CLIPREC: Graph-based Domain Adaptive Network for Zero-Shot Referring Expression Comprehension
IEEE TRANSACTIONS ON MULTIMEDIA(2024)
关键词
Referring expression comprehension,domain adaptive network,zero-shot learning,CLIP
AI 理解论文
溯源树
样例
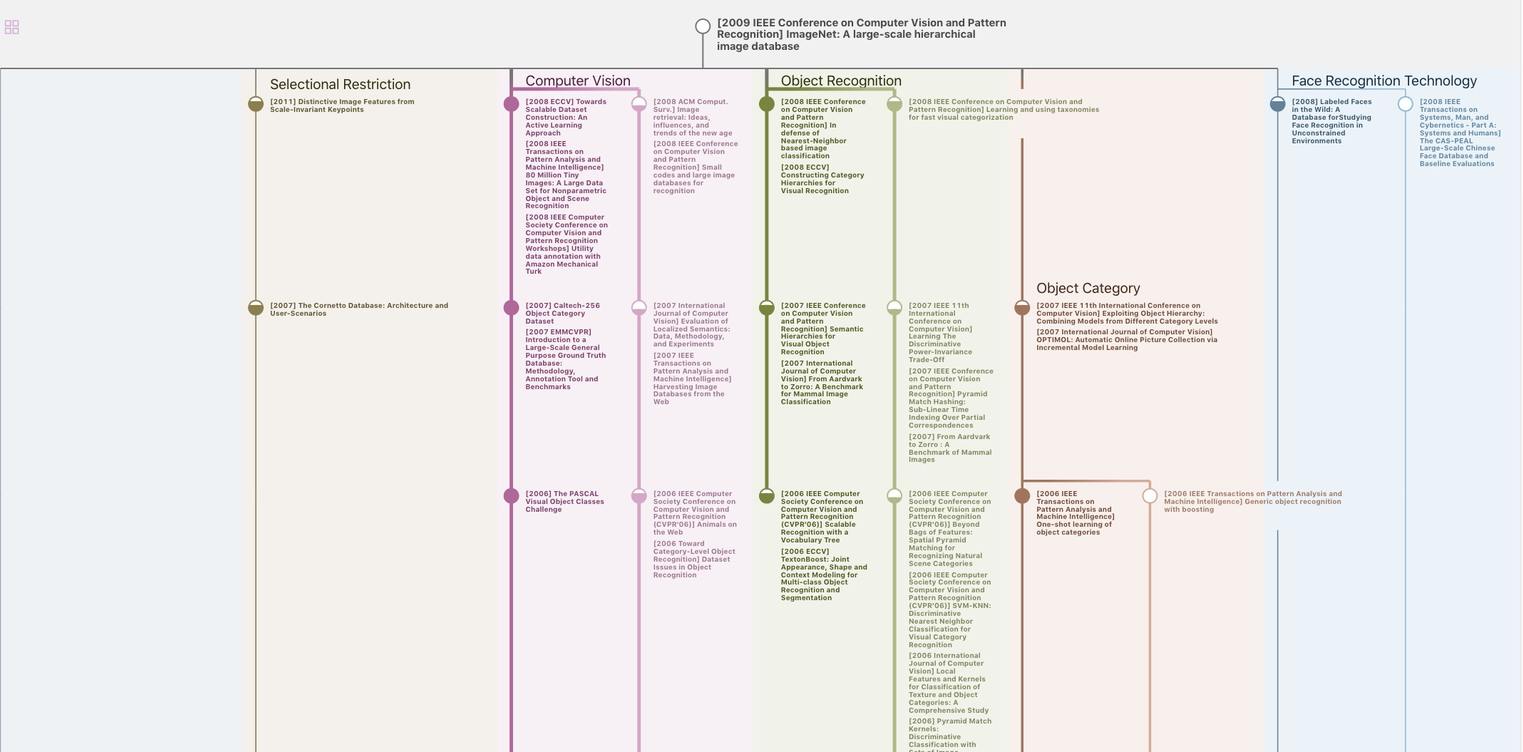
生成溯源树,研究论文发展脉络
Chat Paper
正在生成论文摘要