Panoptic-Level Image-to-Image Translation for Object Recognition and Visual Odometry Enhancement
IEEE TRANSACTIONS ON CIRCUITS AND SYSTEMS FOR VIDEO TECHNOLOGY(2024)
摘要
Image-to-image translation methods have progressed from only considering the image-level information to integrating the global- and instance-level information. However, only the foreground instances are refined, and the background semantics are taken as an entire feature, which causes a substantial loss of the semantic information in the translation. Additionally, the insufficient quality of the translated semantic regions also leads to an unsatisfactory performance of the object recognition or visual odometry tasks in which the translated images/videos are further used. In this paper, we propose a novel generative adversarial network for panoptic-level image-to-image translation (PanopticGAN). The proposed method has three advantages: 1) the extracted panoptic perception (i.e., the foreground instances and background semantic regions) as content codes are aligned with the sampled panoptic style codes, which considers the panoptic-level information to avoid the semantic information loss, and the latent space of each object has a rich fusion of content and style codes to generate the higher-fidelity results; 2) a feature masking module is proposed to extract the representations within each object contour by masks for sharpening the object boundaries; 3) the improved fidelity of the translated semantic regions further contributes to enhancing the performance of the object recognition or visual odometry tasks that the translated images/videos are used in. In this paper, we also annotate a compact panoptic segmentation dataset for the thermal-to-color translation task. Extensive experiments are conducted to demonstrate the effectiveness of our PanopticGAN over the latest methods.
更多查看译文
关键词
Image-to-image translation,panoptic-level,feature masking,generative adversarial networks,image/video enhancement
AI 理解论文
溯源树
样例
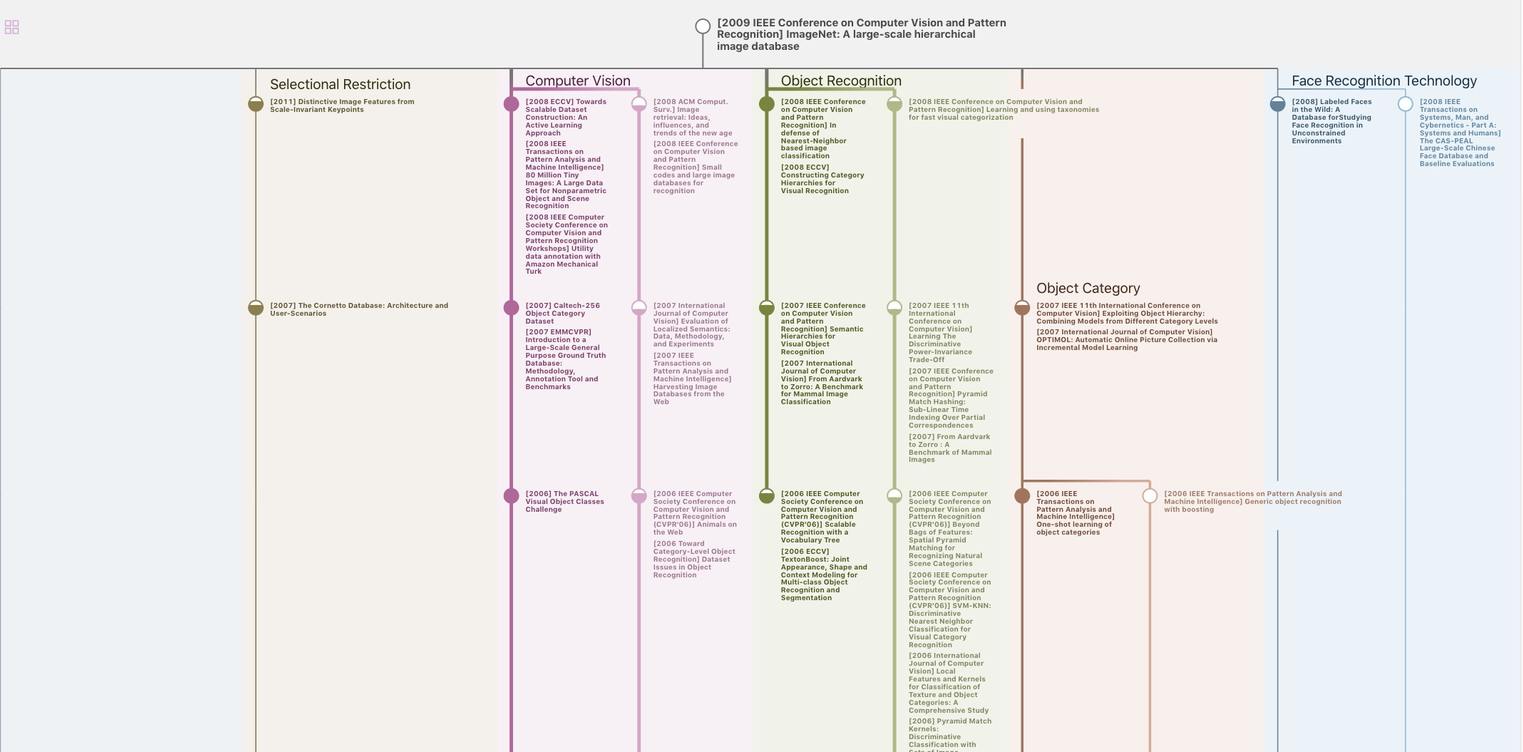
生成溯源树,研究论文发展脉络
Chat Paper
正在生成论文摘要