Privacy-Preserving Collaborative Learning: A Scheme Providing Heterogeneous Protection
IEEE INTERNET OF THINGS JOURNAL(2024)
摘要
With the widespread application of collaborative learning (CL) technology in mobile-crowdsourcing-related scenarios, special attention should be paid to the privacy disclosure problem therein. Many pioneer noise-perturbation-based methods, particularly the differentially private ones, provide only homogeneous protection, which is insufficient for the heterogeneous protection requirements of many practical CL cases. In this article, we propose a privacy-aware mechanism that uses appropriate Gaussian noises to obfuscate the local and aggregated models. The noise variance is determined based on clients' different privacy requirements. By zero-concentrated differential privacy, we analyze clients' privacy-preserving degrees (PPDs) in the uplink and downlink channels. The obtained PPDs demonstrate that the information received by the aggregating server and the peer clients has distinct preservation effects, indicating that our scheme achieves the goal of heterogeneous protection. Moreover, we conduct a theoretical analysis of the performance of the global models aggregated during the iterative process. Finally, we validate the correctness of our theory with experimental results using a real-world data set.
更多查看译文
关键词
Collaborative learning (CL),crowdsourcing,heterogeneous privacy protection,Internet of Things (IoT)
AI 理解论文
溯源树
样例
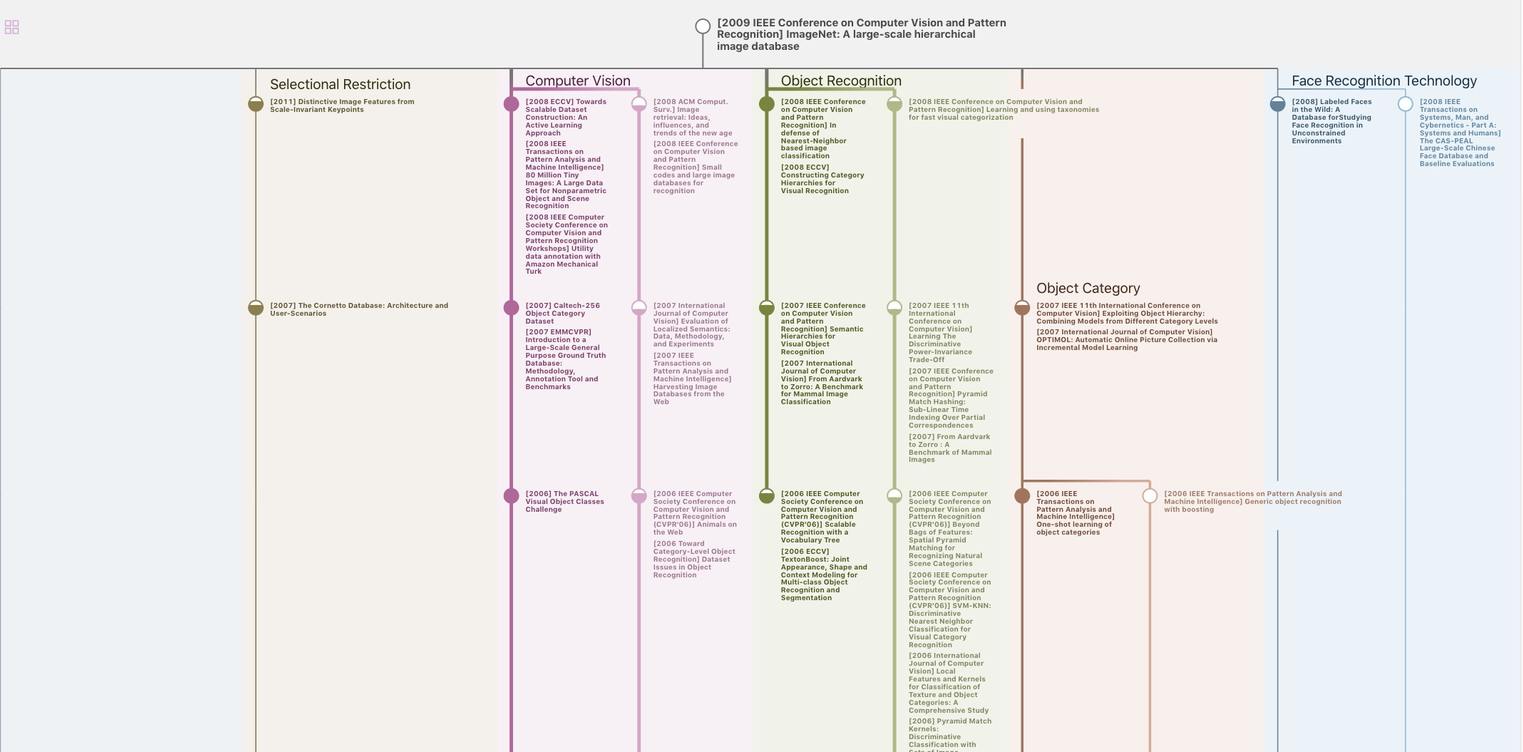
生成溯源树,研究论文发展脉络
Chat Paper
正在生成论文摘要