Reachability of Koopman Linearized Systems Using Explicit Kernel Approximation and Polynomial Zonotope Refinement
Research Square (Research Square)(2023)
Abstract
Abstract Koopman operator linearization approximates nonlinear systems of differential equations with higher-dimensional linear systems. For formal verification using reachability analysis, this is an attractive conversion, as highly scalable methods exist to compute reachable sets for linear systems. However, two main challenges are present with this approach, both of which are addressed in this work. First, the approximation must be sufficiently accurate for the result to be meaningful, which is controlled by the choice of observable functions during Koopman operator linearization. We formulate a more systematic approach for observables generation for black box systems using a two explicit kernel approximation methods: random Fourier features and Gaussian quadrature features. We also utilize a data-dependent reweighting to reduce the number of features by roughly 2-3 times, consequently speeding up reachability analysis while maintaining comparable accuracy. Second, although the dynamics of the higher-dimensional system is linear, simple convex initial sets in the original space can become complex non-convex initial sets in the linear system due to the nonlinear observable functions. We overcome this using a combination of Taylor model arithmetic and polynomial zonotope refinement. Compared with prior work, the result is more efficient, more systematic, and more accurate.
MoreTranslated text
Key words
koopman linearized systems,polynomial zonotope refinement,explicit kernel approximation
AI Read Science
Must-Reading Tree
Example
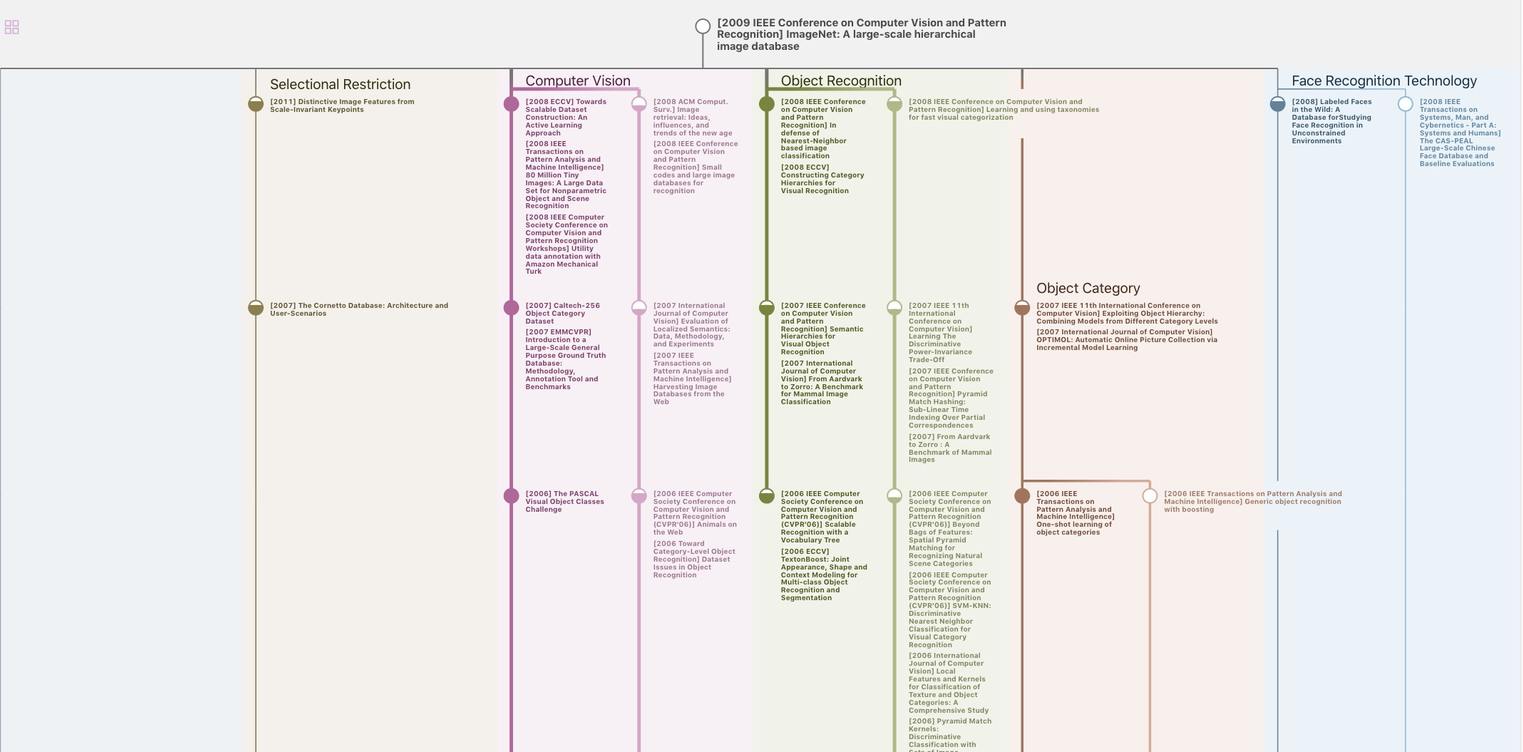
Generate MRT to find the research sequence of this paper
Chat Paper
Summary is being generated by the instructions you defined