Visually Interpretable Fuzzy Neural Classification Network With Deep Convolutional Feature Maps
IEEE TRANSACTIONS ON FUZZY SYSTEMS(2024)
摘要
This article proposes a deep feature map-based fuzzy neural classification network (DFM-FNCN) with applications to shape-based classification problems. The DFM-FNCN is characterized by compact and visually interpretable fuzzy if-then rules. Classification features in the DFM-FNCN are obtained from feature maps in a deep convolutional neural network. The DFM-FNCN employs the divide-and-conquer technique, where a feature map-based fuzzification operation is proposed to find the firing strength of a fuzzy rule, to address the curse of dimensionality problem. The structure and parameters of the DFM-FNCN are learned through online rule generation and gradient descent algorithms, respectively. For visual interpretation of the learned fuzzy rules, this article designs a deep decoder to map the antecedent of a fuzzy rule to an object-shaped image. The inference behavior of a fuzzy rule is interpreted by inspecting the relationship between the visualized antecedent and the classification levels of each class in the consequent. To speed up retraining of the DFM-FNCN in a new scenario, this article proposes a method to select representative retraining images in a low-dimensional fuzzy rule-mapped space. The DFM-FNCN is applied to classify human postures and moving objects. Experimental results show the advantages of high classification accuracy, model interpretability, and retraining abilities of the DFM-FNCN.
更多查看译文
关键词
Feature extraction,Convolutional neural networks,Artificial intelligence,Visualization,Decoding,Image classification,Firing,deep learning,explainable artificial intelligence (AI),fuzzy classifiers,fuzzy neural networks,interpretable fuzzy systems
AI 理解论文
溯源树
样例
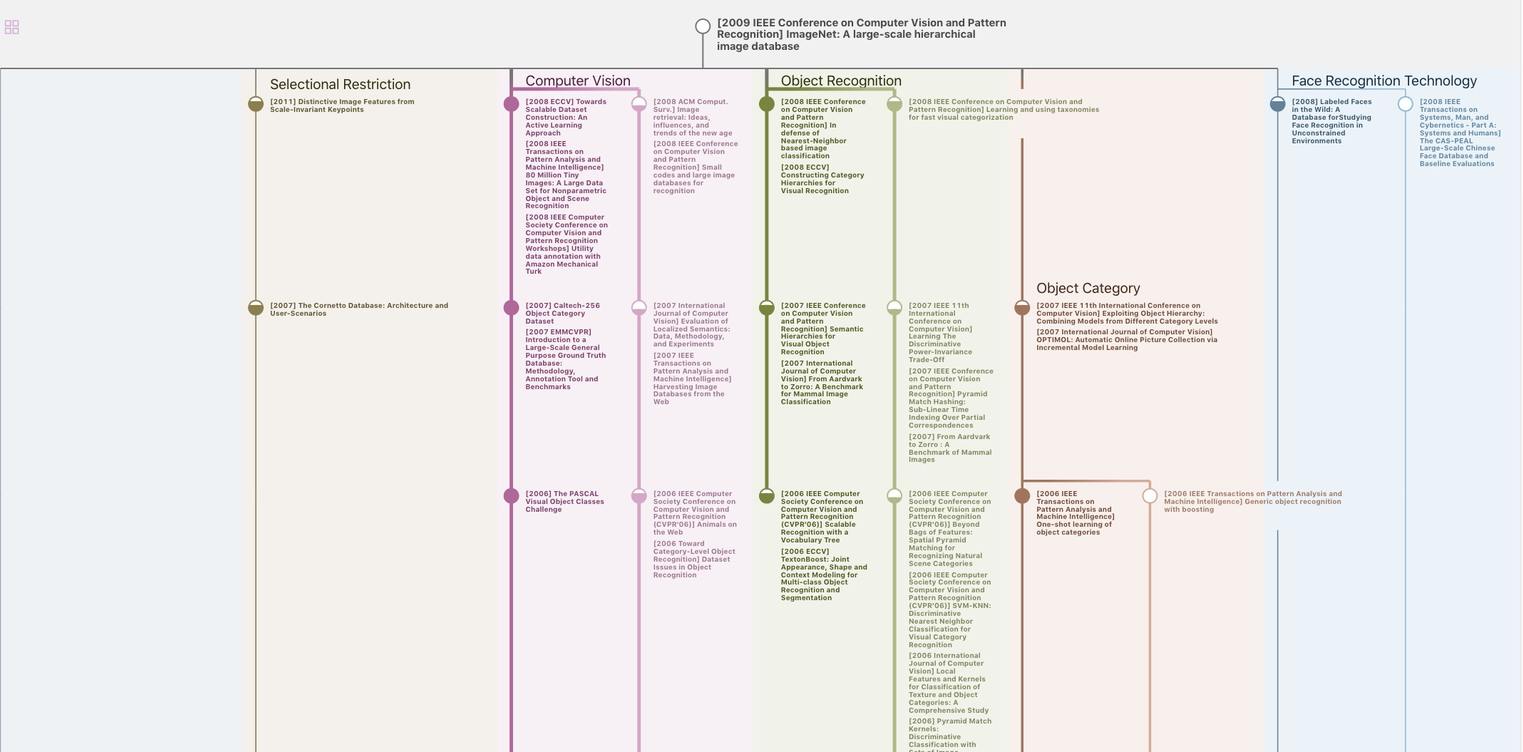
生成溯源树,研究论文发展脉络
Chat Paper
正在生成论文摘要