Integration of bioinformatics analysis and machine learning to identify diagnostic biomarkers and molecular mechanisms of osteoporosis in association with depression
Research Square (Research Square)(2023)
摘要
Abstract Background Both osteoporosis and depression are chronic diseases that cause serious public health problems worldwide. Accumulating evidence suggests that depression is associated with osteoporosis; however, the common mechanisms underlying its pathogenesis are not fully understood. In this study, we used bioinformatics techniques to discover significant diagnostic feature genes in patients with osteoporosis and depression and further investigated the molecular pathways underlying osteoporosis and depression. Methods We acquired microarray datasets from the gene expression omnibus database for osteoporosis and depression. To identify diagnostic feature genes in peripheral blood samples, we used weighted gene co-expression network analysis (WGCNA) and machine learning algorithms, such as least absolute shrinkage and selection operator regression, random forest, and support vector machine-recursive feature elimination. We developed nomogram models and receiver operating characteristic (ROC) curves and validated them using an external dataset. Transcription factors and microRNAs were obtained from the JASPER and RegNetwork repositories and used to construct coregulatory networks. The DSigDB database was used to identify potential gene-targeting drugs. In addition, we performed an enrichment analysis and screened common genes from differentially expressed genes, WGCNA, and public databases to identify hub genes. Results From the combined WGCNA results, 450 common genes were identified to build a protein interaction network, and 104 node genes were selected based on the number of proximity nodes. Based on the results of the application of several machine learning algorithms for the analysis of osteoporosis samples, nine diagnostic feature genes were selected for nomogram construction and diagnostic value assessment. Both the training and validation sets showed that the nomogram had a good diagnostic value (ROC, 0.910–0.942). According to the findings of the common gene analysis, oxidative stress, immune system responses, and inflammatory responses may represent shared pathophysiological pathways between depression and osteoporosis. Conclusion Nine diagnostic feature genes were identified and a nomogram for potential osteoporosis screening of populations with depression was constructed. The findings provide novel insights into the pathogenesis of depression and osteoporosis. Our study provides a basis for the diagnosis of osteoporosis in the peripheral blood samples of populations with depression and is expected to provide new clues for further experimental investigations.
更多查看译文
关键词
osteoporosis,bioinformatics analysis,diagnostic biomarkers
AI 理解论文
溯源树
样例
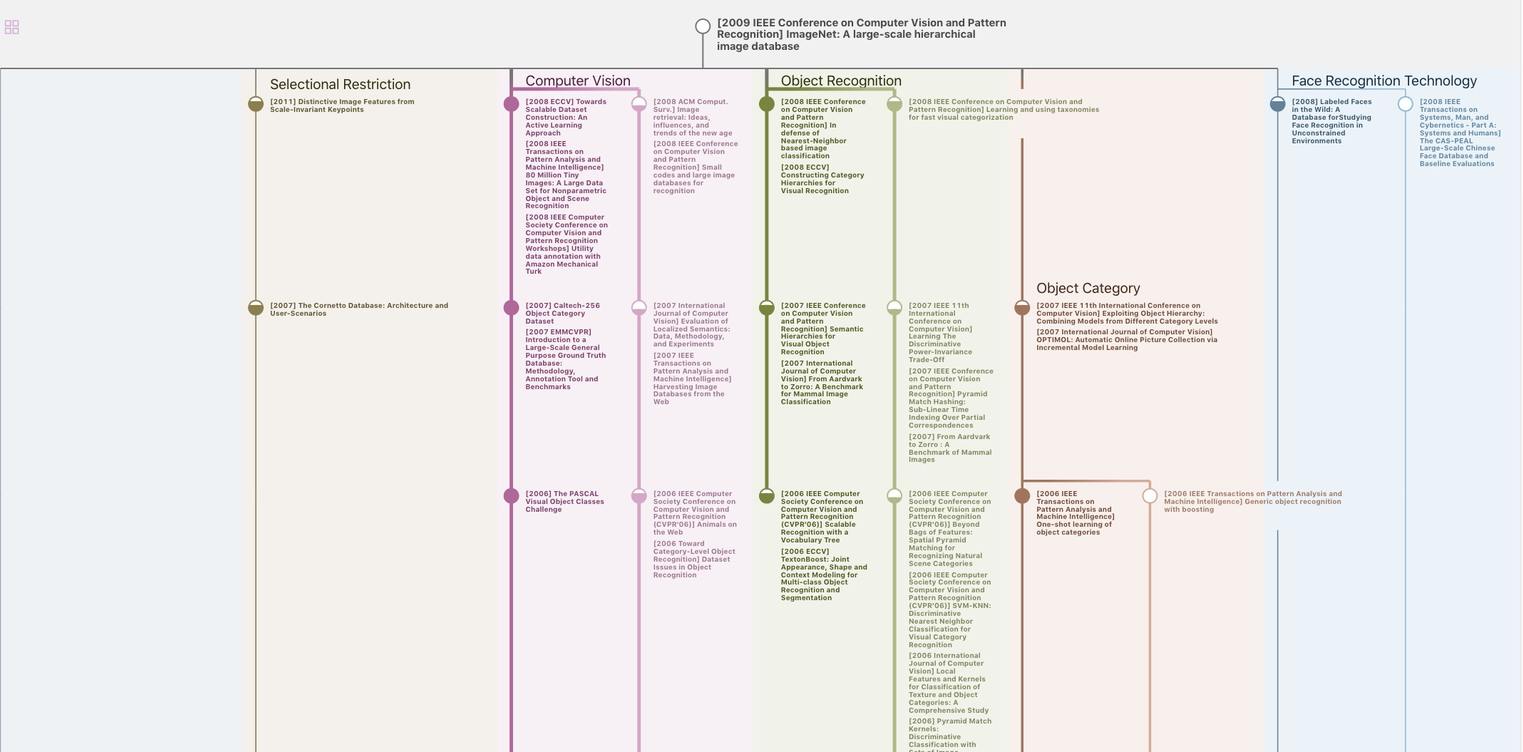
生成溯源树,研究论文发展脉络
Chat Paper
正在生成论文摘要