LECA: A learned approach for efficient cover-agnostic watermarking
IS&T International Symposium on Electronic Imaging Science and Technology(2023)
摘要
In this work, we present an efficient multi-bit deep image watermarking method that is cover-agnostic yet also robust to geometric distortions such as translation and scaling as well as other distortions such as JPEG compression and noise. Our design consists of a light-weight watermark encoder jointly trained with a deep neural network based decoder. Such a design allows us to retain the efficiency of the encoder while fully utilizing the power of a deep neural network. Moreover, the watermark encoder is independent of the image content, allowing users to pre-generate the watermarks for further efficiency. To offer robustness towards geometric transformations, we introduced a learned model for predicting the scale and offset of the watermarked images. Moreover, our watermark encoder is independent of the image content, making the generated watermarks universally applicable to different cover images. Experiments show that our method outperforms comparably efficient watermarking methods by a large margin.
更多查看译文
关键词
cover-agnostic
AI 理解论文
溯源树
样例
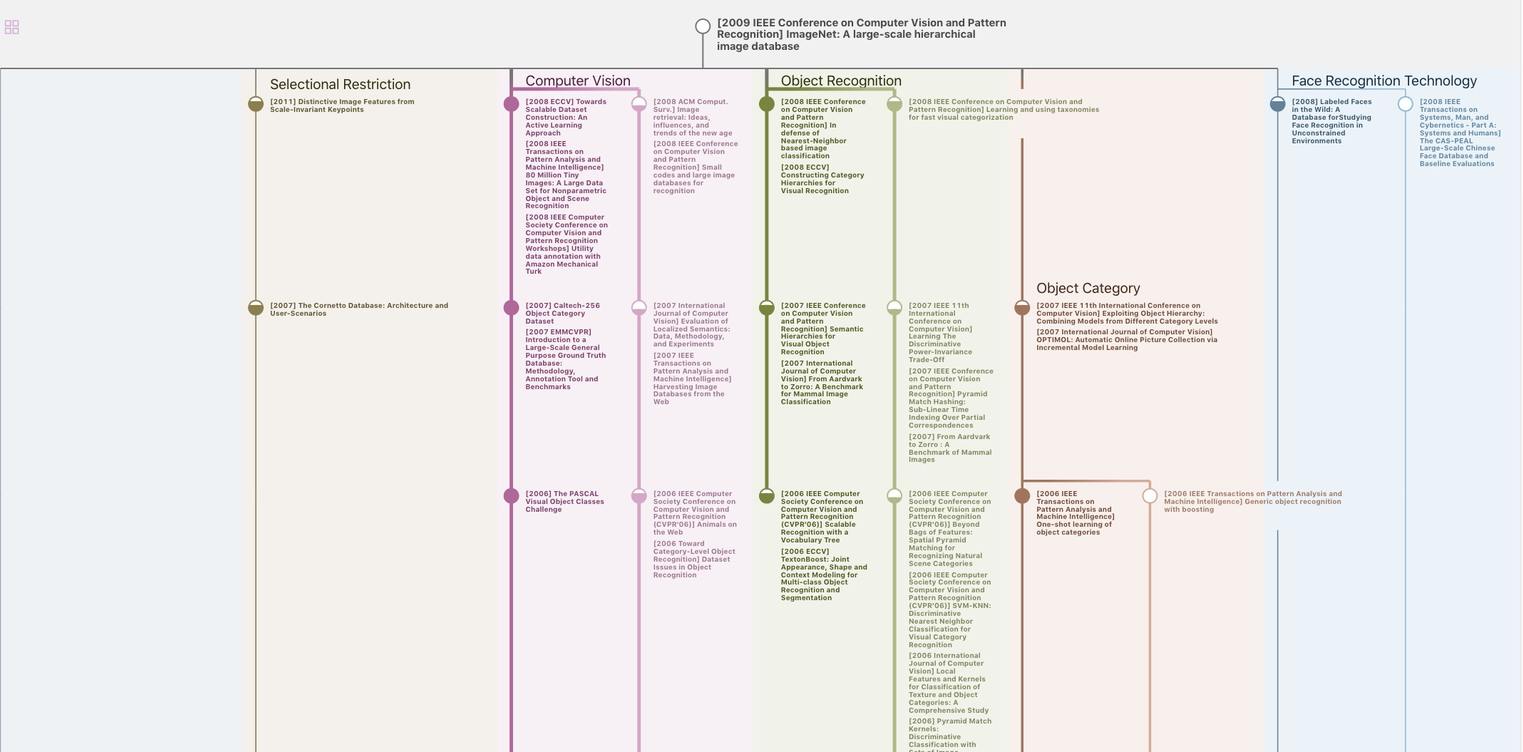
生成溯源树,研究论文发展脉络
Chat Paper
正在生成论文摘要