New Efficient Interpolation Techniques for Medical Images
Research Square (Research Square)(2023)
Abstract
Abstract This paper presents new efficient six image interpolation techniques that aim to obtain high resolution images from low resolution ones. The problem of dealing with image interpolation as an inverse problem was treated in wavelet domain through the first proposed approach. This approach estimates wavelet coefficients in high frequency sub-images using least squares algorithm. The approach reveals performance improvement in PSNR and MSE values compared to bilinear and least squares algorithm. A second approach based on using artificial neural network for modeling the maximum entropy interpolation algorithm was presented. Training the suggested network is achieved by adjusting its weights using learning algorithm to minimize the sum of squared error between target and actual output of output neurons. The proposed approach requires fewer computations than the conventional high-quality image interpolation methods with little reduction in PSNR compared to the maximum entropy algorithm. A third algorithm which combines new contourlet transform and edge-based interpolation algorithm was proposed to improve object boundaries regularity which could be achieved by treating each successive approximation of the high-resolution image as a noisy approximation, hence enforcing the contourlet coefficients to have a sparsity constraint. The proposed algorithm gives an improvement of 2 dB in PSNR compared to IIGR algorithm and achieves the best MSE and correlation coefficient. The fourth approach presents an efficient method to get HR images from the fusion of Magnetic resonance and computed tomography images by adopting least-squares strategy to iteratively obtain wavelet sub-bands of the target HR image. The proposed approach was found to achieve good results especially when the curvelet transform is used to merge the CT and MR images. The fifth approach presents neural modeling of the maximum entropy image interpolation algorithm based on both curvelet and wavelet fusion. Through this approach the fusion process is performed while edges with a curved nature are preserved. Results showed that applying curvelet transform in the fusion of CT and MR images is better than DWT fusion and that interpolating the fused image by neural modeling of maximum entropy image interpolation algorithm is superior to interpolating the original MR and CT images. The sixth approach presents a new image scale-up, super-resolution, approach based on image fusion principle. MR and CT images were scaled-up using sparse-representation modeling with dictionary learning algorithms of Yang et. al. and Michael et. al.. The images were fused by discrete wavelet and curvelet transforms, then scaled-up by the same algorithms. Simulation results showed that scaling-up the fused MR and CT images, either by wavelet or curvelet fusion techniques, gives higher PSNR values than scaling-up the MR and CT image separately.
MoreTranslated text
AI Read Science
Must-Reading Tree
Example
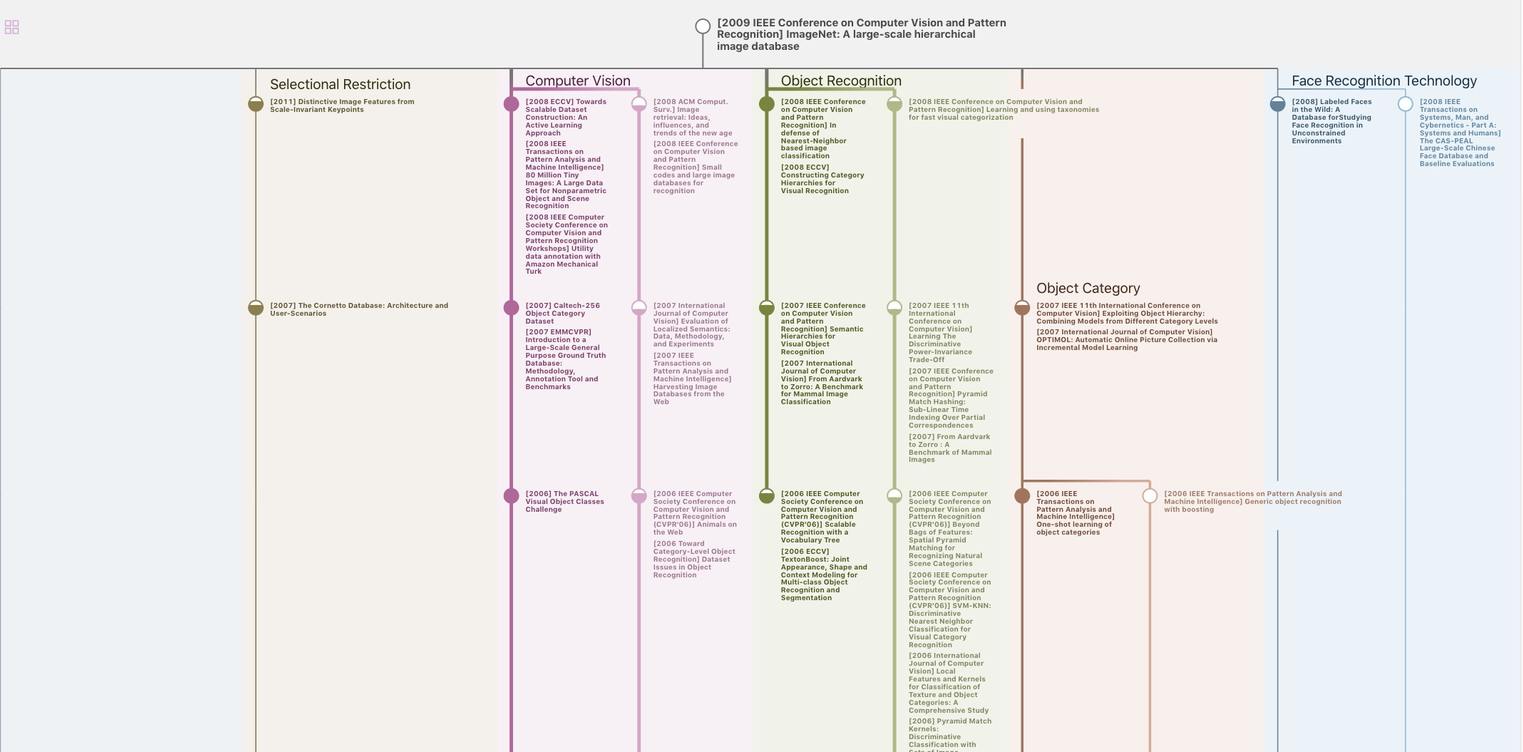
Generate MRT to find the research sequence of this paper
Chat Paper
Summary is being generated by the instructions you defined