Patch Extraction and Classifier for Abnormality Classification in Mammography Imaging
openalex(2023)
摘要
Breast cancer is a fatal disease that affects millions of women worldwide. The number of cases of breast cancer has risen over time. Although it is difficult to prevent this disease, the survival rate can be improved with early detection and proper treatment planning of the disease. Thanks to breakthroughs in deep learning, the progress of computer-assisted diagnosis (CAD) of breast cancer has seen a lot of improvement. Deep neural networks have advanced to the point where their diagnostic capabilities are approaching those of a human specialist. Mammograms are crucial radiological images used to detect breast cancer at its early stage. Mammogram image scaling at the input layer is required for training deep convolutional neural networks (DCNNs) directly on high-resolution images. This could result in the loss of crucial information for discovering medical abnormalities. Instead of developing an image classifier, the idea is to create a patch classifier. The technique for extracting abnormal patches from mammography images is proposed in this research. Patches are extracted from a benchmark and publicly available MIAS dataset. These patches are then used to train deep learning classifiers such as VGG-16, ResNet-50, and EfficientNet-B7. With the patches already included in the CBIS-DDSM dataset, we contrast the results of the MIAS patches. EfficientNet-B7 on CBIS-DDSM patches produced good results (92% accuracy) when compared to other classifiers such as VGG-16 and ResNet-50. We also discovered that ResNet-50 is demonstrated to be quite robust on both datasets.
更多查看译文
关键词
mammography imaging,abnormality classification,patch extraction,classifier
AI 理解论文
溯源树
样例
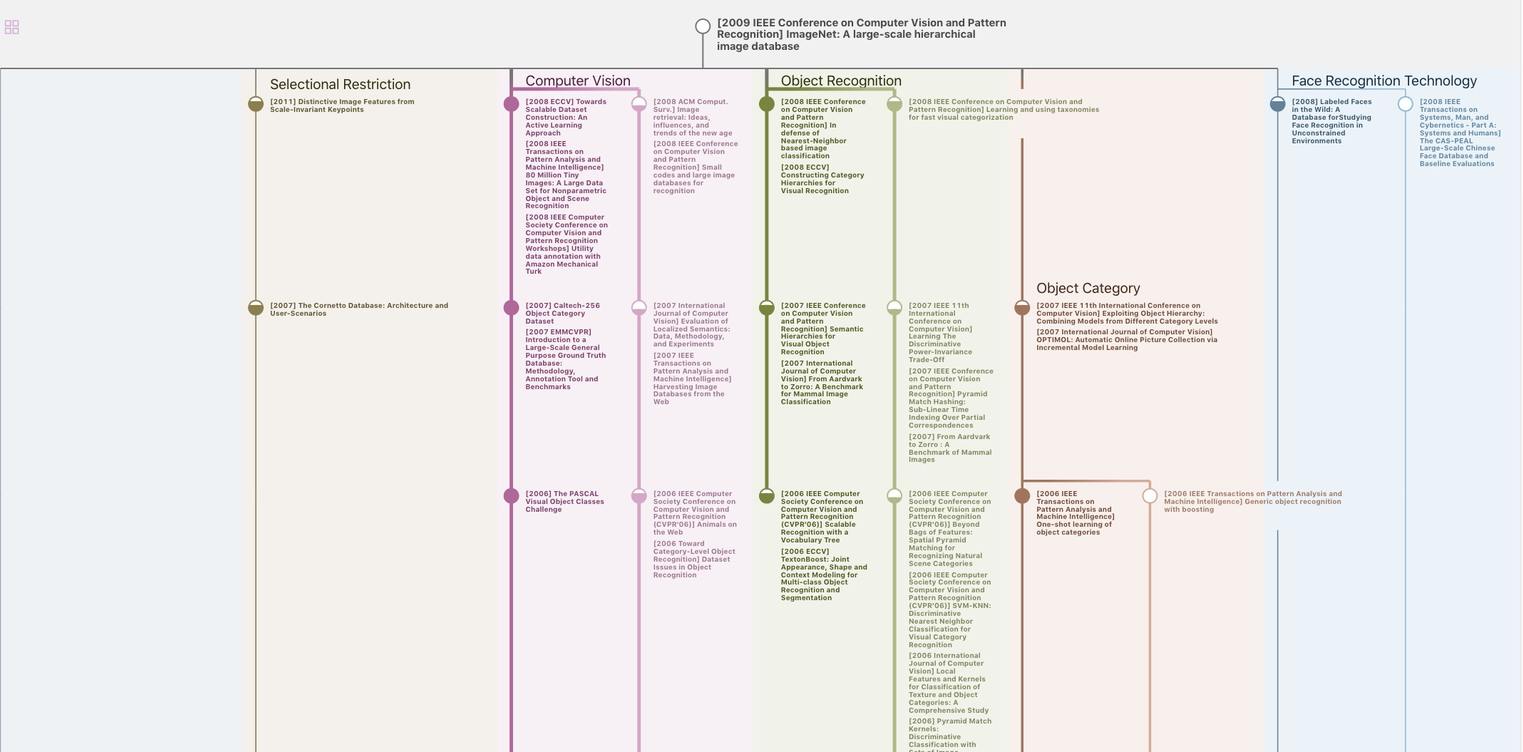
生成溯源树,研究论文发展脉络
Chat Paper
正在生成论文摘要