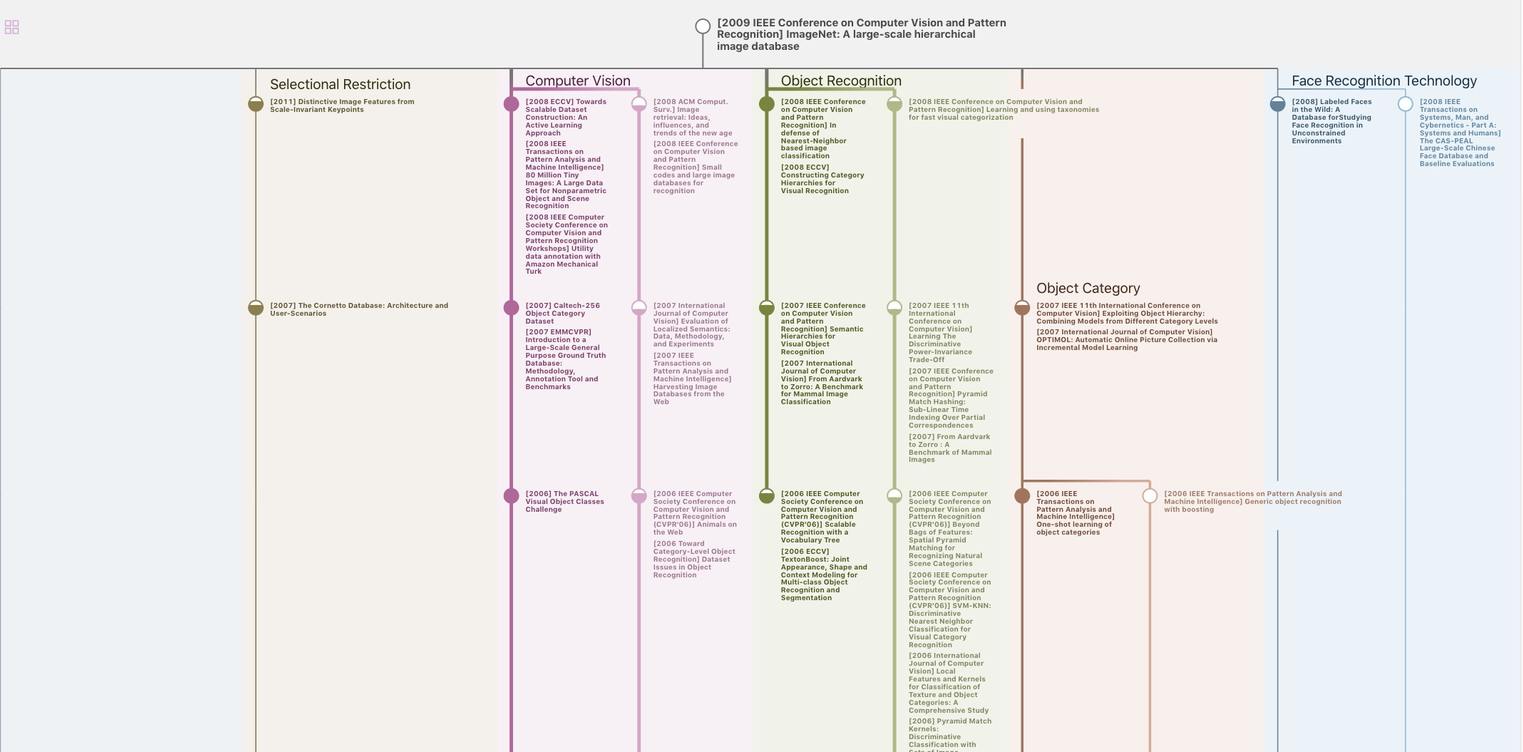
生成溯源树,研究论文发展脉络
Chat Paper
正在生成论文摘要