FOMO: A Fragment-based Objective Molecule Optimization Framework
Research Square (Research Square)(2023)
摘要
Abstract Drug discovery greatly relies on optimizing drug-like molecules to improve their properties. In this paper, we propose FOMO, a Fragment-based Objective Molecule Optimization Framework that addresses the challenge of structure collision in molecule optimization. Traditional graph-based methods only considered the types of atoms, bonds, and their connectivity, but they barely account for the varying importance of varying neighboring moieties. FOMO utilizes a multi-head Attention-based Graph model to incorporate the significance of distinct neighboring atoms and bonds during the process of feature aggregation. FOMO is used to modify moieties, by adding new fragments or removing moieties, aiming to improve specific drug-like properties of a given molecule. Our experiments demonstrate that FOMO, trained on a single dataset, generates molecules with superior properties while maintaining similarity to the original molecule. This is important to avoid immediate loss of pharmacological activity of lead compounds. In comparison to the previous state-of-the-art approaches, FOMO achieves the highest success rates in optimizing QED, DRD, PLogP, and multi-objective optimization tasks.
更多查看译文
关键词
objective molecule optimization framework,fragment-based
AI 理解论文
溯源树
样例
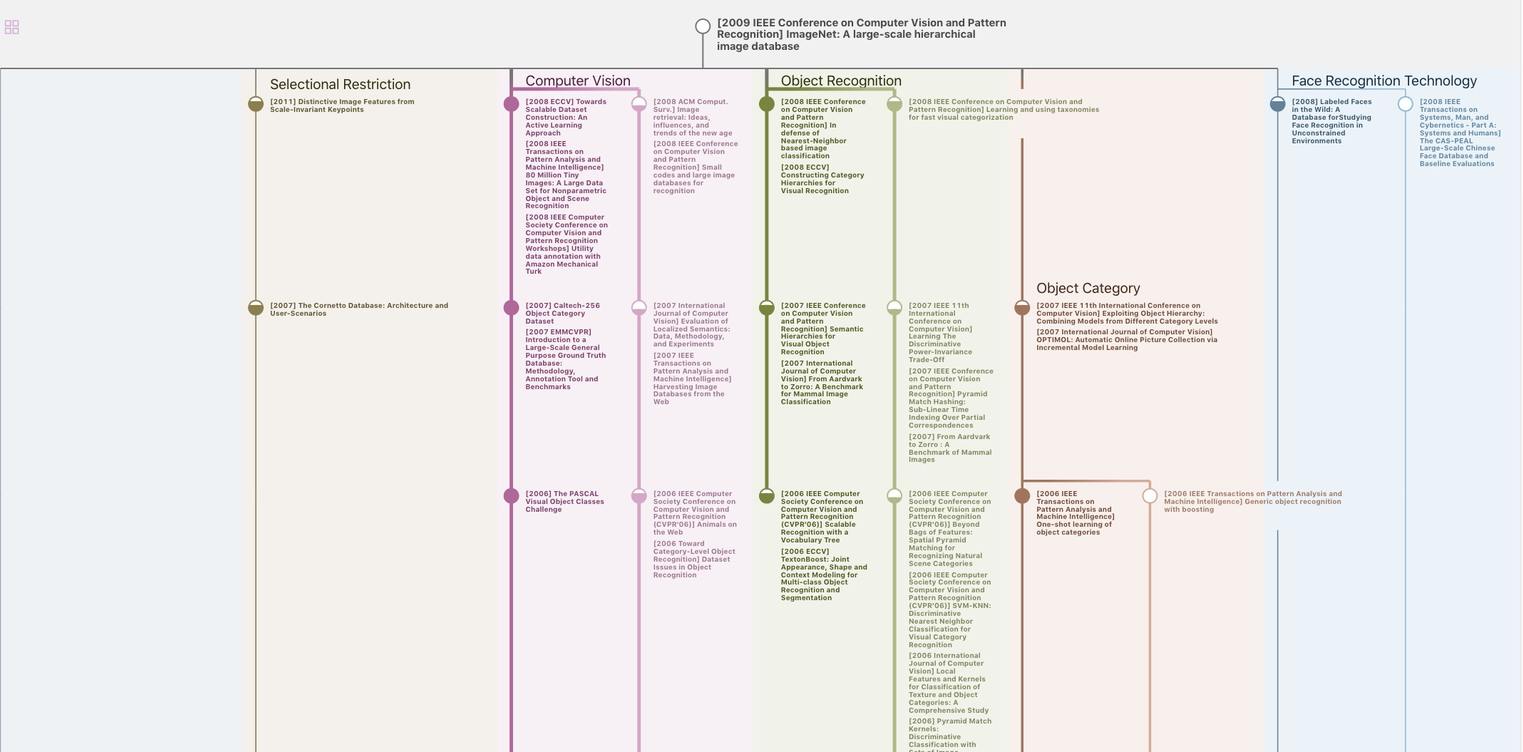
生成溯源树,研究论文发展脉络
Chat Paper
正在生成论文摘要