BPFL: Blockchain-Enabled Distributed Edge Cluster for Personalized Federated Learning
Lecture notes in electrical engineering(2023)
摘要
Due to the fact that the client's data distribution in federated learning (FL) is highly heterogeneous and leads to poor convergence, the concept of personalized federated learning (PFL) is on the rise. PFL aims to tackle the effect of non-IID data and statistical heterogeneity problems along with achieving rapid model convergence and personalized models. Moreover, in the context of clustering-based PFL, it allows a multi-model method with group-level client relationships to accomplish personalization. However, since the current method still relies on the centralized method, where the server orchestrates all processes, this paper introduces a blockchain-enabled distributed edge cluster for PFL (BPFL) that exploits the benefits of blockchain and edge computing to address these shortcomings. Blockchain can be employed to enhance client privacy and security by recording all transactions in immutable distributed ledger networks as well as improving efficient client selection and clustering. Furthermore, an edge computing system offers reliable storage and computation, where computational processing is locally performed in the edge infrastructure to be closer to clients. Thus, it also improves real-time services and low-latency communication of PFL.
更多查看译文
关键词
edge cluster,blockchain-enabled
AI 理解论文
溯源树
样例
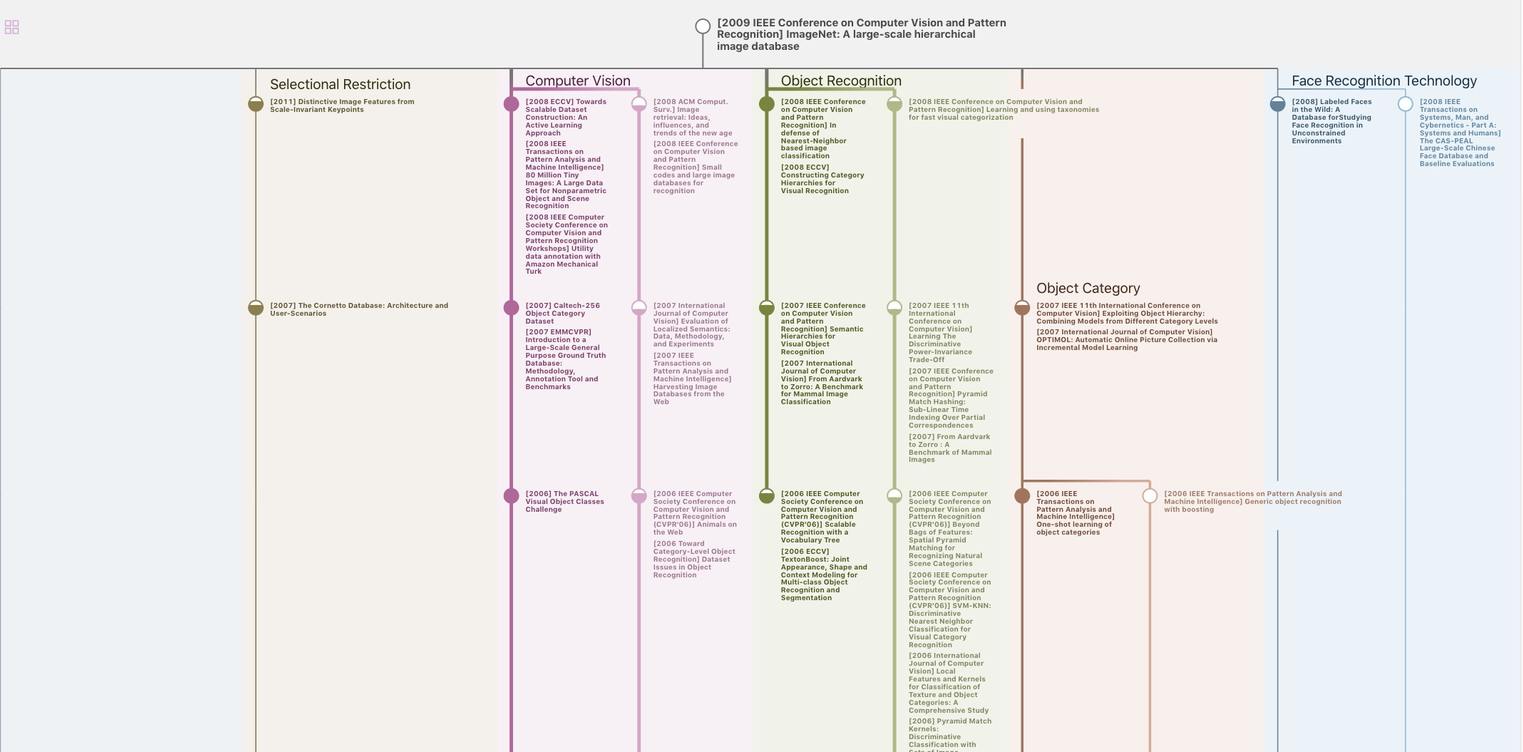
生成溯源树,研究论文发展脉络
Chat Paper
正在生成论文摘要