Adaptive PHD Filter with RCS and Doppler Feature for Space Targets Tracking via Space-Based Radar
IEEE Transactions on Aerospace and Electronic Systems(2023)
Abstract
Discrimination of approaching multiple space targets is a challenging task for space situational awareness (SSA). Compared with ground-based radars, space-based radars cover better coverage and they have no restriction on the curvature of the earth. In this paper, we utilize space-based radar to track objects in space. Note that radar cross section (RCS) characteristics and Doppler information are typical potential target individual features to improve the tracking performance for SSA tasks. However, one of the limitations of utilizing the RCS information is that the fluctuation of targets dynamic RCS is modeled by the typical
$\chi^2$
distribution or Weibull distribution. In practice, fluctuating RCS of typical space targets such as mission-focused space targets, fit poorly with the above distribution models under some altitude angles. To tackle this problem, we proposed a mixed log-normal (MLN) distribution model to compute the intensities of time-varying dynamic RCS likelihood. Then the dynamic RCS likelihood and Doppler information are incorporated into the update recursion of the standard probability hypothesis density (PHD) filter. Furthermore, considering that multiple space-closed targets are misjudged as one under space targets tracking scenarios, an adaptive threshold is devised to merge the Gaussian mixture (GM) components weights to ease this phenomenon simultaneously. The number estimation preservation proof is given and the GM implementation of the proposed filter is also provided in this paper. Simulation results validate the effectiveness and robustness of the proposed RCS & Doppler information aided & GM weights improved probability hypothesis density (RDGI-PHD) filter.
MoreTranslated text
Key words
Space-based radar,space targets tracking,radar cross section (RCS),probability hypothesis density (PHD) filter,adaptive Gaussian mixture (GM) weights merging threshold
AI Read Science
Must-Reading Tree
Example
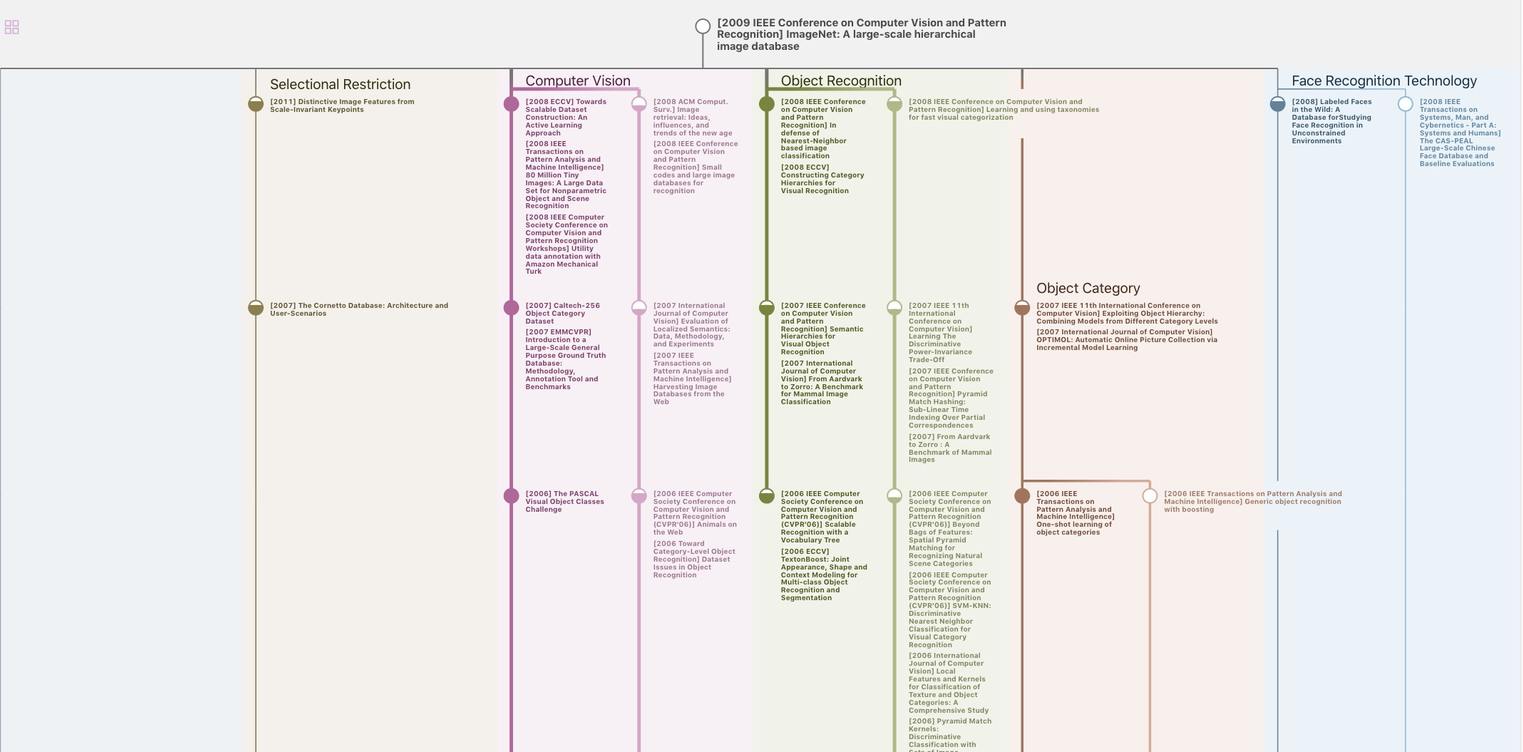
Generate MRT to find the research sequence of this paper
Chat Paper
Summary is being generated by the instructions you defined