BrainCLIP: Bridging Brain and Visual-Linguistic Representation Via CLIP for Generic Natural Visual Stimulus Decoding
arXiv (Cornell University)(2023)
摘要
Due to the lack of paired samples and the low signal-to-noise ratio of functional MRI (fMRI) signals, reconstructing perceived natural images or decoding their semantic contents from fMRI data are challenging tasks. In this work, we propose, for the first time, a task-agnostic fMRI-based brain decoding model, BrainCLIP, which leverages CLIP's cross-modal generalization ability to bridge the modality gap between brain activity, image, and text. Our experiments demonstrate that CLIP can act as a pivot for generic brain decoding tasks, including zero-shot visual categories decoding, fMRI-image/text matching, and fMRI-to-image generation. Specifically, BrainCLIP aims to train a mapping network that transforms fMRI patterns into a well-aligned CLIP embedding space by combining visual and textual supervision. Our experiments show that this combination can boost the decoding model's performance on certain tasks like fMRI-text matching and fMRI-to-image generation. On the zero-shot visual category decoding task, BrainCLIP achieves significantly better performance than BraVL, a recently proposed multi-modal method specifically designed for this task. BrainCLIP can also reconstruct visual stimuli with high semantic fidelity and establishes a new state-of-the-art for fMRI-based natural image reconstruction in terms of high-level semantic features.
更多查看译文
关键词
generic natural visual-linguistic stimulus,bridging brainclip
AI 理解论文
溯源树
样例
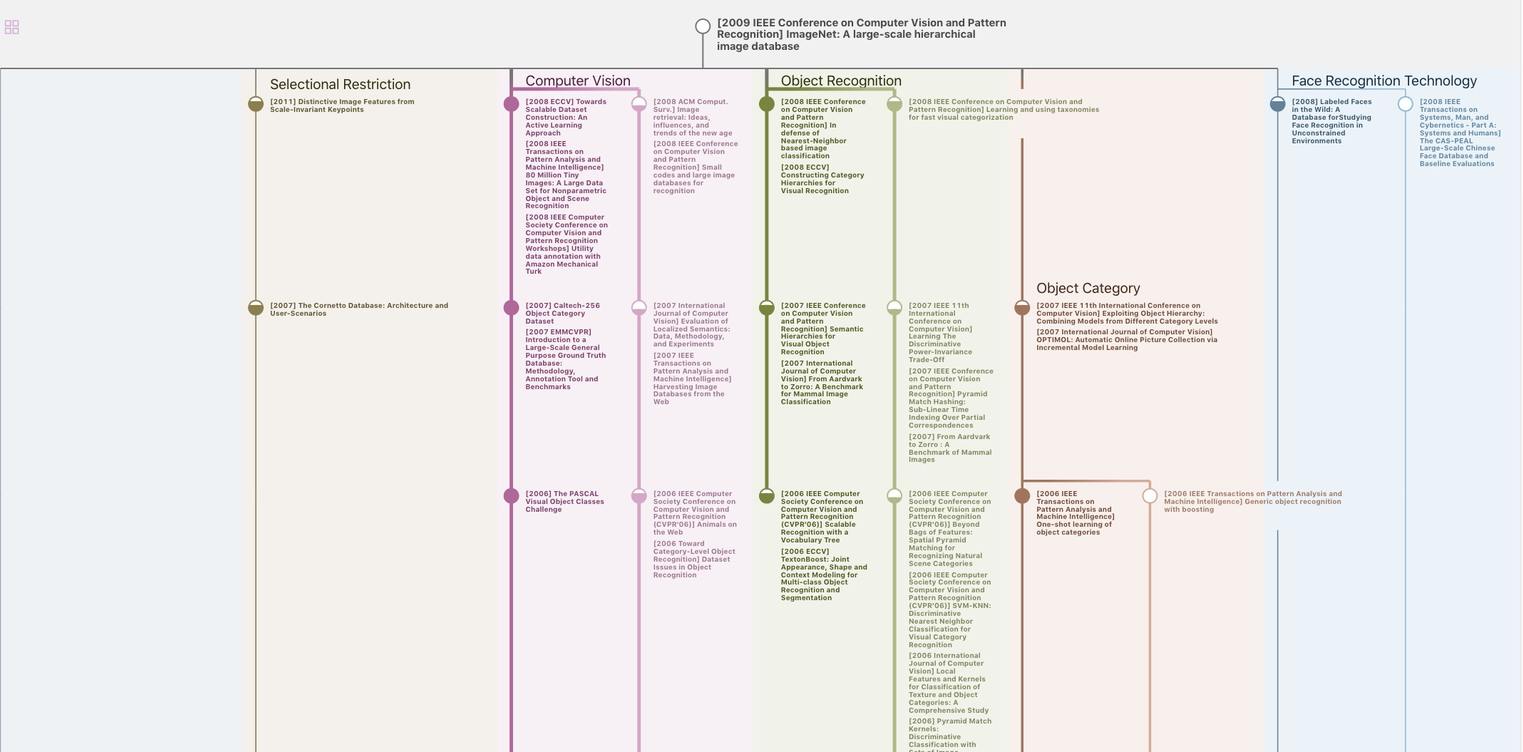
生成溯源树,研究论文发展脉络
Chat Paper
正在生成论文摘要