Utility Of Non-Invasive Tests To Detect any Esophageal Varices In patients with compensated advanced liver disease
Research Square (Research Square)(2023)
摘要
Abstract Background and Aims : Current evidence lay emphasis on detection of clinically significant portal hypertension rather than Varices needing treatment(VNTs) as a decision to start beta-blockers. Esophageal varices (EVs) irrespective of their size are the most reliable indicator of CSPH. While non-invasive tools (NITs) accurately identify those with VNTs, their role to identify any EVs in patients with compensated advanced liver disease(cACLD) is not known. Methods : Patients with cACLD with reliable liver stiffness measurement(LSM), blood parameters and gastroscopy were retrospectively recruited from multinational cohorts. Performance of Baveno-VI (LSM > 20kPa or platelet < 150,000/mm 3 ) and expanded Baveno-VI criteria(LSM > 25kPa or platelet < 110,000/mm 3 ) was assessed to detect VNTs as well as any EVs. This performance was compared with Baveno-VII possible CSPH criteria (LSM ³ 15 kPa and platelet ≤ 150000/mm 3 ) to evaluate its utility to detect any EVs. Result: Patients with cACLD (N=1200) of predominantly viral aetiology (Hepatitis B virus :269, hepatitis C virus: 564, non-alcoholic fatty liver disease: 145, alcoholic liver disease: 130, other: 92) were included. Any EVs and VNTs were present in 514 (42.8%) and 70 (5.8%) patients respectively. Baveno-VI, expanded Baveno-VI and Baveno-VII criteria missed 29/514 (5.6%), 115/514 (22.4%), 19/514 (3.7%) patients with any EVs respectively whereas they misclassified 517/686 (75.4%), 211/686 (30.8%) and 598/686 (87.4%) patients with no EVs as high risk for harbouring EVs. Baveno-VI, expanded Baveno-VI and possible CSPH criteria missed 3/70 (4.3%) and 15/70 (21.4%) and 0/70 (0%) VNTs respectively. Conclusion: Both original Baveno-VI and Baveno-VII criteria are highly sensitive to detect varices in cACLD albeit with a high misclassification rates.
更多查看译文
关键词
esophageal varices,liver disease,non-invasive
AI 理解论文
溯源树
样例
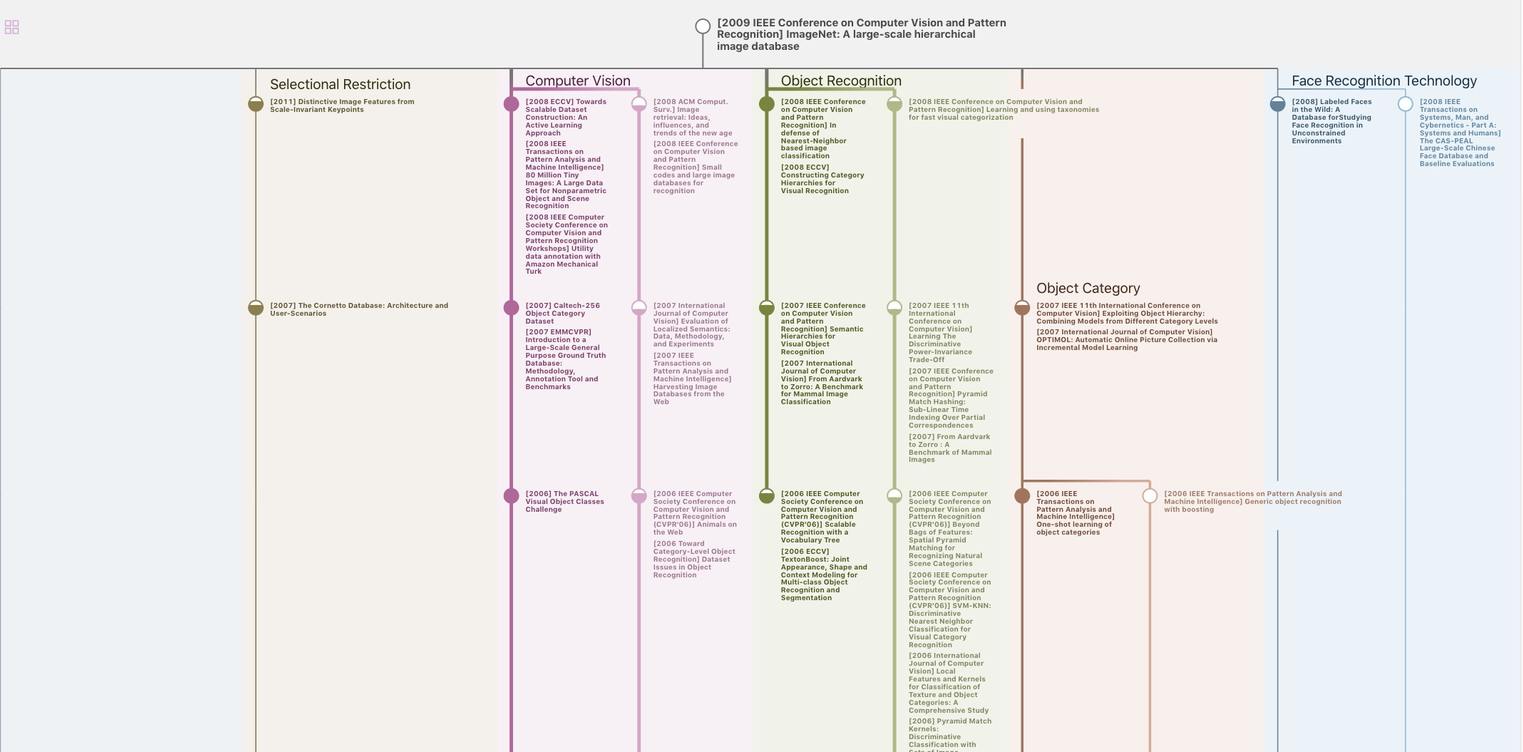
生成溯源树,研究论文发展脉络
Chat Paper
正在生成论文摘要