MINT: Multiplier-less INTeger Quantization for Energy Efficient Spiking Neural Networks
2024 29th Asia and South Pacific Design Automation Conference (ASP-DAC)(2024)
摘要
We propose Multiplier-less INTeger (MINT) quantization, a uniform quantization scheme that efficiently compresses weights and membrane potentials in spiking neural networks (SNNs). Unlike previous SNN quantization methods, MINT quantizes memory-intensive membrane potentials to an extremely low precision (2-bit), significantly reducing the memory footprint. MINT also shares the quantization scaling factor between weights and membrane potentials, eliminating the need for multipliers required in conventional uniform quantization. Experimental results show that our method matches the accuracy of full-precision models and other state-of-the-art SNN quantization techniques while surpassing them in memory footprint reduction and hardware cost efficiency at deployment. For example, 2-bit MINT VGG-16 achieves 90.6% accuracy on CIFAR-10, with roughly 93.8% reduction in memory footprint from the full-precision model and 90% reduction in computation energy compared to vanilla uniform quantization at deployment.
1
1
Code is available at https://github.com/Intelligent-Computing-Lab-Yale/MINT-Quantization
更多查看译文
关键词
Spiking Neural Networks,Integer Quantization,Scaling Factor,Quantification Method,Low Precision,Memory Footprint,Quantization Scheme,Computation Energy,Footprint Reduction,Uniform Quantization,Artificial Neural Network,Energy Conservation,Convolution Operation,Accuracy Drop,Pre-defined Threshold,Backward Propagation,Spike-timing-dependent Plasticity,32-bit Floating-point,Output Spike,Large Batch Size,Input Spike,Hardware Overhead,Total Footprint
AI 理解论文
溯源树
样例
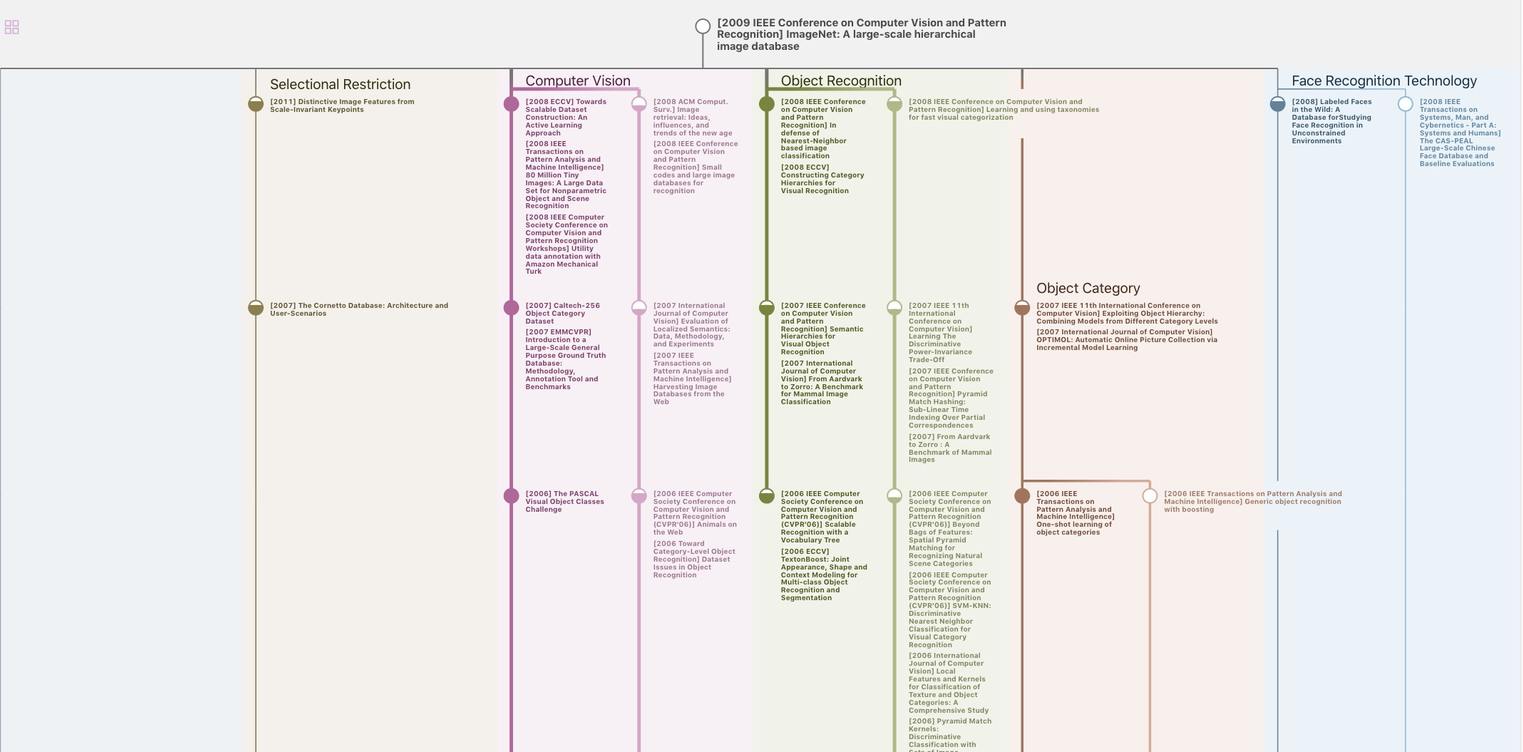
生成溯源树,研究论文发展脉络
Chat Paper
正在生成论文摘要