Hinging on Success: Leveraging the Power of CAR T-Cell Therapy through In-Silico Modeling of Hinge Length and Epitope Location
Journal of Immunology(2023)
摘要
Abstract Genetic engineering of T-cells using chimeric antigen receptors (CARs) has shown promise in the treatment of relapsed/refractory B-cell leukemia. However, a significant percentage of patients will eventually relapse, often due to the emergence of tumor cells expressing low levels of the target antigen. Previous research has demonstrated that the hinge domain of the CAR plays a crucial role in regulating the cytotoxic capabilities of these cells. In this study, we assessed the potential to model optimal hinge length for a given single-chain variable fragment (scFv) in relation to the location of the targeted epitope. We designed an array of hinges originating from CD8α and incorporated these hinges into CARs targeting CD19, CD22, CD33, and CD20 antigens. We found that the location of the epitope is critical in determining the optimal hinge; a narrow range of short hinges is more effective against membrane-distal epitopes while long hinges are more effective against membrane-proximal epitopes. To build an in-silico model, we used AlphaFold2 (AF2), reducing the length of the multiple sequence alignment (MSA) clusters to introduce stochasticity and increase the conformational diversity of the structural predictions for the CAR hinges and target proteins. We discovered that CARs with a predicted intermembrane distance of 15nm between the CAR and ligand were particularly effective against antigen-low leukemic cells. Our findings suggest that intermembrane distance can predict CAR responsiveness against a specific epitope, enabling the rapid optimization of CARs by adjusting hinge length. The development of this modeling strategy will facilitate the design of CARs with optimized cytotoxic potential against a wide range of novel targets. Intramural Research Program of the National Cancer Institute (Bethesda, MD)
更多查看译文
关键词
hinge length,t-cell,in-silico
AI 理解论文
溯源树
样例
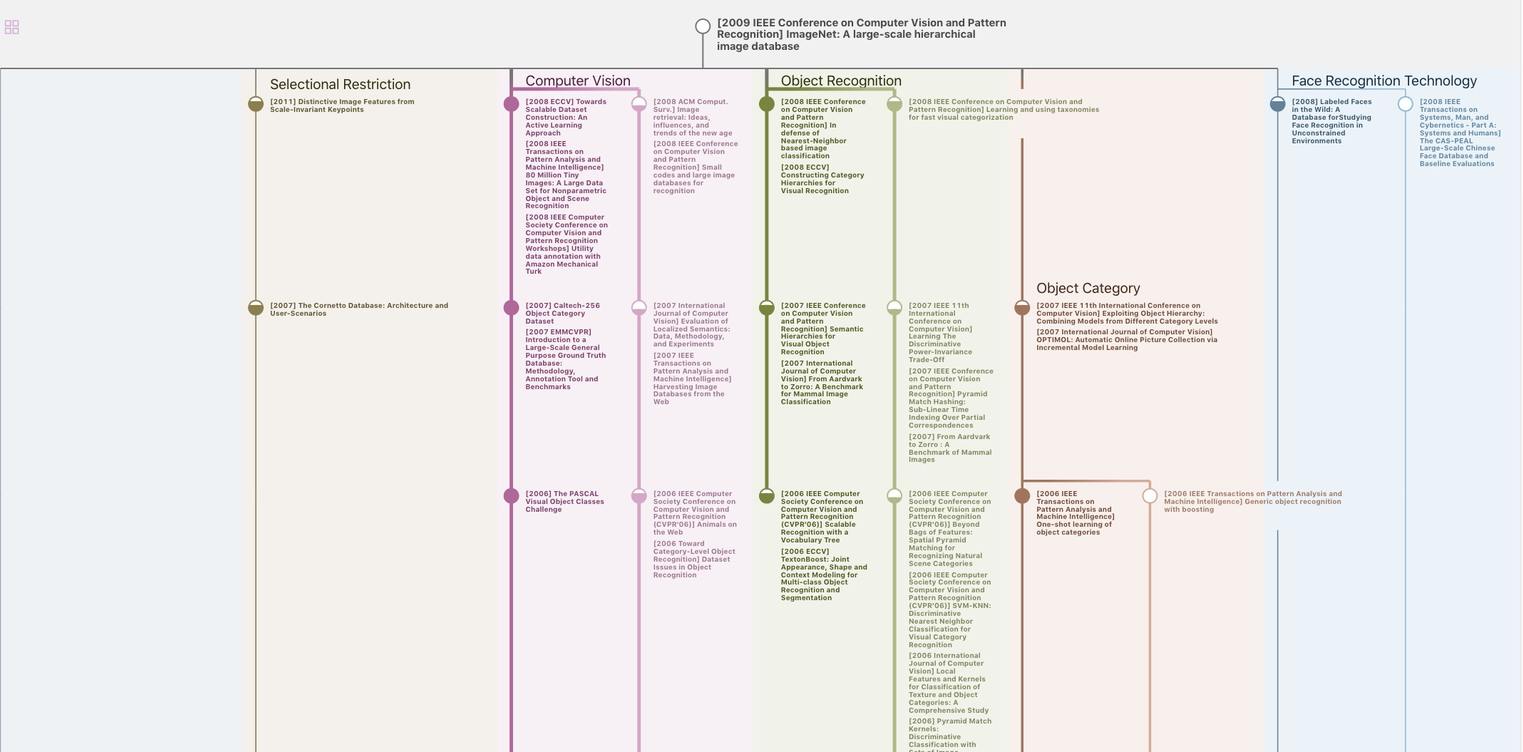
生成溯源树,研究论文发展脉络
Chat Paper
正在生成论文摘要