Robust 3D Semantic Segmentation Based on Multi-Phase Multi-Modal Fusion for Intelligent Vehicles.
IEEE Trans. Intell. Veh.(2024)
摘要
3D semantic segmentation is a key technology for intelligent vehicles. Recently, great efforts have been made to achieve accurate and robust 3D semantic segmentation results through LiDAR-camera fusion, due to the complementary information of images like color and texture. However, current LiDAR-camera fusion methods still lag behind LiDAR-only methods in complex and varied scenarios containing sparse and similar geometric features. Three main factors limit their performance: sparse mappings between two modalities, the contribution of different modality features, and the gap between results of two modalities. Addressing that, we present a multi-phase LiDAR-camera fusion method, which comprehensively fuses information of two modalities in three phases: first, a context sampler block is proposed to fuse low-level image contextual features into LiDAR points; second, a cross dimensional fusion block is designed to implement the interaction of shared features for hierarchical supervision while retaining private features for jointly refining their predictions; third, a modality perceptual conformance loss is induced to make them learn from each other in an explicit manner by minimizing the gap between two modality predictions. Quantitative and qualitative evaluations with state-of-the-art (SOTA) methods are performed on urban dataset SemanticKITTI, off-road dataset RELLIS-3D and our unstructured test set. The experimental results validate the competitiveness of our method in improving accuracy and scene-adaptivity.
更多查看译文
关键词
Intelligent vehicles,3D semantic segmentation,multi-modal fusion,multi-phase
AI 理解论文
溯源树
样例
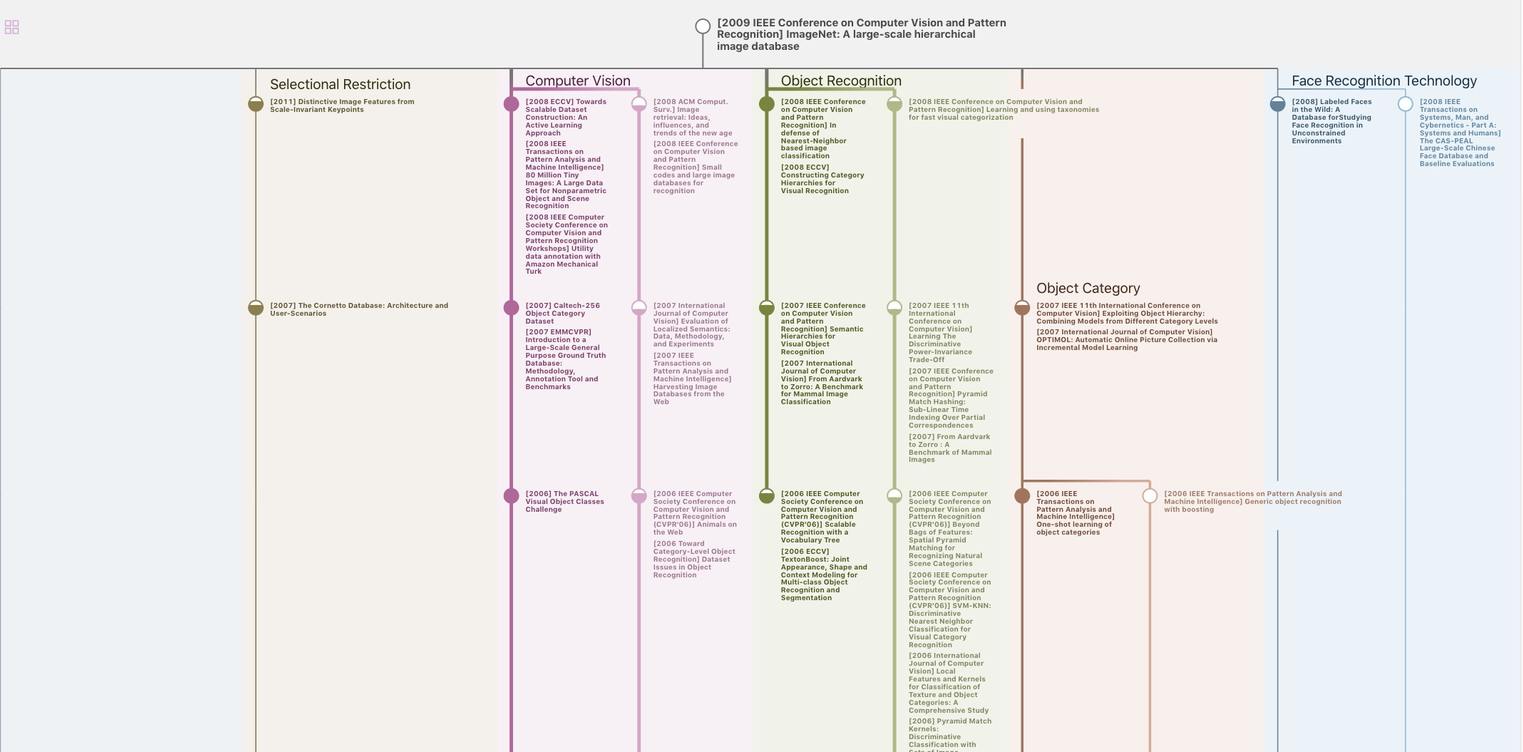
生成溯源树,研究论文发展脉络
Chat Paper
正在生成论文摘要