Integrating hybrid modelling and transfer learning for new bioprocess predictive modelling
Computer-aided chemical engineering(2023)
Abstract
Hybrid modelling combines data-driven and mechanistic modelling, providing a cost-effective solution to modelling complex (bio)chemical processes when the underlying mechanisms are not fully understood. While more data-efficient than pure data-driven methods, sufficient experimental data must still be collected through time-consuming experiments for hybrid model construction when applied to simulate a newly investigated process. To address this challenge and accelerate design of new systems, transfer learning has been recently implemented to transfer knowledge from a well-understood source domain to a less-studied target domain. Therefore, in this study, hybrid modelling and transfer learning are integrated for the first time to predict a novel dynamic process for microalgal lutein synthesis through photo-production. Specifically, a hybrid model was built to extract primary knowledge about a well-known lutein production strain, Desmodesmus sp., for which considerable process data and a high-fidelity kinetic model are available from the literature. Then transfer learning was used to update the hybrid model using limited process data for a recently isolated microalgal strain, Chlorella sorokiniana. Once built, the hybrid transfer model was tested by predicting biomass growth and lutein production of the new strain under various operating conditions. Furthermore, bootstrapping was adopted to estimate and propagate the uncertainty of the hybrid transfer models when predicting each batch process. The performance and efficiency of the hybrid transfer learning model were thoroughly demonstrated through experimental verification. It was found that hybrid transfer learning more efficiently modularised primary knowledge than previous pure data-driven transfer learning techniques, enabling more selective updates of source-specific knowledge to preserve generalisable knowledge. This study, therefore, provides a novel contribution to the predictive modelling and knowledge transfer of complex bioprocesses.
MoreTranslated text
Key words
new bioprocess predictive modelling,hybrid modelling,transfer learning
AI Read Science
Must-Reading Tree
Example
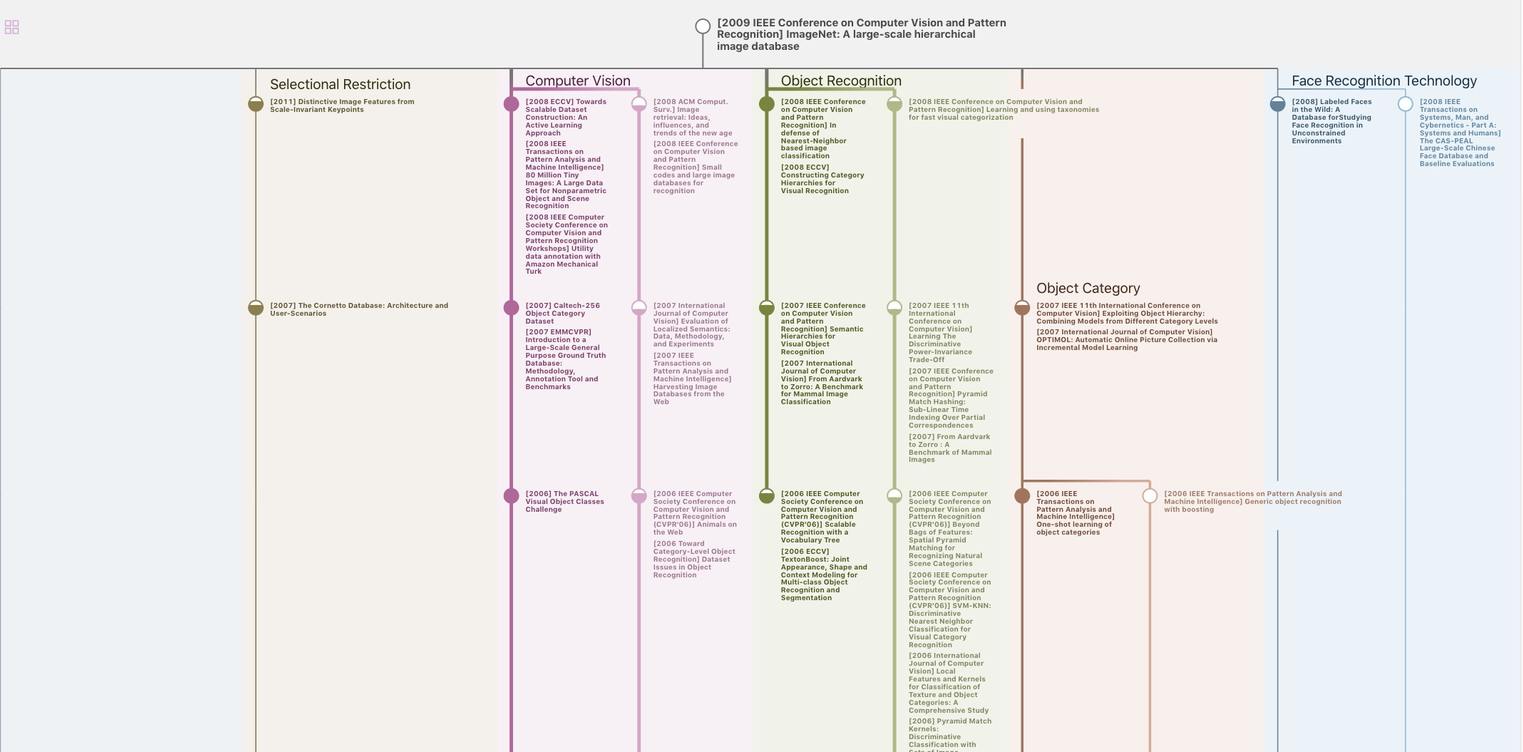
Generate MRT to find the research sequence of this paper
Chat Paper
Summary is being generated by the instructions you defined