What did the T cell see? A deep-learning model of CD8+ T cell activation reveals sharp antigen discrimination at the single cell level
Journal of Immunology(2023)
摘要
Abstract Immunotherapies such as checkpoint blockade antibodies to block T cell exhaustion have been successful in several cancers such as non-small cell lung cancer and melanoma, but limited in others (e.g., pancreatic or prostate carcinomas) owing to differences in tumor antigenicity. Therefore, quantifying tumor antigenicity is critical for successful immunotherapies. Our lab has shown that antigenicity can be encoded in a single parameter derived from bulk cytokine dynamics in ex vivo co-cultures between antigen presenting cells (APCs) and T cells. Here we built a model that can capture the antigenicity seen by individual cells. Using a custom robotic platform, we generated high-throughput kinetics of T cell activation in co-culture with APCs by analyzing cells at various timepoints across a large set of activation conditions. We performed spectral flow cytometry to measure the expression of up to 30 surface markers and intracellular signals per cell. To analyze our content-rich datasets, we designed a machine learning-based model that can classify the antigen seen by an individual cell using expression values from flow cytometry. The model performs well not only at classifying T cells (ROC-AUC > 0.91), but also APCs (ROC-AUC > 0.88), suggesting that each individual leukocyte may register the quality of antigen being presented. Blocking cytokine signaling disrupted this antigen classification. Our study demonstrates that every individual lymphocyte can bridge local and global response to achieve high discriminatory power of antigens.
更多查看译文
关键词
sharp antigen discrimination,single cell level,deep-learning deep-learning
AI 理解论文
溯源树
样例
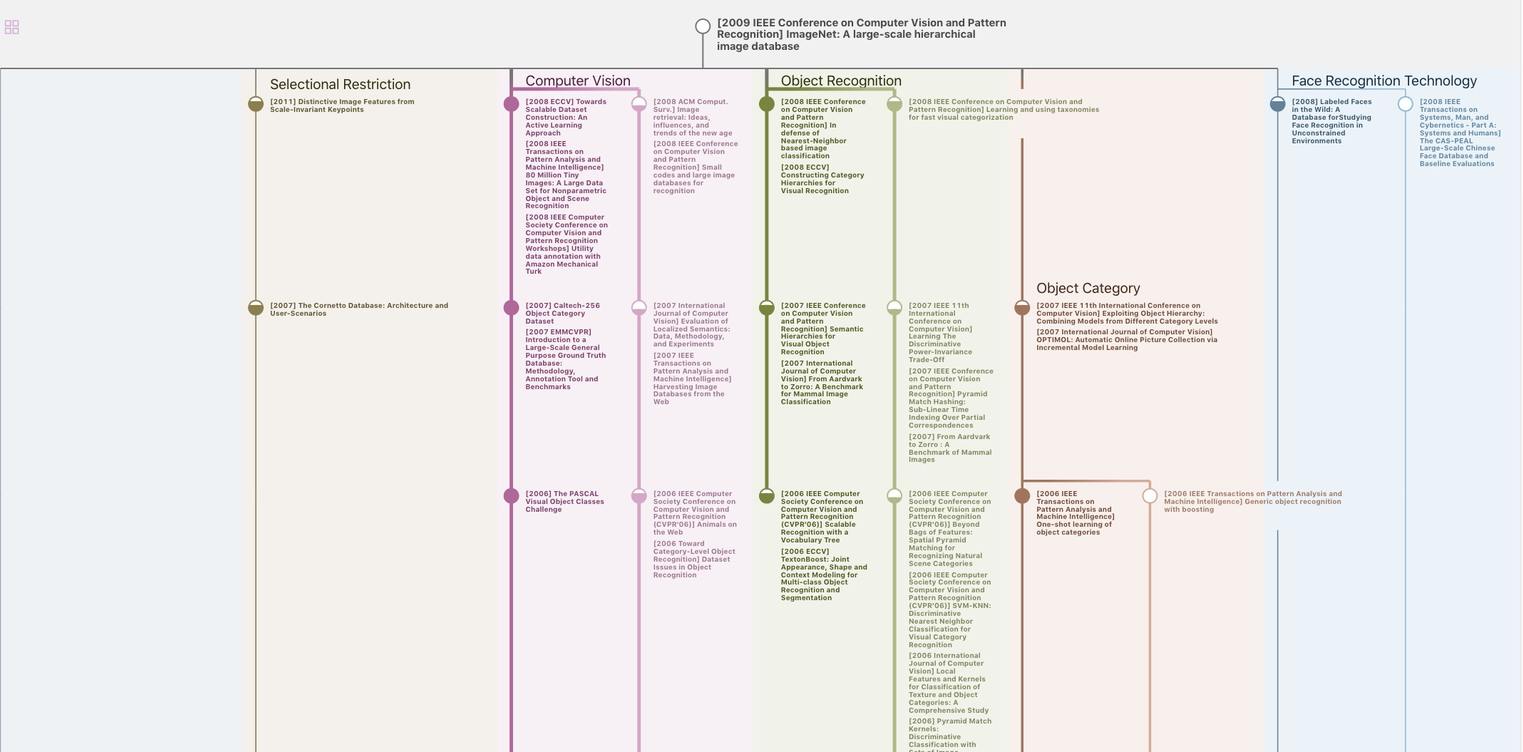
生成溯源树,研究论文发展脉络
Chat Paper
正在生成论文摘要