Iteratively Refined Behavior Regularization for Offline Reinforcement Learning
arXiv (Cornell University)(2023)
摘要
One of the fundamental challenges for offline reinforcement learning (RL) is ensuring robustness to data distribution. Whether the data originates from a near-optimal policy or not, we anticipate that an algorithm should demonstrate its ability to learn an effective control policy that seamlessly aligns with the inherent distribution of offline data. Unfortunately, behavior regularization, a simple yet effective offline RL algorithm, tends to struggle in this regard. In this paper, we propose a new algorithm that substantially enhances behavior-regularization based on conservative policy iteration. Our key observation is that by iteratively refining the reference policy used for behavior regularization, conservative policy update guarantees gradually improvement, while also implicitly avoiding querying out-of-sample actions to prevent catastrophic learning failures. We prove that in the tabular setting this algorithm is capable of learning the optimal policy covered by the offline dataset, commonly referred to as the in-sample optimal policy. We then explore several implementation details of the algorithm when function approximations are applied. The resulting algorithm is easy to implement, requiring only a few lines of code modification to existing methods. Experimental results on the D4RL benchmark indicate that our method outperforms previous state-of-the-art baselines in most tasks, clearly demonstrate its superiority over behavior regularization.
更多查看译文
关键词
policy,learning,in-sample
AI 理解论文
溯源树
样例
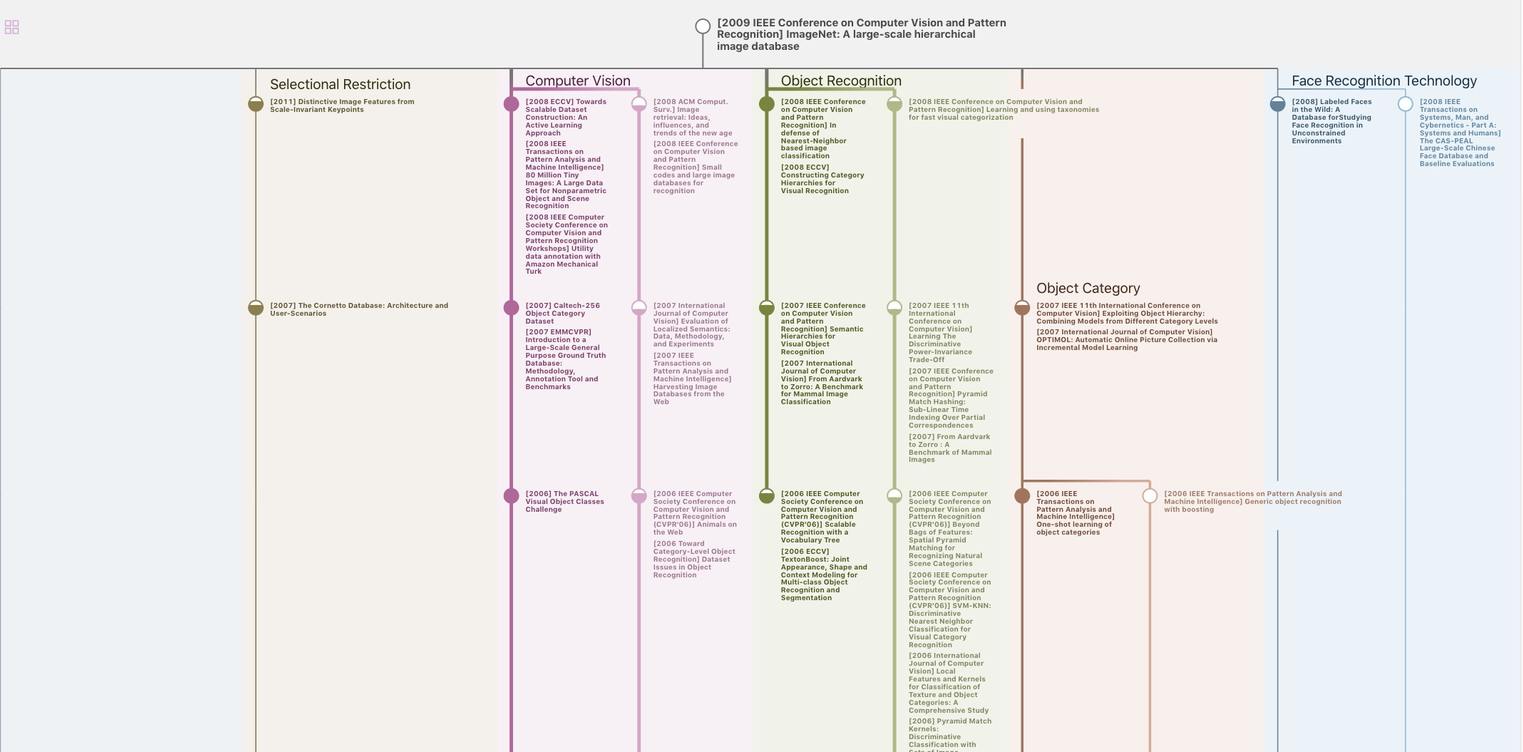
生成溯源树,研究论文发展脉络
Chat Paper
正在生成论文摘要