Efficient Decentralized Multi-agent Learning in Asymmetric Bipartite Queueing Systems
Operations Research(2023)
摘要
New Algorithm Enables Efficient Decentralized Learning in Bipartite Queueing Systems Bipartite queueing systems, where agents with individual job queues request service from a pool of heterogeneous servers, are standard models for service applications like data networks and call centers. Traditionally, a central controller schedules agent requests with full knowledge of system parameters. However, emerging applications require decentralized operation without this central coordination or complete system information. This presents challenges as agents lack the global knowledge needed to efficiently route jobs. Recent research into efficient decentralized learning algorithms for such systems faces limitations in nonoptimal throughput, demanding computations, or degrading efficiency with exponential queue growth. In contrast, this paper introduces an algorithm that enables queues to efficiently learn decentralized scheduling policies while ensuring throughput optimality. The approach is computationally lightweight, achieving queue length bounds that scale polynomially rather than exponentially in system size. Experiments demonstrate faster convergence and robustness of our algorithm compared with prior decentralized algorithms.
更多查看译文
关键词
decentralized,multi-agent
AI 理解论文
溯源树
样例
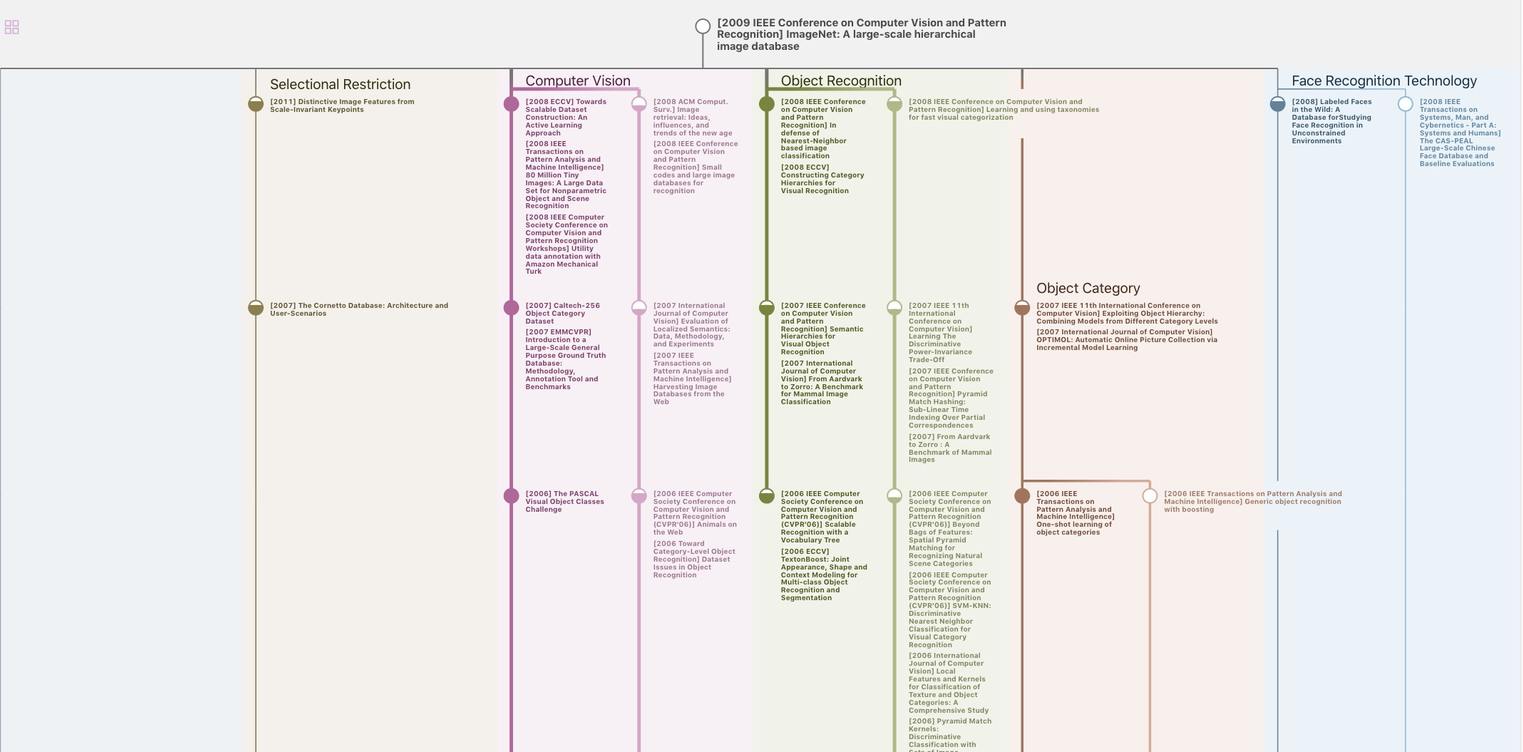
生成溯源树,研究论文发展脉络
Chat Paper
正在生成论文摘要