Prototypical Latent Space Regularization for Improved Reconstruction Quality in Variational Autoencoders
Research Square (Research Square)(2023)
摘要
Abstract Variational autoencoders use latent space regularization with known distributions to facilitate stochastic synthesis via simple sampling procedures. However, existing regularization approaches often struggle to balance regularization and reconstruction quality effectively. To address this, we propose a distributed latent space regularization approach using prototypical deep clustering with known distributions. Our method achieves high-quality data synthesis by decoding samples around prototypes, without the need for auxiliary networks to explicitly learn decoder synthesis from codes. Our approach enables obtaining an appropriate number of clusters for solid regularization with better reconstruction quality and improved synthesis control. Through widespread exploratory benchmarks, we demonstrate that our regularization centered on optimal prototypes' coordinates or cluster centroids neutralizes the adverse effects of regularization terms on autoencoder reconstruction quality, matching non-regularized autoencoders' performance. Additionally, we report promising results for interpreting data representatives via simple prototype synthesis and controlling the synthesis of samples with prototype-like characteristics by decoding noise around prototype coordinates using known distributions.
更多查看译文
关键词
variational autoencoders,improved reconstruction quality
AI 理解论文
溯源树
样例
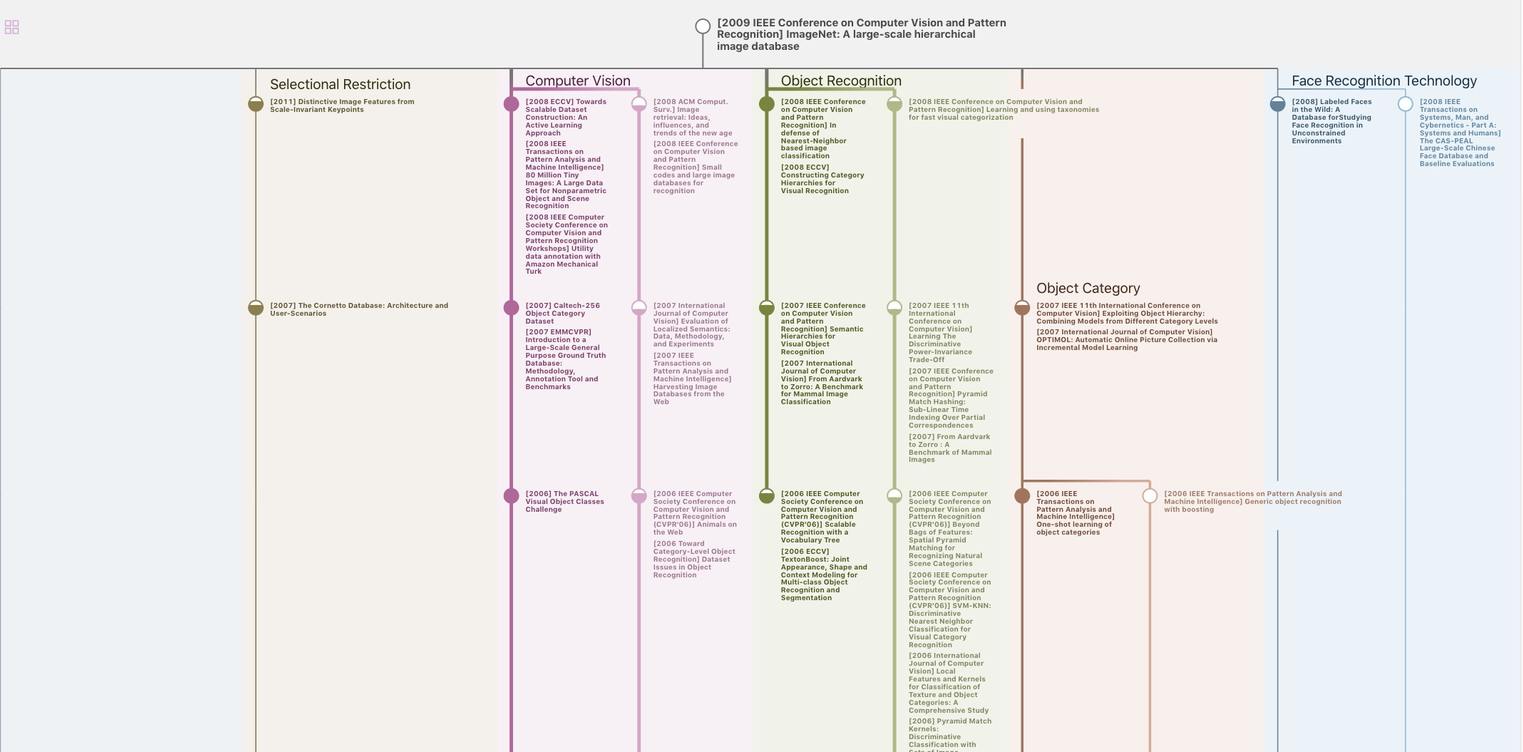
生成溯源树,研究论文发展脉络
Chat Paper
正在生成论文摘要