Identifying Exoplanets in TESS Data by Deep Learning
Smart innovation, systems and technologies(2023)
摘要
Deep Learning algorithms are currently widely used in astronomy for providing accurate predictions in classification problems, and more specifically to the search for planets outside the Solar System, called “exoplanets” (e.g., [16] and references therein). For instance, NASA’s Transiting Exoplanet Survey Satellite (TESS) has already observed 76% of the sky for over 3 years, producing millions of light curves but only $$\sim $$ 221 planets have been confirmed to date. That’s because the light curves need to be individually inspected by humans in order to validate the presence of planetary transits in them. To remove the human-interaction element that is slowing down the process, we developed a Convolutional Neural Network (CNN) that can automatically identify the exoplanets from TESS light curves. Given the scarcity of labeled TESS light curves, we use Transfer Learning (TL) to train our model on a more robust and larger training set, i.e., the $$\sim $$ 15,700 labeled light curves from the NASA’s Kepler survey. We then used the learned representation as basic knowledge and fine-tuned the CNN upper layers by making them task dependent on the TESS labeled samples. Moreover, we use the dropout and adaptive reparameterization techniques to prevent our model from overfitting and approximately combining exponentially many different neural network architectures efficiently. We show that the use of TL in parallel with regularization techniques significantly improves the performance of the net, which achieves a precision of 87% and a recall of 81%, thus outperforming similar algorithms already available in the literature (see, e.g., [15, 21]).
更多查看译文
关键词
exoplanets,tess data,deep learning
AI 理解论文
溯源树
样例
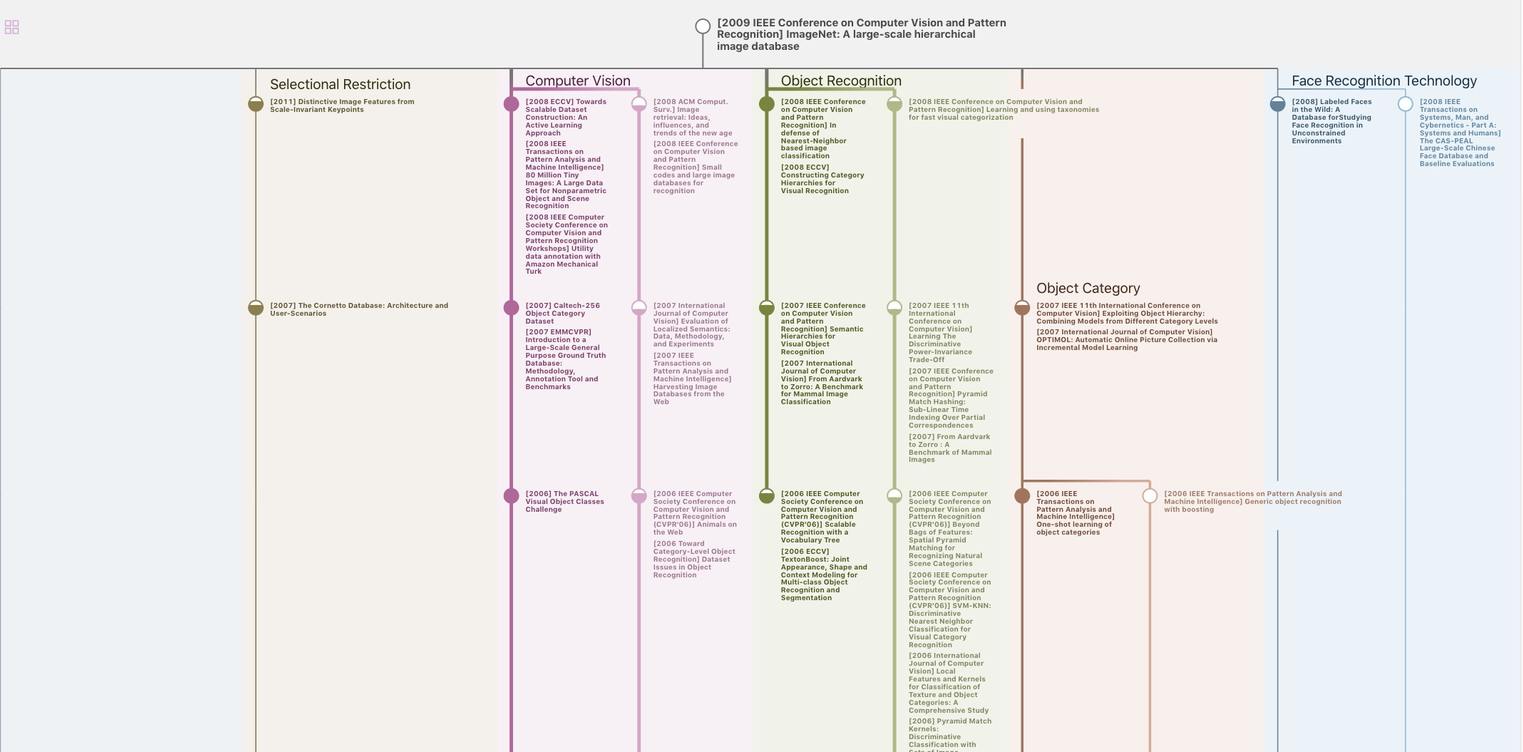
生成溯源树,研究论文发展脉络
Chat Paper
正在生成论文摘要