Abstract 4291: Deconvolution of cell type composition in FFPE tissue: Application to benign breast disease
Cancer Research(2023)
Abstract
Abstract Purpose: Benign breast disease (BBD) is viewed as a non-obligate precursor stage in the development of breast cancer (BC). Transcriptome profiling using RNA sequencing (RNA-seq) of bulk formalin-fixed paraffin-embedded (FFPE) tissue blocks has been routinely used in BC research. However, RNA-seq of heterogeneous bulk samples represents an averaged expression profile across all cell mixtures. Downstream analyses, such as biomarker discovery, are likely confounded by differences in cell proportions. There is a lack of research in optimizing strategies for cell type deconvolution especially for FFPE samples. We evaluated and optimized strategies for cell type deconvolution using existing single cell RNA-seq (scRNA-seq) and single nucleus RNA-seq (snRNA-seq) breast tissue references, and applied these to a cohort of BBD FFPE samples to assess risk of subsequent BC. Methods: 7 scRNA-seq and 3 snRNA-seq public samples from women’s breast tissue were normalized using SCTransform, then integrated using Harmony to form a complete reference set. Bioinformatics evaluations examined the performance of existing statistical and deep learning-based deconvolution methods. Computational simulations were conducted to evaluate those methods on three scenarios: 1) baseline; 2) incomplete reference set; 3) FFPE artifacts. FFPE artifacts were simulated based on a generalized additive model using 7 in-house paired FFPE-fresh frozen samples. Finally, we applied the best performing method to our BBD cohort of 62 samples. Differential expression analysis adjusting for cell proportions was performed to identify transcriptomic aberrations in BBD cases. Results: snRNA-seq was more sensitive in capturing adipocytes, while scRNA-seq was more sensitive in detecting lymphocytes in breast tissue. The median number of detectable genes per cell was higher for scRNA-seq than snRNA-seq before integration (1264 vs 558). Marker gene-based regression methods (e.g. CIBERSORT) displayed higher variance and lower bias compared to methods directly using scRNA-seq data (e.g. SCDC). Incomplete reference data led to increased bias and little effect on variance. FFPE artifacts had a higher impact on performance compared to incomplete reference data. Overall, Scaden, a deep learning-based method, was the top performing method across all simulation scenarios and was especially robust to FFPE artifacts. 59 differential expressed genes (DEGs) were identified with fold change > 1.5 and p value < 0.01. DEGs involved in BC, e.g. S100A8, WNT4 and HIST1H3H, would be left out if cell proportions were not properly adjusted. Conclusions: We constructed the first complete single cell reference data for women’s breast tissue and benchmarked existing cell type deconvolution methods. Compared to analyses not adjusting for cell proportions, we observed substantial differences in perturbed genes and pathways associated with BC risk for BBD patients. Citation Format: Yuanhang Liu, Chen Wang, Stacey J. Winham, Aditya Bhagwate, Robert A. Vierkant, Julie M. Cunningham, Bryan M. McCauley, William A. Jons, Derek C. Radisky, Amy C. Degnim. Deconvolution of cell type composition in FFPE tissue: Application to benign breast disease. [abstract]. In: Proceedings of the American Association for Cancer Research Annual Meeting 2023; Part 1 (Regular and Invited Abstracts); 2023 Apr 14-19; Orlando, FL. Philadelphia (PA): AACR; Cancer Res 2023;83(7_Suppl):Abstract nr 4291.
MoreTranslated text
Key words
ffpe tissue,cell type composition,deconvolution
AI Read Science
Must-Reading Tree
Example
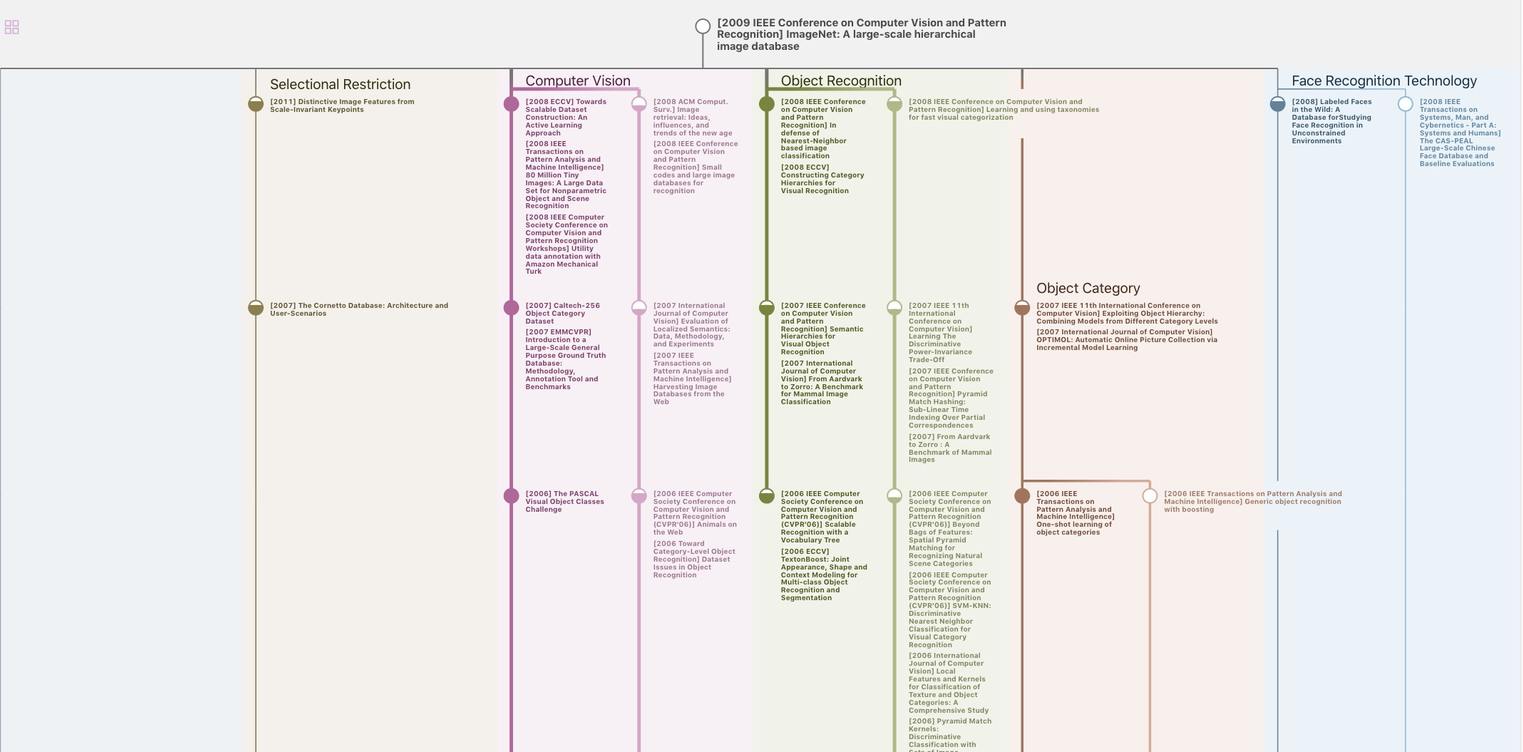
Generate MRT to find the research sequence of this paper
Chat Paper
Summary is being generated by the instructions you defined