Onsite Early Prediction of Peak Amplitudes of Ground Motion Using Multi-scale STFT Spectrogram
Research Square (Research Square)(2023)
摘要
Abstract On-site earthquake early warning (EEW) techniques, relying on single-station seismic wave measurements, have demonstrated efficacy in mitigating damage caused by destructive earthquakes. Traditionally, these methods leverage diverse P-wave attributes from the initial seismic wave seconds subsequent to the trigger event to estimate earthquake intensity and potential harm. Recent advancements in deep learning, particularly convolutional neural networks (CNN), have introduced several approaches for predicting peak ground amplitudes in EEW, yielding promising outcomes. In this study, we propose employing a multi-scale short-time frequency transform spectrogram as the input for a CNN prediction model to enable early on-site estimation of peak ground acceleration, velocity, and displacement. We evaluate the predictive accuracy for earthquakes containing low-frequency components and contrast the results against alternative methods utilizing wavelet packet transform spectrogram and a combined input of time history and Fourier spectrum. Our findings indicate reduced errors in the predicted peak ground motion amplitudes using the proposed methodology, particularly in relation to peak ground velocity and displacement, as compared to the alternative approaches investigated.
更多查看译文
关键词
ground motion,peak amplitudes,early prediction,multi-scale
AI 理解论文
溯源树
样例
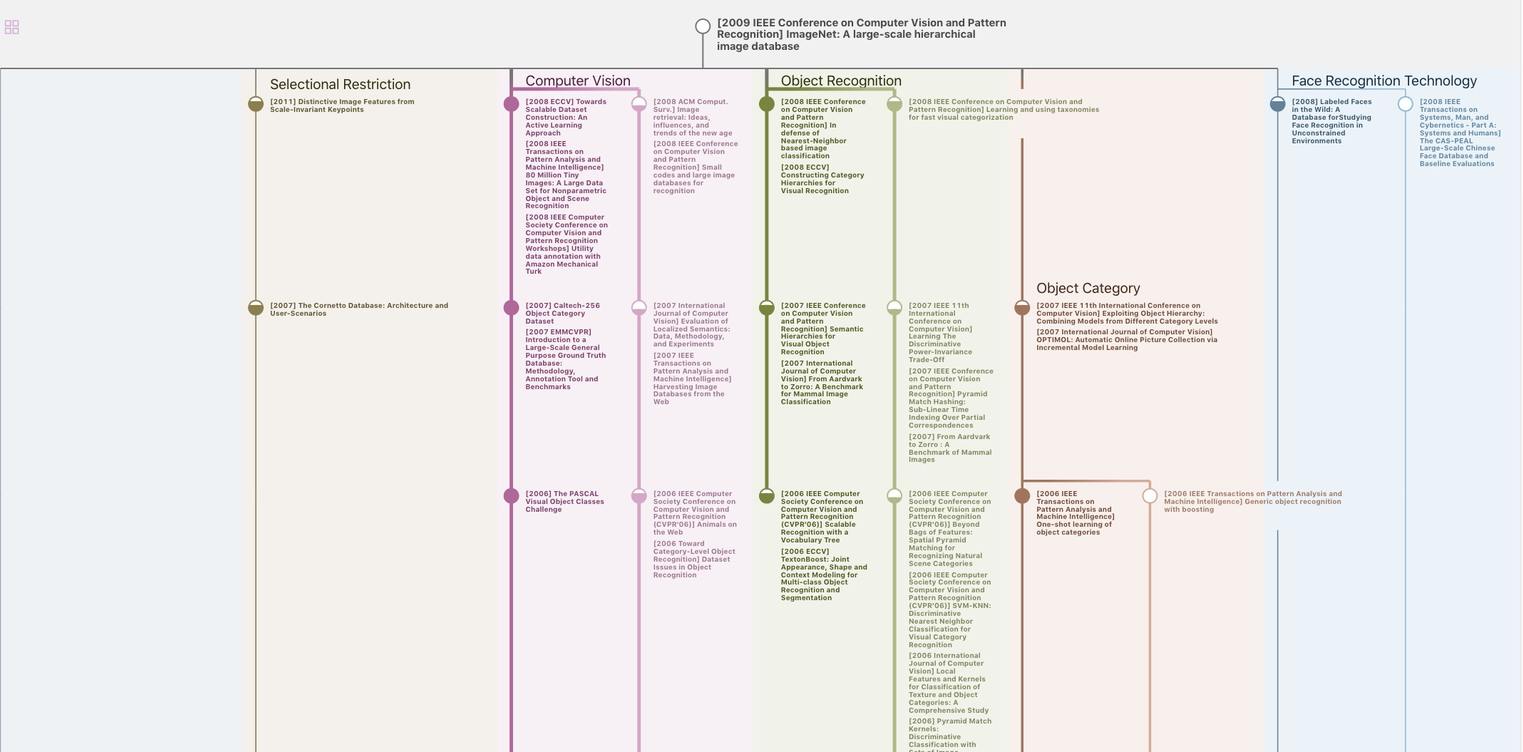
生成溯源树,研究论文发展脉络
Chat Paper
正在生成论文摘要