Fluid Identification Using XGBoost Combined with MAHAKIL in Low-Permeability Reservoirs
Spe Journal(2024)
Abstract
Low -resistance reservoirs have been of great interest as a key topic in the study of low -permeability reservoirs. Accurate identification of fluid properties is a challenging problem in the effective exploration of such reservoirs. Logging data, as a common identification tool, can provide rich and highly accurate geological information. Here, we combine extreme gradient boosting (XGBoost) and MAHAKIL's oversampling method for fluid property identification using logging data from low -permeability sandstone reservoirs. The MAHAKIL method is used to solve the class imbalance problem due to unbalanced training samples, and the data are fed into XGBoost to build a geological model with complex abstract feature weights related to fluid properties through multiple decision trees. We first demonstrate that MAHAKIL can improve the XGBoost model accuracy using four evaluation criteria, namely, the F1 -score, recall, precision, and accuracy, among which the F1 -score is most applicable to the classification problem of reservoir fluid prediction. Then, the receiver operating characteristic (ROC) and area under the curve (AUC) values are used to demonstrate that MAHAKIL improves the XGBoost model performance. Finally, the results and performance of this method and the traditional XGBoost model with unbalanced real data are compared. The results show that the proposed method is superior for fluid property identification in low -permeability sandstone reservoirs with imbalanced learning samples.
MoreTranslated text
Key words
fluid identification,xgboost,low-permeability
AI Read Science
Must-Reading Tree
Example
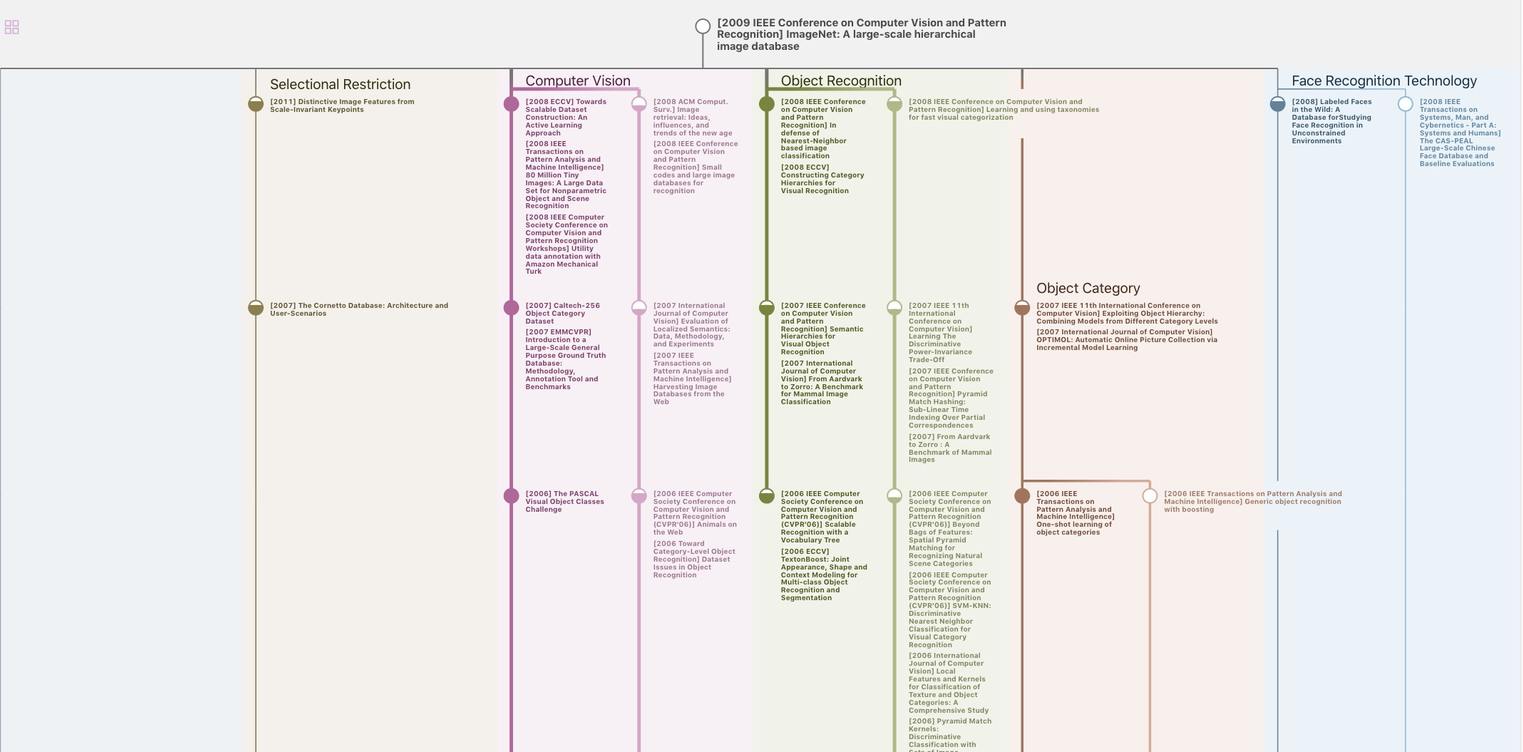
Generate MRT to find the research sequence of this paper
Chat Paper
Summary is being generated by the instructions you defined