Automated detection of lacunar infarcts from MRI images
Alzheimer's & Dementia(2023)
Abstract
Background When MRI images of patients with memory problems are assessed, the presence of lacunar infarcts is evaluated. Lacunar infarcts, fluid‐filled cavities of 3‐15 mm in diameter, are one possible result of cerebral small vessel disease and therefore relevant in dementia diagnostics. In this study, we propose an automated tool for detecting lacunar infarcts on MRI images. Method We adapted our previous approach [1] in three ways: 1) post‐processing steps were added to the convolutional neural network segmentation pipeline, e.g., to exclude enlarged perivascular spaces and segmentations located inside sulci or ventricles, 2) memory clinic data from the Amsterdam Dementia Cohort and PredictND cohort were included in addition to the LADIS cohort data, and 3) the ground truth was defined by three experienced neuroradiologists (A, B, C) instead of one rater. T1 and T2‐FLAIR images were available for all cases and T2 for a subset in visual rating. The dataset was composed of 393 subjects and each expert rated 1156 potential lacunar infarcts as “1 = no”, “2 = probably no”, “3 = probably yes” or “4 = yes”. A consensus (CO) was created by calculating the average and using a cutoff at 2.5. The agreement was measured between individual raters (A‐B, B‐C, A‐C), between automatic rating and individual raters (AU‐A, AU‐B, AU‐C), and between the automatic rating and the consensus rating (AU‐CO) using Kappa. Correlation coefficient for the number of lacunar infarcts per subject was defined. Cross‐validation was used in training the algorithm. Result Out of 1156 candidate regions evaluated, 540 were considered as lacunar infarcts based on consensus. The individual raters agreed with each other in 74% of cases whether the shown lesion was lacunar infarct (kappa = 0.48). Correspondingly, automatic rating agreed with the individual raters in 74% of cases (kappa = 0.47). When automatic rating was compared with consensus, agreement/accuracy was 78% (kappa = 0.55). The correlation coefficient for the number of lacunar infarcts detected automatically from the whole image and visually (consensus) was 0.85 (Figure 1). Conclusion This study suggests that the agreement in detecting lacunar infarcts automatically is comparable to inter‐rater variability between experienced neuroradiologists. [1] Jokinen et al. Stroke 2020 Nov 9; 50(1)
MoreTranslated text
Key words
mri images,lacunar
AI Read Science
Must-Reading Tree
Example
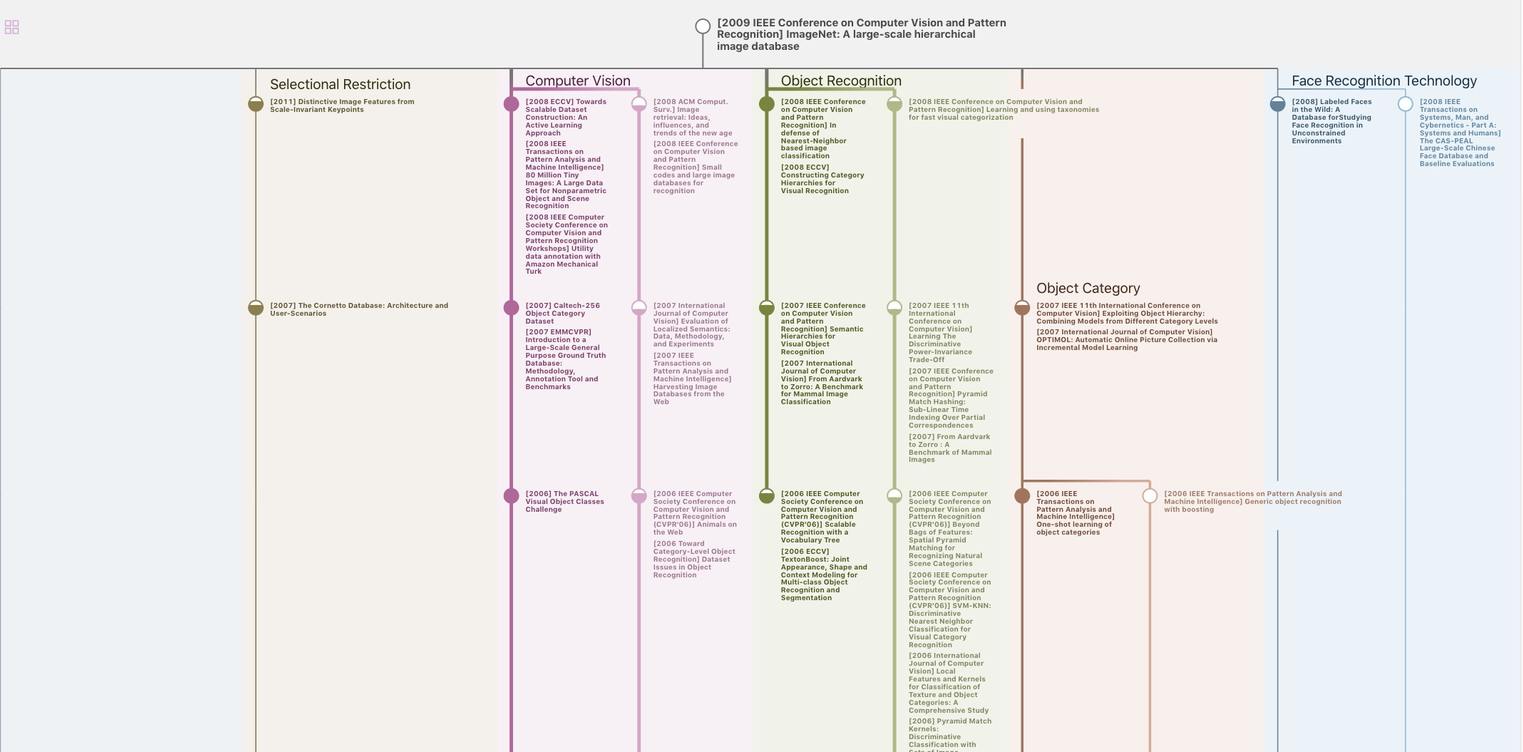
Generate MRT to find the research sequence of this paper
Chat Paper
Summary is being generated by the instructions you defined