Deep Neighborhood Structure-Preserving Hashing for Large-Scale Image Retrieval
IEEE TRANSACTIONS ON MULTIMEDIA(2024)
摘要
Deep hashing integrates the advantages of deep learning and hashing technology, and has become the mainstream of the large-scale image retrieval field. However, when training the deep hashing models, most of the existing approaches regard the similarity margin of image pairs as a constant. Once similarity distance exceeds the fixed margin, the network will not learn anything, which easily results in model collapses. In this paper, we address this dilemma with a novel unified deep hashing framework, termed Deep Neighborhood Structure-preserving Hashing (DNSH), to generate the similarity-preserving and discriminative hash codes. Specifically, by extracting the discriminative object characteristics with large variances, we design an adaptive margin quadruplet loss to further explore the underlying similarity relationship between image pairs, reflecting the correct semantic structure among its neighbors. Based on the quadruple form, we develop a quadruple regularization to decrease quantization errors between binary-like embedding and hashing codes. Furthermore, through learning bit balance and bit independent terms jointly, we present the binary code constraint loss to alleviate redundancy in different bits. Extensive evaluations on four popular benchmark datasets demonstrate that our proposed deep hashing framework achieves an excellent performance than the comparison methods.
更多查看译文
关键词
Binary codes,Semantics,Quantization (signal),Feature extraction,Training,Dogs,Convolutional neural networks,Adaptive margin,deep hashing,image retrieval,large variances,neighborhood structure-preserving,quadruplet loss,quadruple regularization
AI 理解论文
溯源树
样例
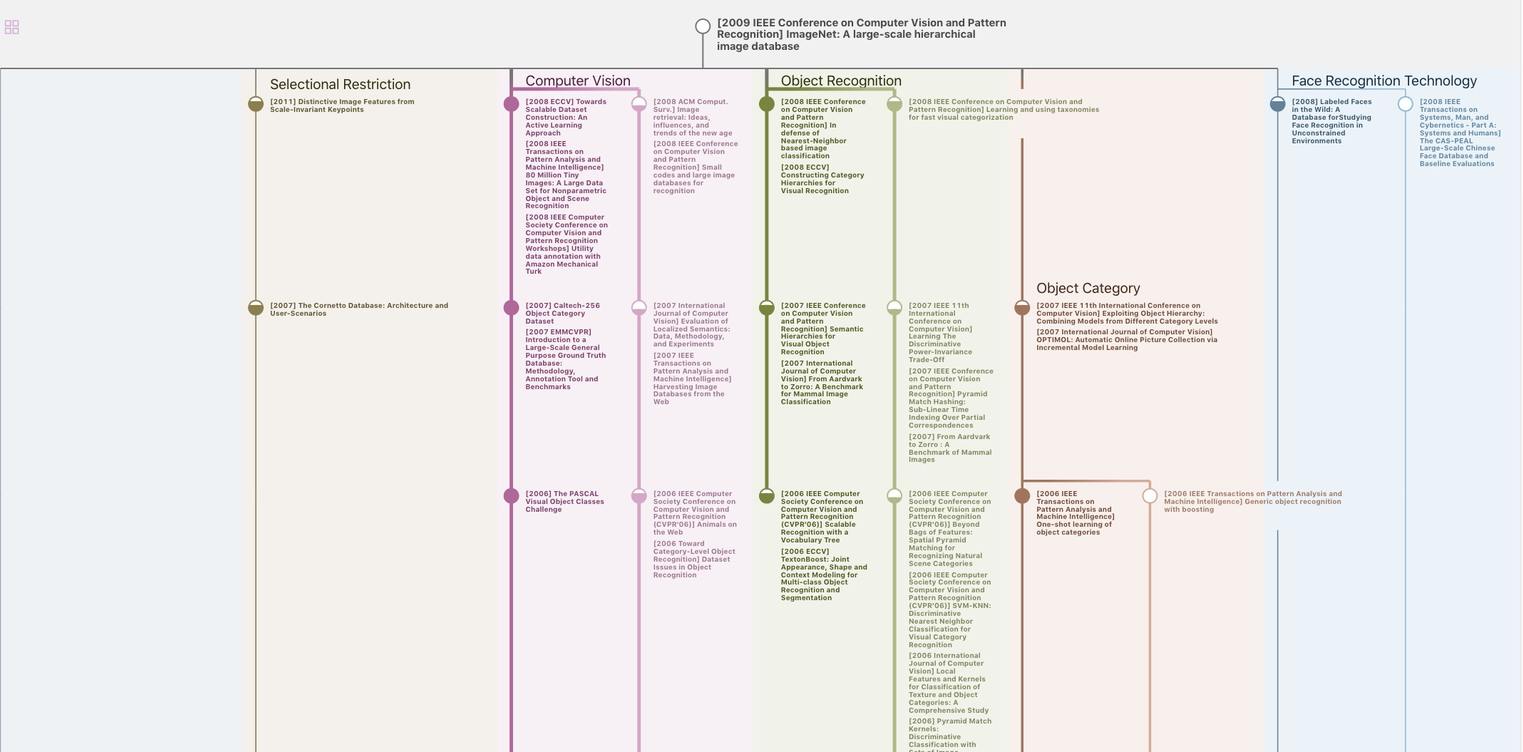
生成溯源树,研究论文发展脉络
Chat Paper
正在生成论文摘要