Multi-Label Feature Selection via Positive or Negative Correlation.
IEEE Trans. Emerg. Top. Comput. Intell.(2024)
摘要
Feature selection, a meaningful preprocessing technique in machine learning, plays a key role in multi-label learning to select more discriminative features. Recently, multi-label feature selection algorithms based on sparse regression model have received extensive attention. However, in the existing sparse regression model, feature-label correlations are obtained from a single viewpoint via a loss function. With the aim of learning richer information, in this article, the sparse regression model is integrated with a new feature-label correlation, which allows different viewpoints to be considered simultaneously. For this reason, a new correlation, called positive or negative correlation, is proposed. Furthermore, in many existing multi-label feature selection algorithms, each feature is associated with all of the labels, while in many scenarios, each feature is associated with only some labels. Aiming at the above two shortcomings, a new multi-label feature selection algorithm is proposed in four stages. First, a pseudo-label space is built and the global label correlation is embedded into this space. Second, the positive or negative correlation between features and labels is calculated. Third, a new objective function is established. The weight matrix and feature ranking results are obtained through the optimization process. Final, using the weight matrix as prior knowledge, the proposed algorithm exploits label-specific and label-group-specific features techniques to further improve performance. Extensive experiments on over than 20 multi-label datasets show its superior performance.
更多查看译文
关键词
Multi-label learning,feature selection,correlation,label-specific features
AI 理解论文
溯源树
样例
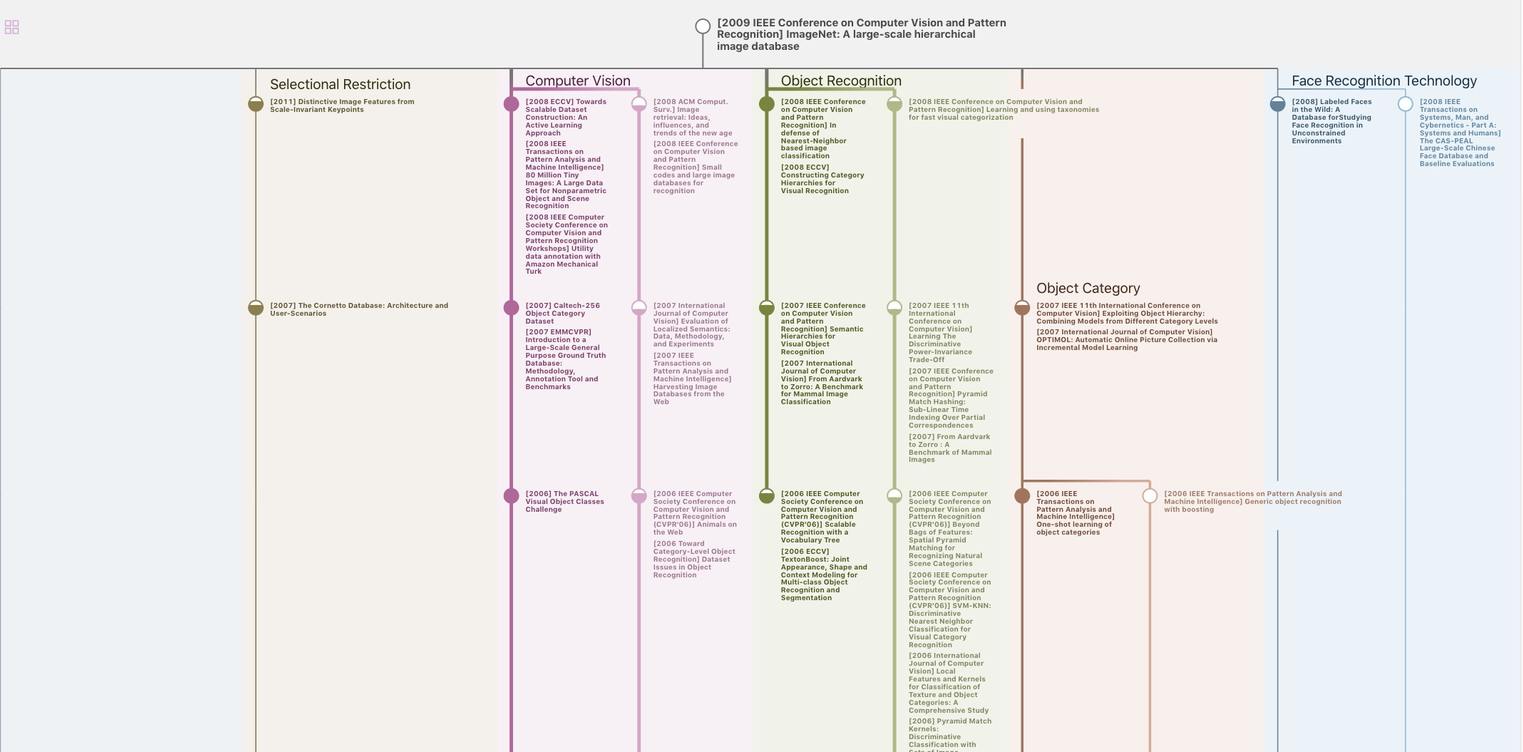
生成溯源树,研究论文发展脉络
Chat Paper
正在生成论文摘要