Explainable Document Classification via Pattern Structures
Lecture notes in networks and systems(2023)
摘要
Inherently explainable Machine Learning (ML) models are able to provide explanations for their predictions by virtue of their construction. The explanations of a ML model are more comprehensible if they are expressed in terms of its input features. Our paper proposes an inherently explainable pipeline for document classification using pattern structures and Abstract Meaning Representation (AMR) graphs. The pipeline generates two kinds of explanations: intermediate and final ones, that justify its classifications. Intermediate explanations are represented as significant subgraphs found in the document graphs of test documents. Final explanations are the sentences of the test documents, that correspond to the significant subgraphs.
更多查看译文
关键词
classification,structures
AI 理解论文
溯源树
样例
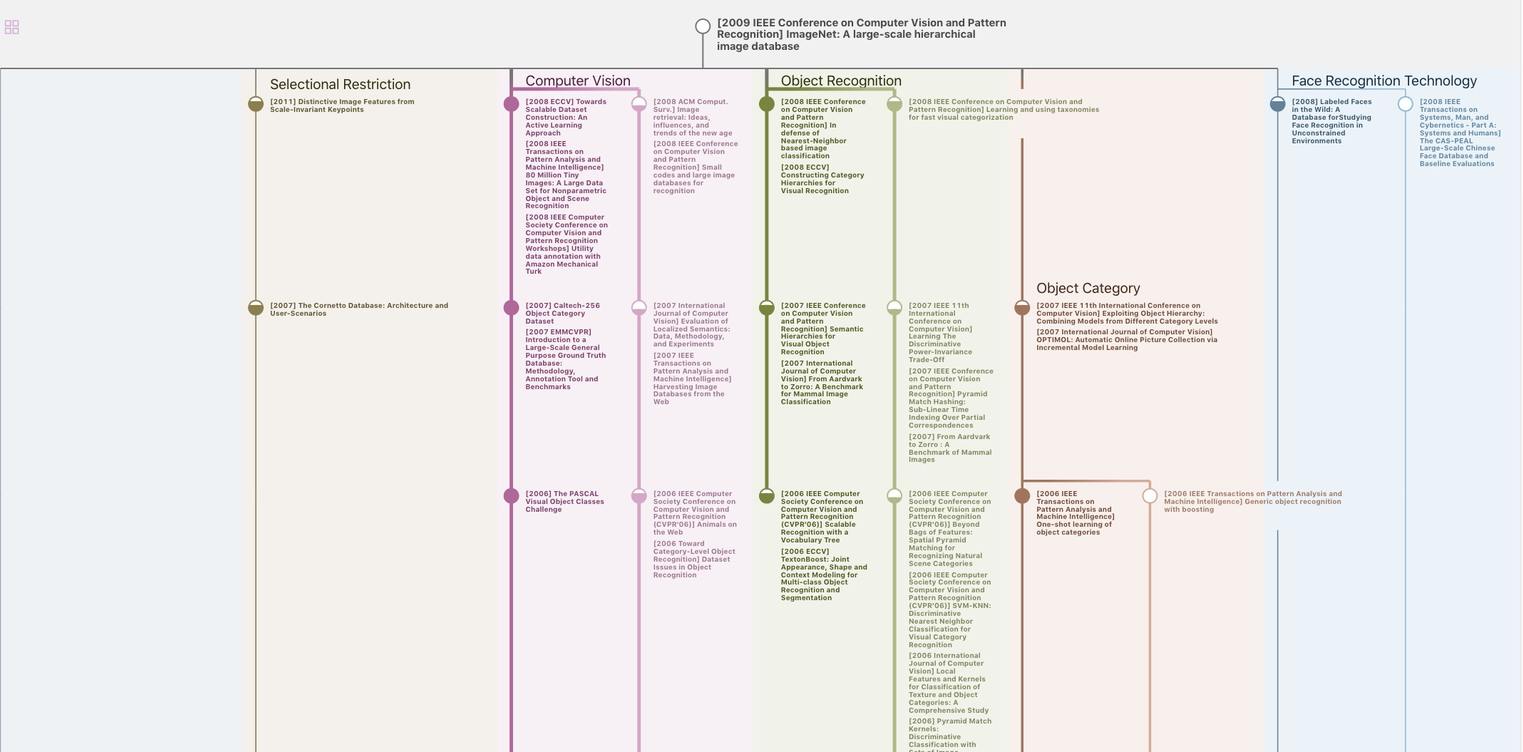
生成溯源树,研究论文发展脉络
Chat Paper
正在生成论文摘要