Permeability Prediction from Seismic Data Using the Data-Model-Driven Approach
Springer series in geomechanics and geoengineering(2023)
Abstract
Rock petrophysics experiments and digital core technology have found that permeability is influenced by pore structure such as pore size and morphology, but the two factors usually may not be taken into account together in permeability prediction from seismic data. To better predict permeability, we attempt to derive a permeability model including pore size and morphology parameters on the basis of the Kozeny model. The modified Kozeny model explains that permeability is a function of porosity, aspect ratio, minor semi-axis, and tortuosity. We also employ the random forest regression algorithm, combined with the model derived above, to propose a data-model-driven approach to predict permeability. Specifically, we first calculate the aspect ratio by P-waves velocity and porosity based on the DEM model. Then we fit a random forest regression model to predict the values of minor semi-axis and tortuosity, using aspect ratio, elastic parameters, density, and porosity. Finally, we obtain the predicted permeability values referring to the expression in the modified Kozeny model. Applications on logging and seismic data from the gas field show that the method is reliable and of high precision and the error is controlled within half an order of magnitude.
MoreTranslated text
Key words
seismic data,permeability,prediction,data-model-driven
AI Read Science
Must-Reading Tree
Example
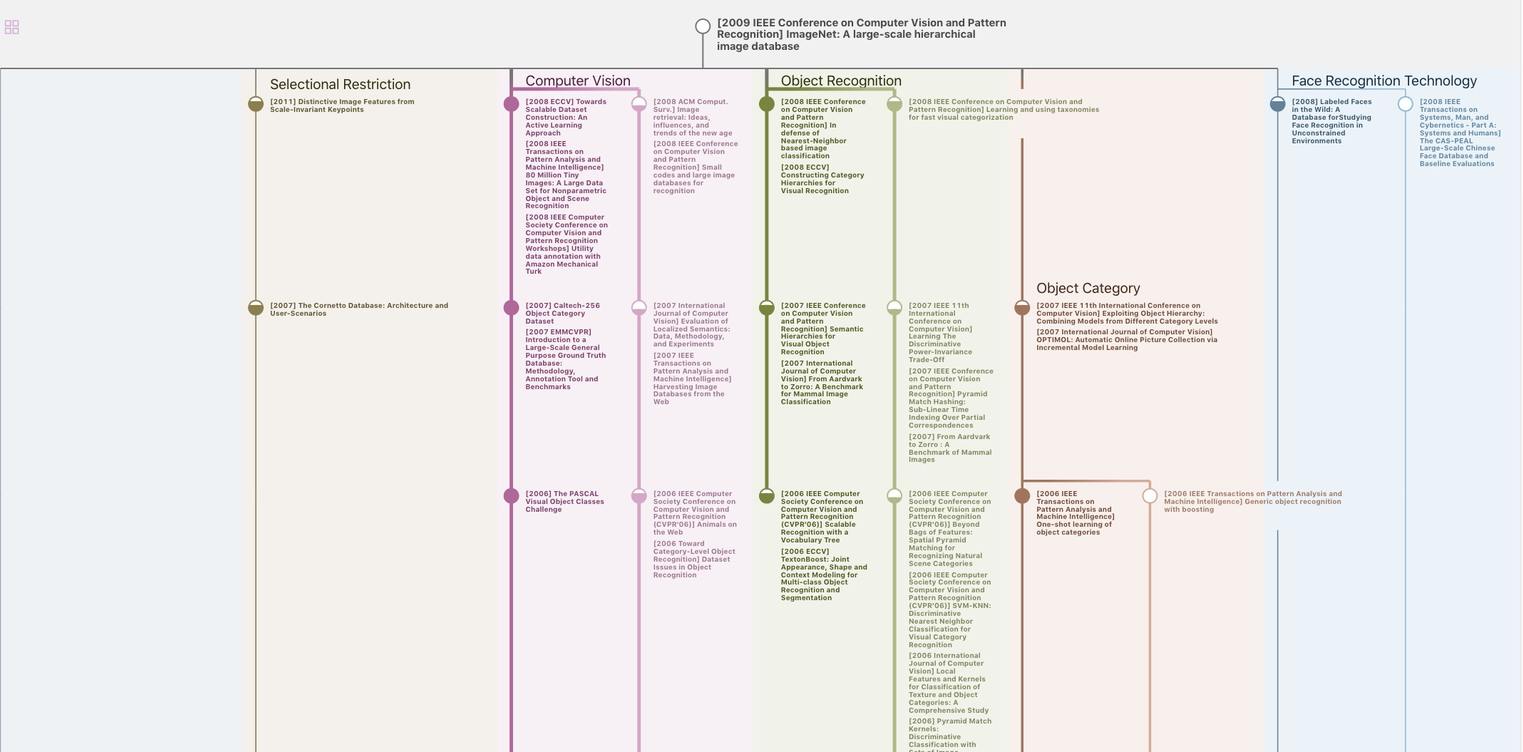
Generate MRT to find the research sequence of this paper
Chat Paper
Summary is being generated by the instructions you defined