Cavitation noise characterization and classification using machine-learning techniques
Journal of the Acoustical Society of America(2023)
Abstract
Noise characterization of cavitation has use in biomedicine, sonochemistry, and waste degradation. Typically, spectral features of the cavitation noise signals obtained through Fourier analysis are specific to the experimental set-up and are analyzed to classify the signals in terms of presumed domains of bubble behaviors. The objective of this research was to develop the experimental capabilities and algorithm to monitor and classify cavitation a priori using machine learning (ML) in a precise, repeatable, and translatable fashion among various setups and applications. Initially, simultaneous high-speed videos and passive acoustics maps were acquired for a single oscillating bubble in a simple setup. Machine learning methods such as support vector machine and convolutional neural networks were used to classify the acoustic data and train a classification algorithm. The algorithm was then adapted for a novel sonochemical reactor that induces spontaneous cavitation nucleation and utilizes a passive cavitation detector to monitor cavitation noise. Further research will be done in correlating potassium iodide (KI) degradation with frequency spectra using ML classification in order to optimize the sonochemical efficiency.
MoreTranslated text
Key words
cavitation,machine-learning machine-learning techniques,machine-learning machine-learning,noise,classification
AI Read Science
Must-Reading Tree
Example
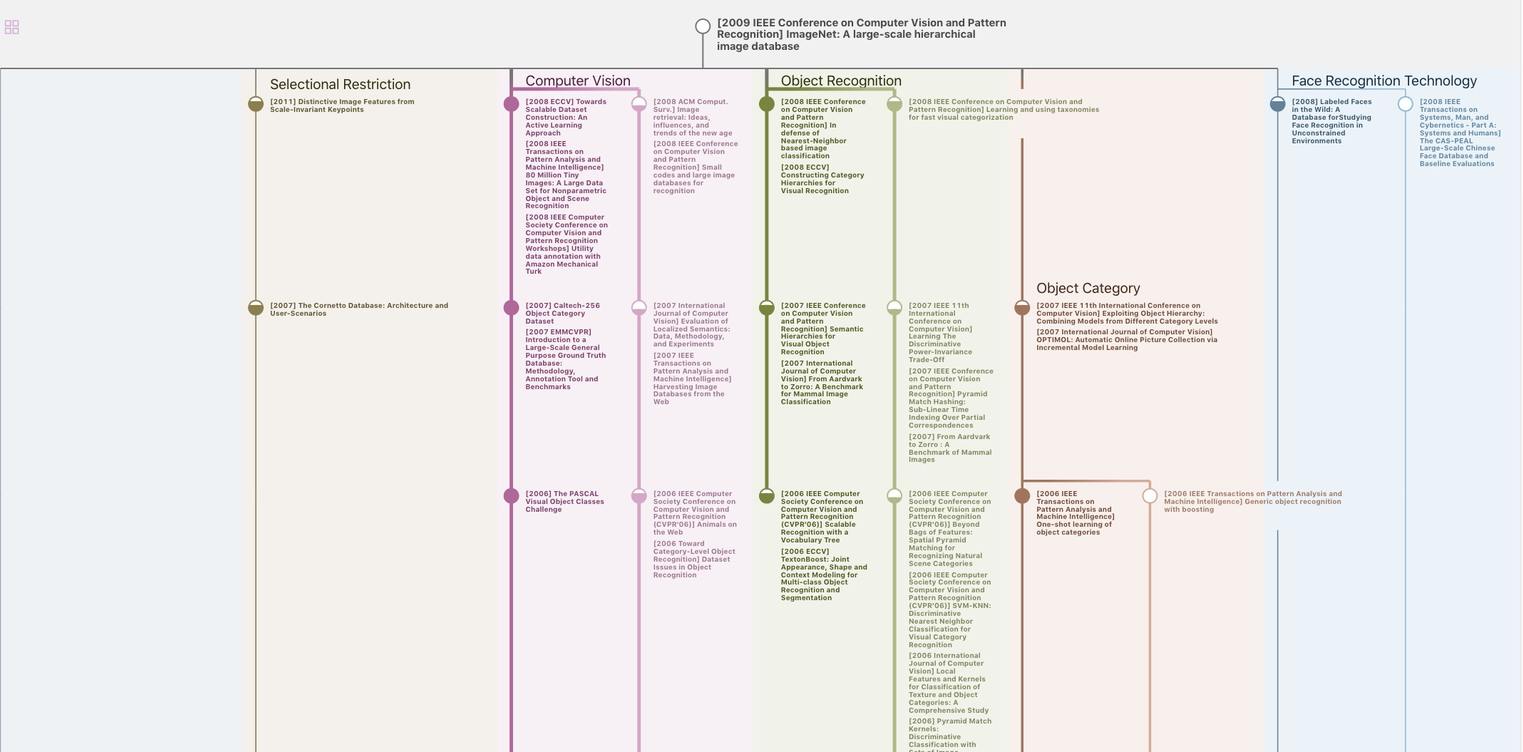
Generate MRT to find the research sequence of this paper
Chat Paper
Summary is being generated by the instructions you defined