UMIM: Utility-Maximization Incentive Mechanism for Mobile Crowd Sensing
IEEE Transactions on Mobile Computing(2023)
摘要
Mobile Crowd Sensing (MCS) represents a novel paradigm which utilizes intelligent devices carried by mobile users to collect and transmit data. Appropriate incentives are essential to recruit enough participants for sensing tasks. Existing works have designed some incentive mechanisms for MCS, which are not suitable for scenarios when the participants increase significantly as a result of the booming cost. To solve the above cost problem, a Utility-Maximization Incentive Mechanism (UMIM) is proposed in this paper by leveraging the influence propagation on the social network. Participants in the same social network can benefit from the data shared by others, which shows the utility of sensing data and can be regarded as a non-monetary incentive and make the participants stay positive under relative low payoff. Therefore, by improving the utility of sensing data, the incentive cost can be effectively reduced. To maximize the data utility, we further design a tree-based structure to improve the priority experience replay mechanism of Proximal Policy Optimization (PPO) in UMIM. This improvement makes the high priority experience to be sampled more quickly and efficiently, as a result, the network can learn more effectively. Numerical results show that UMIM can further improve the data utility and have better convergence.
更多查看译文
关键词
Mobile Crowd Sensing (MCS),Utility-Maximization Incentive Mechanism (UMIM),Social Utility,Deep Reinforcement Learning (DRL),Proximal Policy Optimization (PPO),Priority Experience Replay
AI 理解论文
溯源树
样例
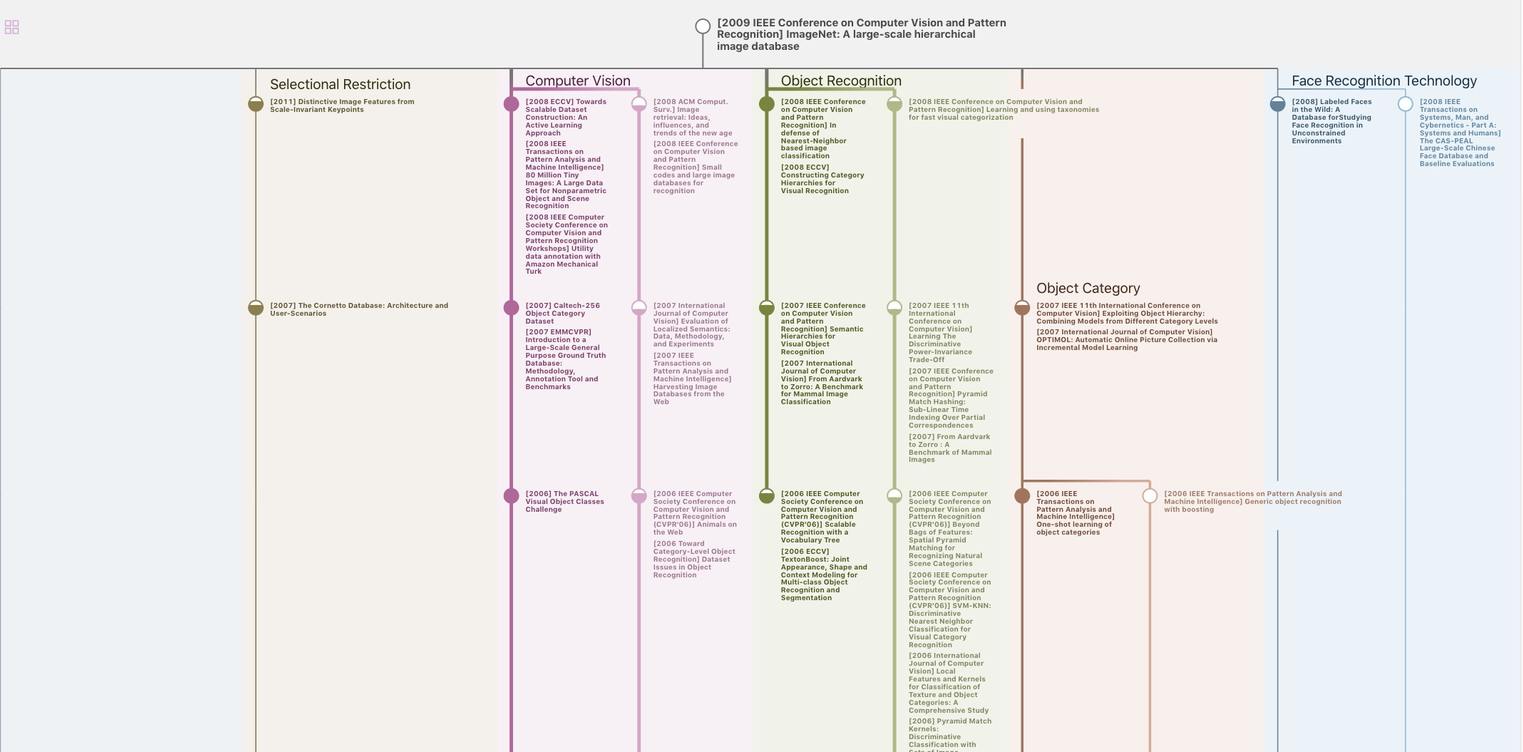
生成溯源树,研究论文发展脉络
Chat Paper
正在生成论文摘要