Integrating Language Guidance Into Image-Text Matching for Correcting False Negatives
IEEE TRANSACTIONS ON MULTIMEDIA(2024)
摘要
Image-Text Matching (ITM) aims to establish the correspondence between images and sentences. ITM is fundamental to various vision and language understanding tasks. However, there are limitations in the way existing ITM benchmarks are constructed. The ITM benchmark collects pairs of images and sentences during construction. Therefore, only samples that are paired at collection are annotated as positive. All other samples are annotated as negative. Many correlations are missed in these samples that are annotated as negative. For example, a sentence matches only one image at the time of collection. Only this image is annotated as positive for the sentence. All other images are annotated as negative. However, these negative images may contain images that correspond to the sentences. These mislabeled samples are called false negatives. Existing ITM models are optimized based on annotations containing mislabels, which can introduce noise during training. In this paper, we propose an ITM framework integrating Language Guidance (LG) for correcting false negatives. A language pre-training model is introduced into the ITM framework to identify false negatives. To correct false negatives, we propose language guidance loss, which adaptively corrects the locations of false negatives in the visual-semantic embedding space. Extensive experiments on two ITM benchmarks show that our method can improve the performance of existing ITM models. To verify the performance of correcting false negatives, we conduct further experiments on ECCV Caption. ECCV Caption is a verified dataset where false negatives in annotations have been corrected. The experimental results show that our method can recall more relevant false negatives.
更多查看译文
关键词
Correcting false negatives,image-text matching,language guidance
AI 理解论文
溯源树
样例
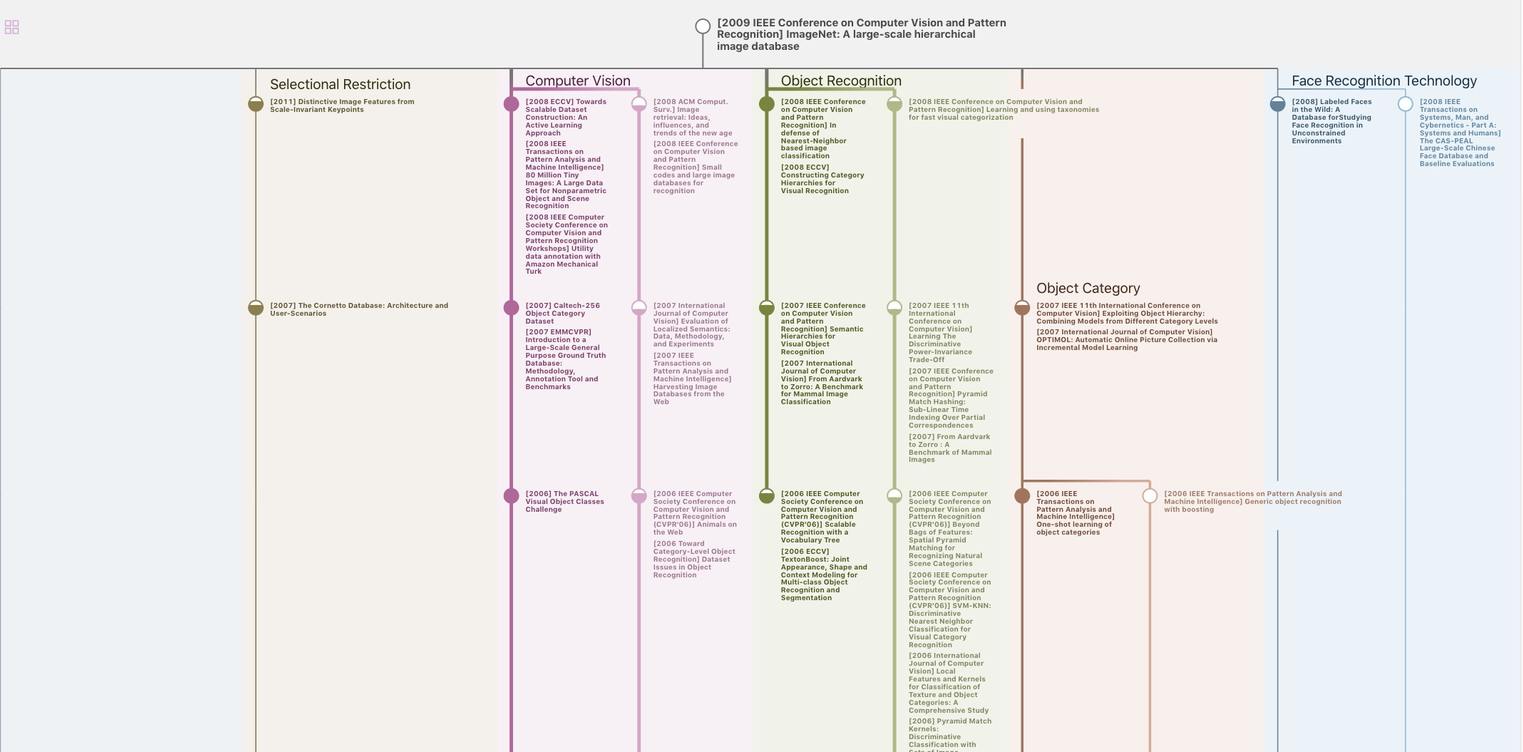
生成溯源树,研究论文发展脉络
Chat Paper
正在生成论文摘要