Multimodal Diagnosis of COVID-19 Using Deep Wavelet Scattering Networks
Internet of things(2023)
摘要
Since December 2019, healthcare systems worldwide have been challenged with the COVID-19 pandemic. Within the computer research community, efforts have been directed towards the potential of artificial intelligence in the diagnosis of COVID-19 cases. Majority of the proposed methods have been based on Convolutional neural network (CNN). Nevertheless, CNN-based methods continue to be challenged in settings with limited resources. In an attempt to alleviate this challenge within the COVID-19 context, this chapter presents a novel method for identifying COVID-19 in X-ray and CT scan images based on Deep Wavelet Scattering Networks (DWSN) and subspace learning. Wavelet scattering networks are used to learn a deep invariant representation from the training set. Subspace learning is then achieved through a joint best non-linear approximation approach in the wavelet-scattering domain. The proposed method is used through a unified framework for single-modal and multi-modal diagnosis of COVID-19. Several experiments were conducted to evaluate the performance of the proposed method. Experimental results have demonstrated the capabilities of the proposed method to identify COVID-19, in X-ray and CT-scan images with state-of-the-art accuracies. Wavelet Scattering-based multi-modal fusion resulted in an average accuracy of 98.77% with improvements of 1.16% and 2.81% compared to single modality X-ray and CT scan diagnosis, respectively.
更多查看译文
关键词
diagnosis
AI 理解论文
溯源树
样例
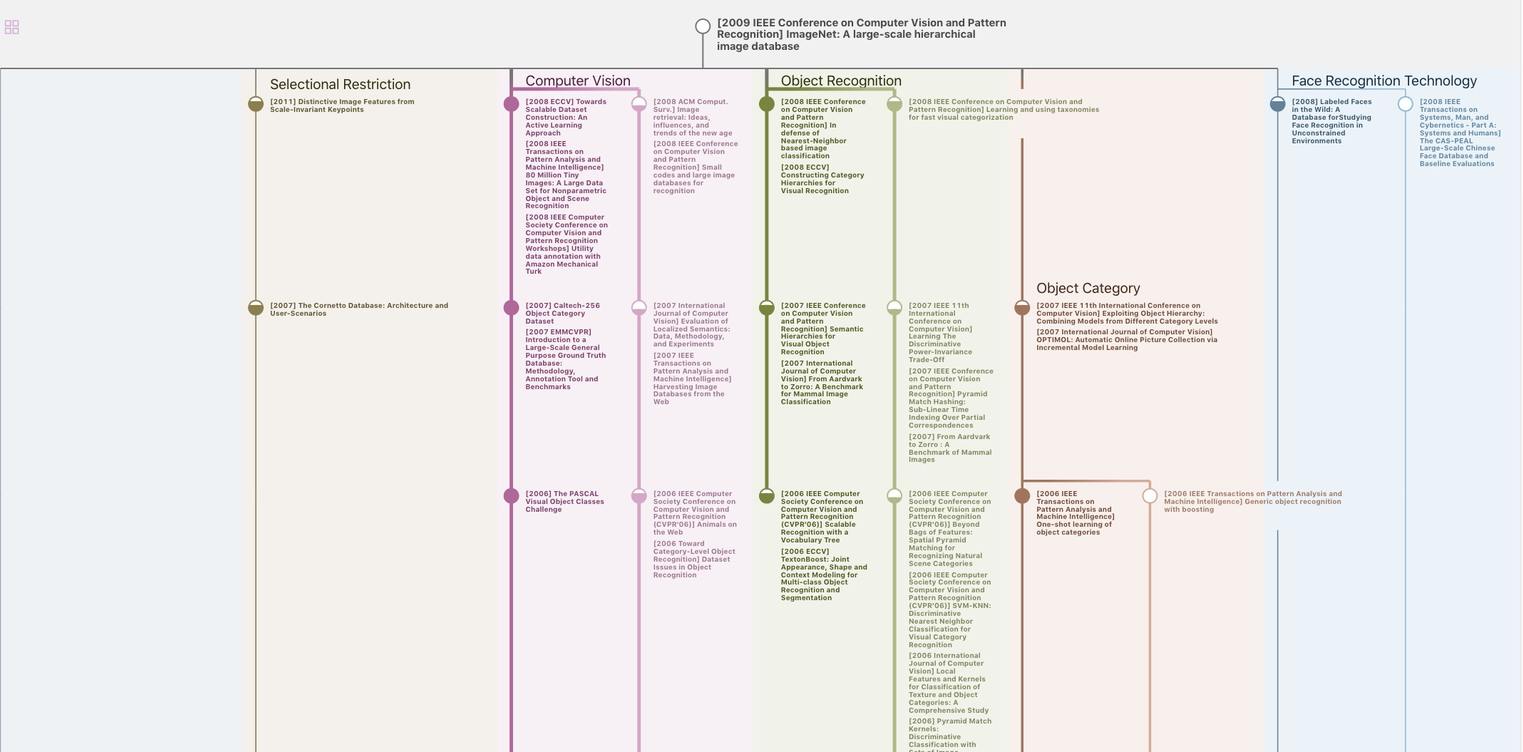
生成溯源树,研究论文发展脉络
Chat Paper
正在生成论文摘要