Fast and Scalable Unsupervised Deep Subgraph Anomaly Detection
Research Square (Research Square)(2023)
摘要
Abstract Mining anomalous subgraphs in networks is a crucial task in various application scenarios, such as disease outbreak detection, financial fraud detection, and activity monitoring in social networks. However , identifying anomalous subgraphs is a challenging problem due to their complex topological structures, high-dimensional attributes, various notions of anomalies, and the exponentially large subgraph searching space in a given graph. Classical shallow models typically rely on handcrafted anomaly measure functions, which are not effective when prior knowledge is unavailable. To address this issue, deep learning-based methods have been proposed as an end-to-end way of learning anomaly measure functions. However, while these methods have been successful in detecting node-level, edge-level, and graph-level anomalies, detecting anomalous subgraphs has been largely underex-plored due to difficulties in subgraph representation learning, lackness of supervision, end-to-end anomaly quantification, and their scalability. In this paper, we propose a novel deep framework called the Anomalous Subgraph Autoencoder (AS-GAE) to extract anomalous subgraphs in an unsupervised and weakly-supervised manner. To enhance the scal-ability of our proposed model on large-scale graphs, we further design an edge sampling technique that reduces the time and memory complexity from a quadratic term of the number of nodes to a linear term of the number of edges. Our proposed method is demonstrated to be superior in terms of accuracy and efficiency through extensive experiments on two synthetic datasets and nine real-world datasets.
更多查看译文
关键词
detection
AI 理解论文
溯源树
样例
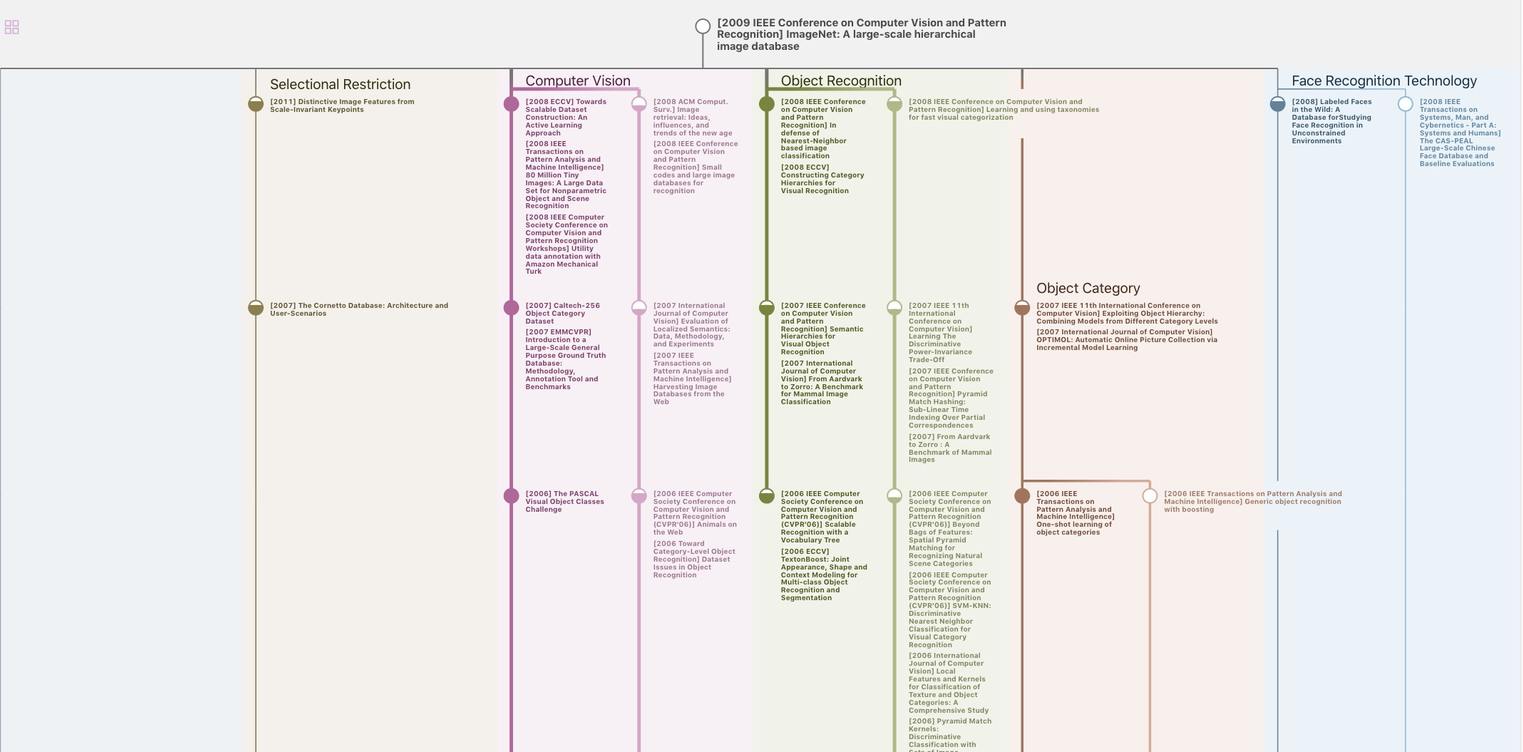
生成溯源树,研究论文发展脉络
Chat Paper
正在生成论文摘要