Optimization of porous layer structure of high-temperature proton exchange membrane fuel cell based on deep learning and Monte Carlo method
INTERNATIONAL JOURNAL OF HYDROGEN ENERGY(2024)
摘要
The optimization of the membrane electrode assembly (MEA) is a crucial aspect in improving the performance of high-temperature proton exchange membrane fuel cells (HT-PEMFCs). Through experimental validation, a three-dimensional HT-PEMFC computational fluid dynamics (CFD) model was established to simulate the transport of charge, mass, and momentum across various porous layers. A deep neural network (DNN) was designed to quantify the influence of porous layer structures on cells performance and determined the optimal solution of the MEA parameters for different Pt loadings. The training data of DNN were generated using 11 porous layer structural parameters selected via a Monte Carlo method, and their corresponding current densities predicted by the validated HT-PEMFC CFD model. The DNN model showed an average relative error of only 0.76% in predicting current density when compared to CFD simulations. Using a biobjective genetic algorithm (GA) in conjunction with the DNN model, the optimized cell power densities at a voltage of 0.4 V and Pt loadings of 0.3 mg/cm2 and 0.5 mg/cm2 are 18% and 9% higher than the maximum values reported in the literature, respectively. The proposed approach combining DNN model and GA can serve as a useful guide for the design of HT-PEMFC in practical applications.(c) 2023 Hydrogen Energy Publications LLC. Published by Elsevier Ltd. All rights reserved.
更多查看译文
关键词
HT-PEMFC,DNN,Monte Carlo,Bi-objective genetic algorithm
AI 理解论文
溯源树
样例
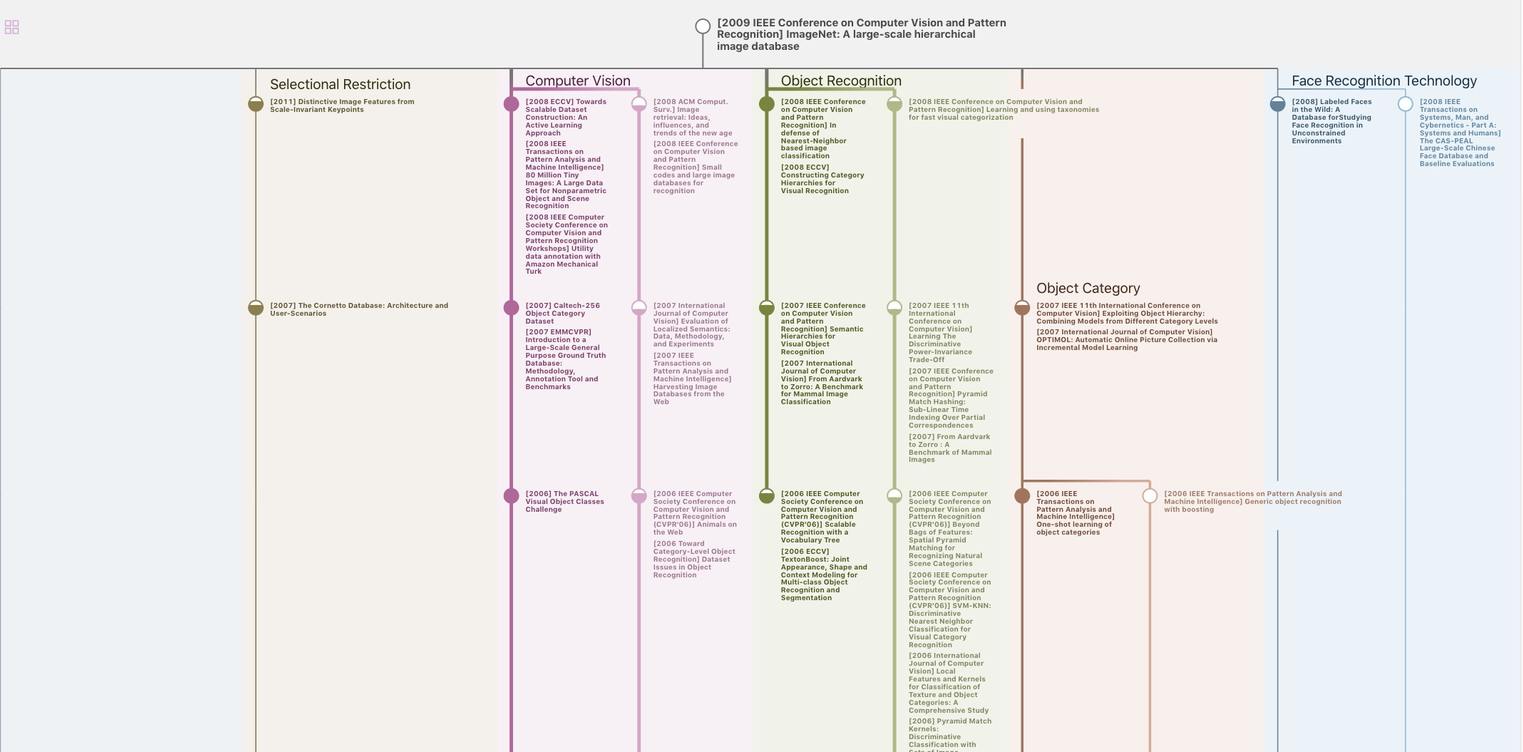
生成溯源树,研究论文发展脉络
Chat Paper
正在生成论文摘要