Adaptive Horizontal Federated Learning-Based Demand Response Baseline Load Estimation
IEEE TRANSACTIONS ON SMART GRID(2024)
Abstract
To improve the operational security and reliability of distribution networks, distribution network operators will encourage customers to modify their demand profiles and then give them financial compensation. The compensation is always settled based on customer baseline load (CBL). The estimation of CBL can be achieved using both traditional historical data methods and non-participant data methods. However, relying solely on a single method for estimating baseline load for some residential customers may result in significant estimation errors. To estimate baselines for residential loads under different conditions, this paper proposes a novel deep learning method that intelligently selects historical data-based CBL and non-participant data-based CBL. This method provides a special way for taking advantage of the historical load data of demand response (DR) participant and the concurrent load data of nonparticipants. Moreover, to preserve the privacy of residential customers, a horizontal federated learning framework is proposed to train local neural networks in residential units. In the federated learning framework, a novel adaptive learning rate method is proposed to improve the performance of local neural networks under low global aggregation frequency. Compared with the state-of-the-art CBL estimation methods, the improved federated learning method can significantly reduce the seriously inaccurate estimation and improve the overall performance of CBL estimation under various condition. The effectiveness and superiority of the proposed method have been verified on a real-world load dataset.
MoreTranslated text
Key words
Estimation,Federated learning,Load modeling,Pattern matching,Neural networks,Deep learning,Adaptation models,Customer baseline load (CBL),deep learning,horizontal federated learning,privacy-preserving,homomorphic encryption,logistic chaotic map
AI Read Science
Must-Reading Tree
Example
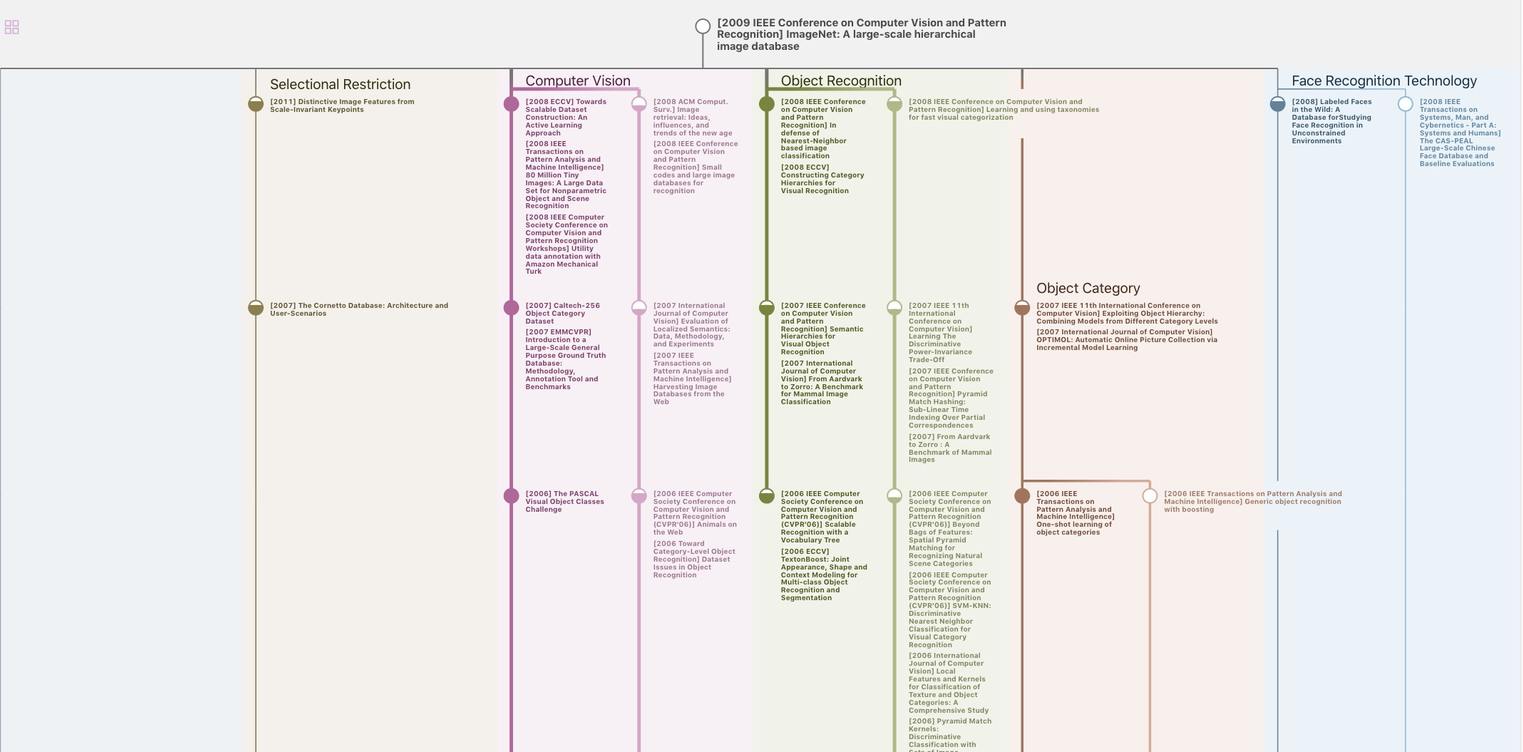
Generate MRT to find the research sequence of this paper
Chat Paper
Summary is being generated by the instructions you defined