Abstract 4276: Recovering false negatives in CRISPR fitness screens with JLOE
Cancer Research(2023)
摘要
Abstract It is widely accepted that pooled library CRISPR-Cas9 knockout screens offer greater sensitivity and specificity than prior technologies in detecting essential genes whose disruption leads to fitness defects, a critical step in identifying candidate cancer targets. However, the assumption that CRISPR screens are saturating has been largely untested and it is still poorly understood whether possible systematic biases in CRISPR screens affect our understanding of human cell-intrinsic gene essentiality. In the absence of a ground truth, the actual true positive, false positive and false negative rates of a typical genome-wide CRISPR-Cas9 knockout screen are still unknown. Through integrated analysis of screen data in hundreds of cancer cell lines generated by the Cancer Dependency Map, we examine some of the biases characteristic of genome-wide CRISPR-Cas9 knockout screening. With our computational modeling approaches, we estimate the true positive rate, false positive rate and show that a typical CRISPR screen has a ~20% false negative rate, in addition to library-specific false negatives. Furthermore, replicability of essential gene hits falls sharply as gene expression decreases, while cancer subtype-specific genes within a tissue show distinct profiles compared to false negatives. With our cumulative analyses across tissues, we identified an improved new set of core essential genes, which, like the previous set, can be used as a metric for the quality control of genome-wide CRISPR-Cas9 knockout screens. Our results also suggest only a small number of lineage-specific essential genes, with the overwhelming majority of genes showing overlap between related tissues and are enriched for transcription factors that define pathways of tissue differentiation. To recover false negatives in these genome- wide CRISPR knockout screens, we introduce a method called Joint Log Odds of Essentiality (JLOE), which extends fundamental concepts from our Bayesian framework of BAGEL algorithm to improve analysis across multiple cell line screens. JLOE uses information derived from previous screens to update the probability of essentiality of each screen based on prior observations. We show that JLOE selectively rescues the false negatives without an increased false discovery rate and improves screen concordance with the Sanger dataset, reducing the number of background-specific essential genes. Overall, our methods offer a significantly improved analysis of genome-wide CRISPR-Cas9 knockout screens, detecting and correcting for the previously unidentified false negatives and enhancing our identification of cancer-specific vulnerabilities as therapeutic targets. Citation Format: Merve Dede, Traver Hart. Recovering false negatives in CRISPR fitness screens with JLOE. [abstract]. In: Proceedings of the American Association for Cancer Research Annual Meeting 2023; Part 1 (Regular and Invited Abstracts); 2023 Apr 14-19; Orlando, FL. Philadelphia (PA): AACR; Cancer Res 2023;83(7_Suppl):Abstract nr 4276.
更多查看译文
关键词
crispr fitness screens
AI 理解论文
溯源树
样例
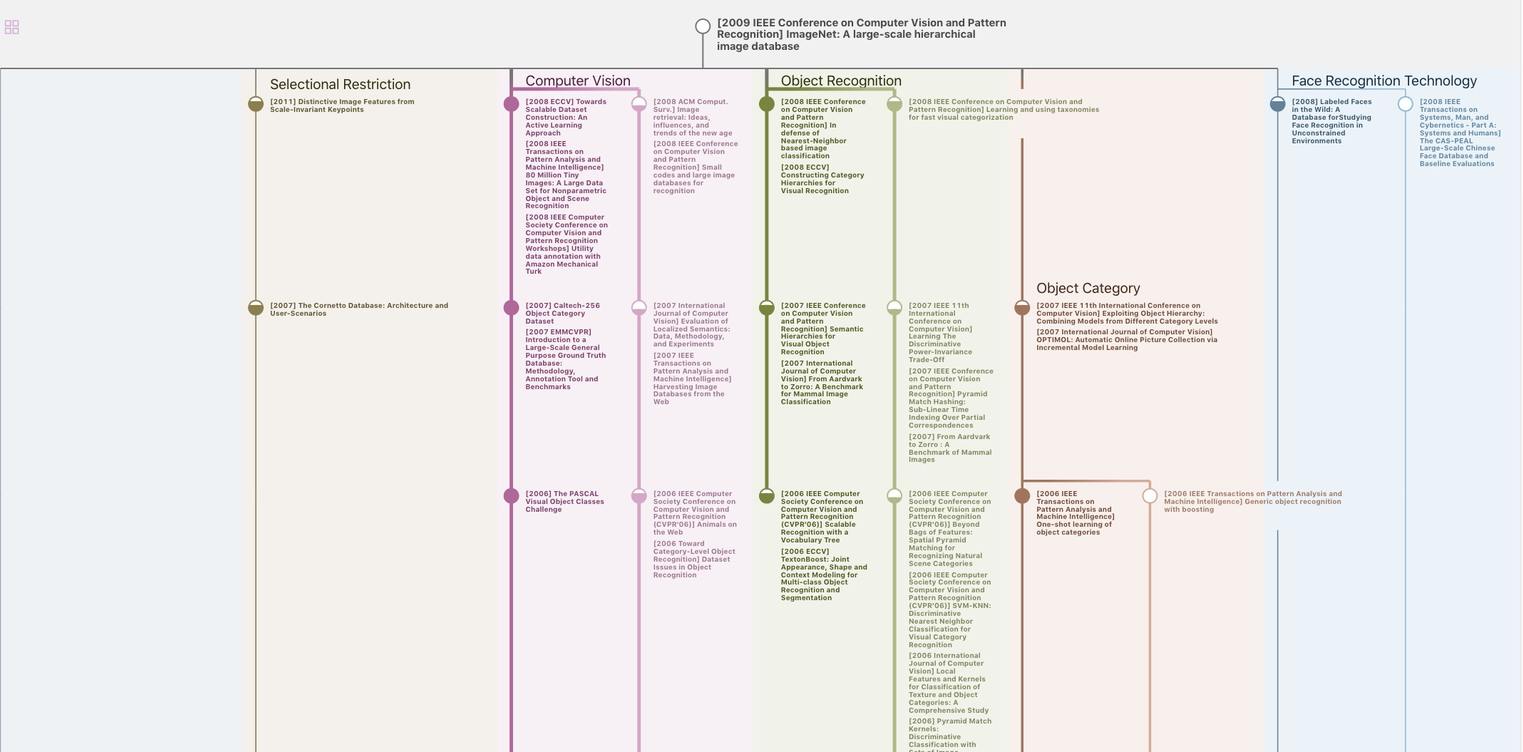
生成溯源树,研究论文发展脉络
Chat Paper
正在生成论文摘要