Unsupervised Learning for Ultra-Reliable and Low-Latency Communications with Practical Channel Estimation
IEEE Transactions on Wireless Communications(2023)
摘要
In this paper, we optimize the resource allocation for channel estimation and data transmission and the packet size to maximize the resource utilization efficiency subject to the constraints of ultra-reliable low-latency communications (URLLC). With practical channel estimation, the packet error probability (PEP) does not have a closed-form expression. To solve the problem, we develop novel model-based and model-free unsupervised deep learning algorithms to train a deep neural network for resource allocation and data transmission. Two types of reliability constraints are considered over a wireless link: 1) average PEP constraint; 2) constraint on the probability that PEP is higher than a threshold. The simulation results show that the learning algorithms can guarantee both types of reliability constraints. Compared with a benchmark that maximizes the number of symbols for data transmission and uses the maximum ratio transmission precoding, the learning method with the codebook-based precoding achieves a lower average signal-to-interference-plus-noise ratio (SINR), but improves the resource utilization efficiency by three times. It is because the resource utilization efficiency of URLLC is dominated by the tail distribution of SINR, not the average SINR, and the SINR of the benchmark has a much longer tail distribution than the learning method.
更多查看译文
关键词
Ultra-reliable and low-latency communications,unsupervised deep learning,channel estimation,resource allocation
AI 理解论文
溯源树
样例
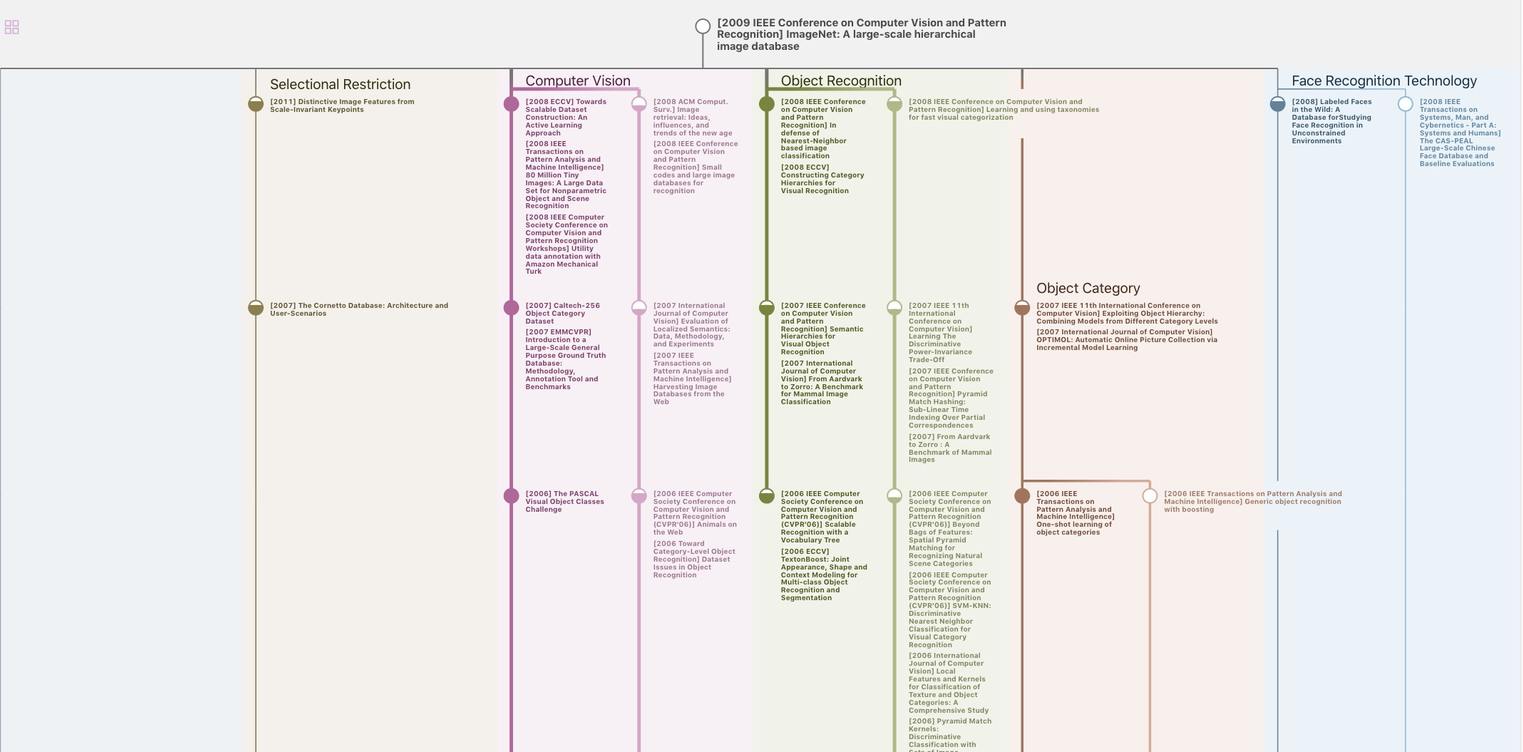
生成溯源树,研究论文发展脉络
Chat Paper
正在生成论文摘要